PDF Malware Detection Based on Optimizable Decision Trees
ELECTRONICS(2022)
摘要
Portable document format (PDF) files are one of the most universally used file types. This has incentivized hackers to develop methods to use these normally innocent PDF files to create security threats via infection vector PDF files. This is usually realized by hiding embedded malicious code in the victims' PDF documents to infect their machines. This, of course, results in PDF malware and requires techniques to identify benign files from malicious files. Research studies indicated that machine learning methods provide efficient detection techniques against such malware. In this paper, we present a new detection system that can analyze PDF documents in order to identify benign PDF files from malware PDF files. The proposed system makes use of the AdaBoost decision tree with optimal hyperparameters, which is trained and evaluated on a modern inclusive dataset, viz. Evasive-PDFMal2022. The investigational assessment demonstrates a lightweight and accurate PDF detection system, achieving a 98.84% prediction accuracy with a short prediction interval of 2.174 mu Sec. To this end, the proposed model outperforms other state-of-the-art models in the same study area. Hence, the proposed system can be effectively utilized to uncover PDF malware at a high detection performance and low detection overhead.
更多查看译文
关键词
portable document format (PDF), machine learning, detection, optimizable decision tree, AdaBoost, PDF malware, evasion attacks, cybersecurity
AI 理解论文
溯源树
样例
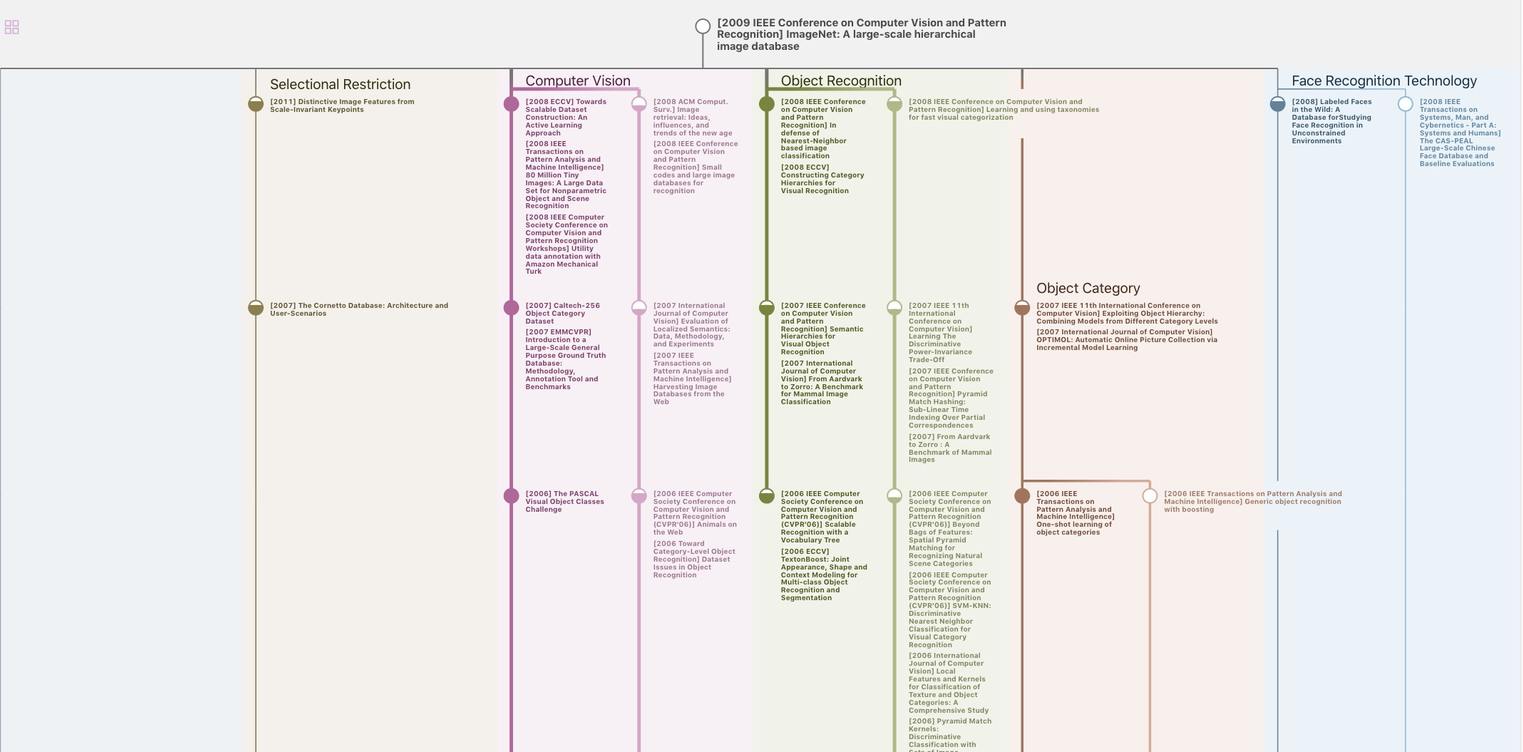
生成溯源树,研究论文发展脉络
Chat Paper
正在生成论文摘要