A prior knowledge-embedded reinforcement learning method for real-time active power corrective control in complex power systems
FRONTIERS IN ENERGY RESEARCH(2022)
摘要
With the increasing uncertainty and complexity of modern power grids, the real-time active power corrective control problem becomes intractable, bringing significant challenges to the stable operation of future power systems. To promote effective and efficient active power corrective control, a prior knowledge-embedded reinforcement learning method is proposed in this paper, to improve the performance of the deep reinforcement learning agent while maintaining the real-time control manner. The system-level feature is first established based on prior knowledge and cooperating with the equipment-level features, to provide a thorough description of the power network states. A global-local network structure is then constructed to integrate the two-level information accordingly by introducing the graph pooling method. Based on the multi-level representation of power system states, the Deep Q-learning from Demonstrations method is adopted to guide the deep reinforcement learning agent to learn from the expert policy along with the interactive improving process. Considering the infrequent corrective control actions in practice, the double-prioritized training mechanism combined with the lambda-return is further developed to help the agent lay emphasis on learning from critical control experience. Simulation results demonstrate that the proposed method prevails over the conventional deep reinforcement learning methods in training efficiency and control effects, and has the potential to solve the complex active power corrective control problem in the future.
更多查看译文
关键词
active power corrective control, deep Q-learning from demonstrations, graph pooling, power system, prior knowledge
AI 理解论文
溯源树
样例
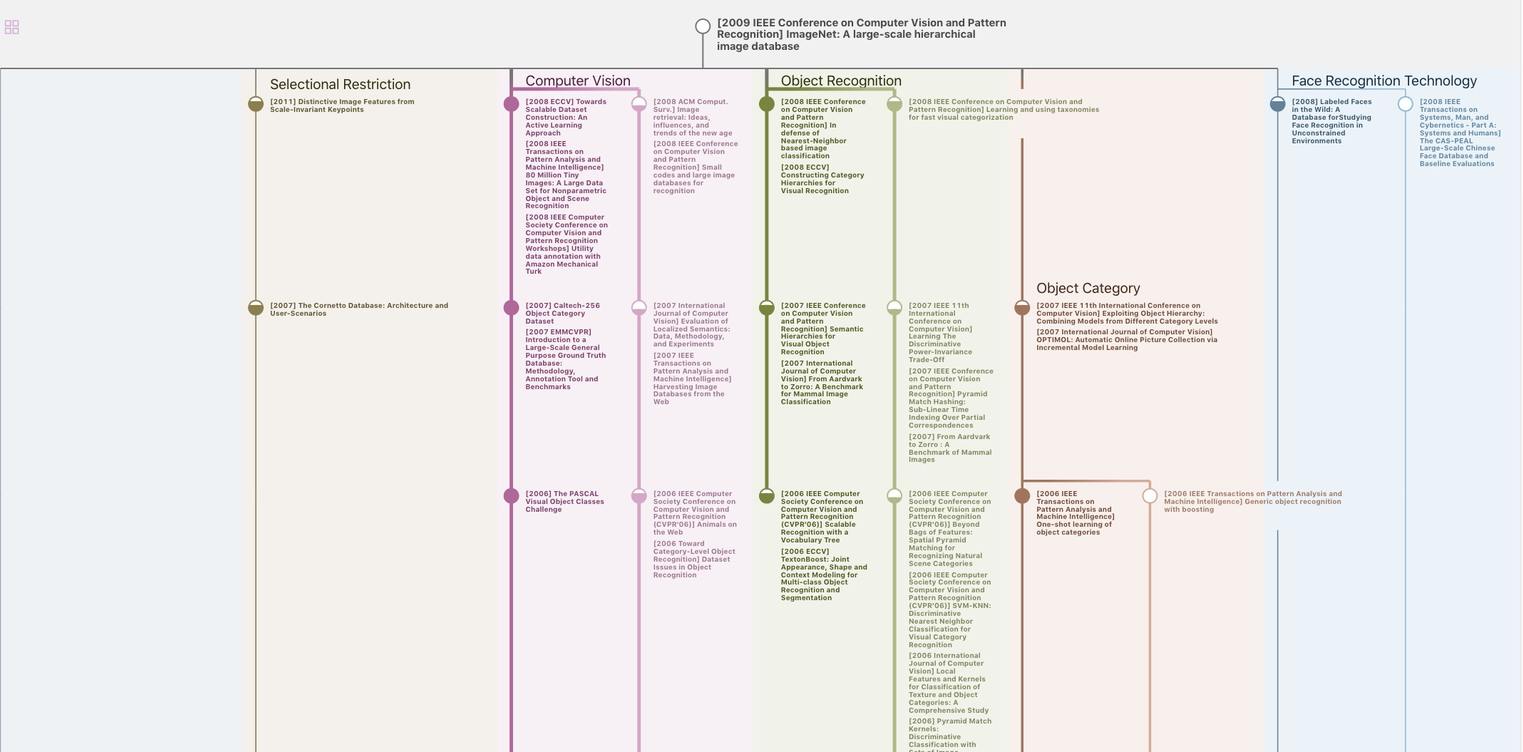
生成溯源树,研究论文发展脉络
Chat Paper
正在生成论文摘要