Semantic-Aware Registration with Weakly-Supervised Learning
CANCER PREVENTION THROUGH EARLY DETECTION, CAPTION 2022(2022)
摘要
Medical image registration is a fundamental task for many clinical applications. Most deep learning-based image registrations methods have achieved brilliant performance owing to the incorporation of mask information. However, existing mask-guided registration methods only focus on volumetric registration inside the paired masks, ignoring the inherent attributes of the anatomical structure in other dimensions (e.g., smoothness of organ surface, connectivity of tubular structures, etc.). To address this problem, we proposed a novel semantic-aware registration network suitable for multi-organs registration with different anatomical structures. By analyzing the structural characteristics of various organs or tissues, semantic constraints are directly imposed on the deformation field from two geometrically meaningful dimensions (surface, contour) to preserve the topology of organs or tissues. To ensure the versatility of our proposed network, only a randomly selected segmentation mask is used for supervision at each iteration during training. Such a training strategy can satisfy the accurate registration of an individual organ at the inference stage. Experiments on a pelvic CT dataset with 112 subjects show that our method can achieve higher registration accuracy and preserve the anatomical structure more effectively than state-of-the-art methods.
更多查看译文
关键词
learning,semantic-aware,weakly-supervised
AI 理解论文
溯源树
样例
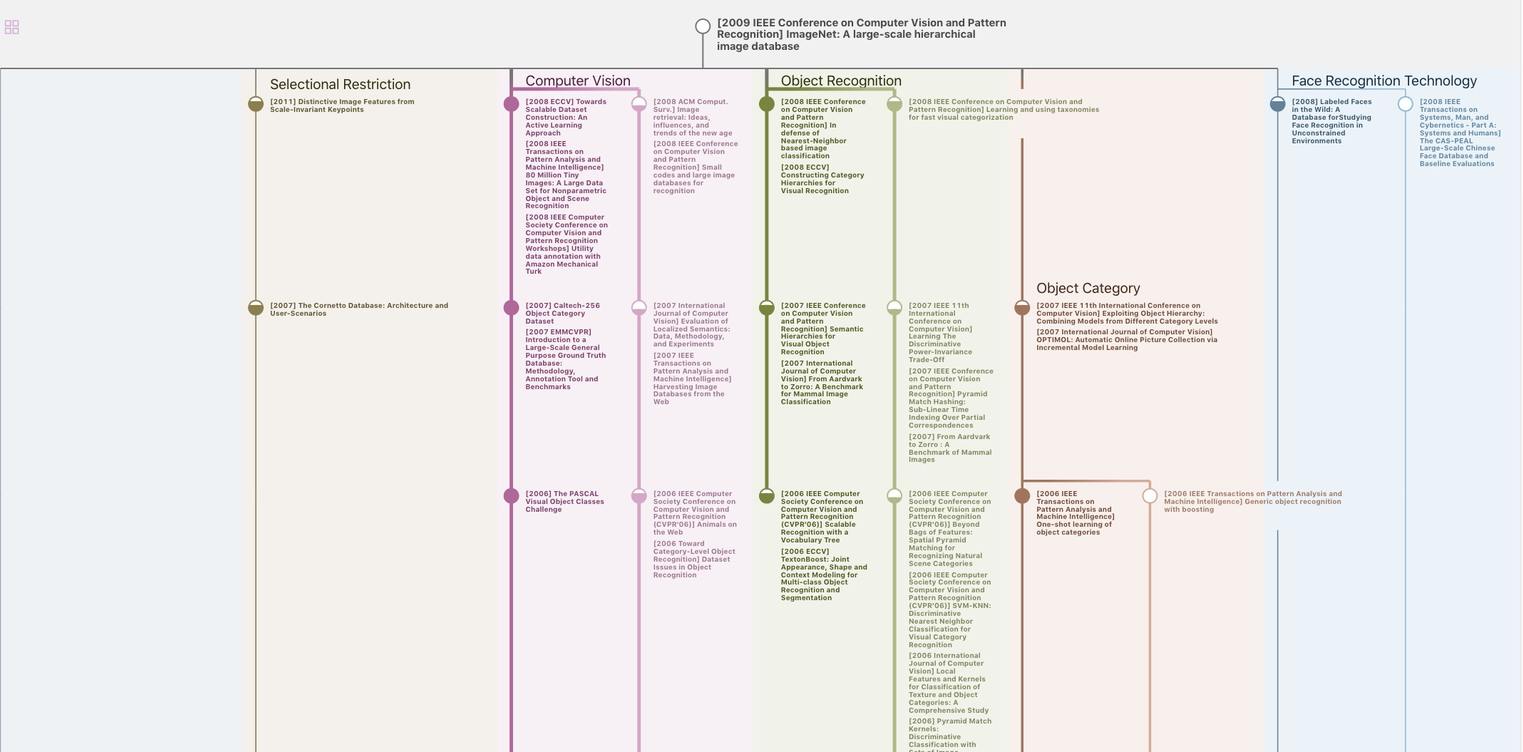
生成溯源树,研究论文发展脉络
Chat Paper
正在生成论文摘要