Iterative Prior Resampling and Rejection Sampling to Improve 1-D Geophysical Imaging Based on Bayesian Evidential Learning (BEL1D)
GEOPHYSICAL JOURNAL INTERNATIONAL(2023)
摘要
SUMMARY The non-uniqueness of the solution of inverse geophysical problem has been recognized for a long-time. Although stochastic inversion methods have been developed, deterministic inversion using subsequent regularization is still more widely applied. This is likely due to their efficiency and robustness, compared to the computationally expensive and sometimes difficult to tune to convergence stochastic methods. Recently, Bayesian evidential learning 1-D imaging has been presented to the community as a viable tool for the efficient stochastic 1-D imaging of the subsurface based on geophysical data. The method has been proven to be as fast, or sometimes even faster, than deterministic solution. However, the method has a significant drawback when dealing with large prior uncertainty as often encountered in geophysical surveys: it tends to overestimate the uncertainty range. In this paper, we provide an efficient way to overcome this limitation through iterative prior resampling (IPR) followed by rejection sampling. IPR adds the posterior distribution calculated at a former iteration to the prior distribution in a subsequent iteration. This allows to sharpen the learning phase of the algorithm and improve the estimation of the final posterior distribution while rejection sampling eliminates models not fitting the data. In this contribution, we demonstrate that this new approach allows BEL1D to converge towards the true posterior distribution. We also analyse the convergence behaviour of the algorithm and derive guidelines for its application. We apply the approach for the interpretation of surface waves dispersion curves but the approach can be generalized to other geophysical methods.
更多查看译文
关键词
Inverse theory,Machine learning,Probability distributions,Surface waves and free oscillations
AI 理解论文
溯源树
样例
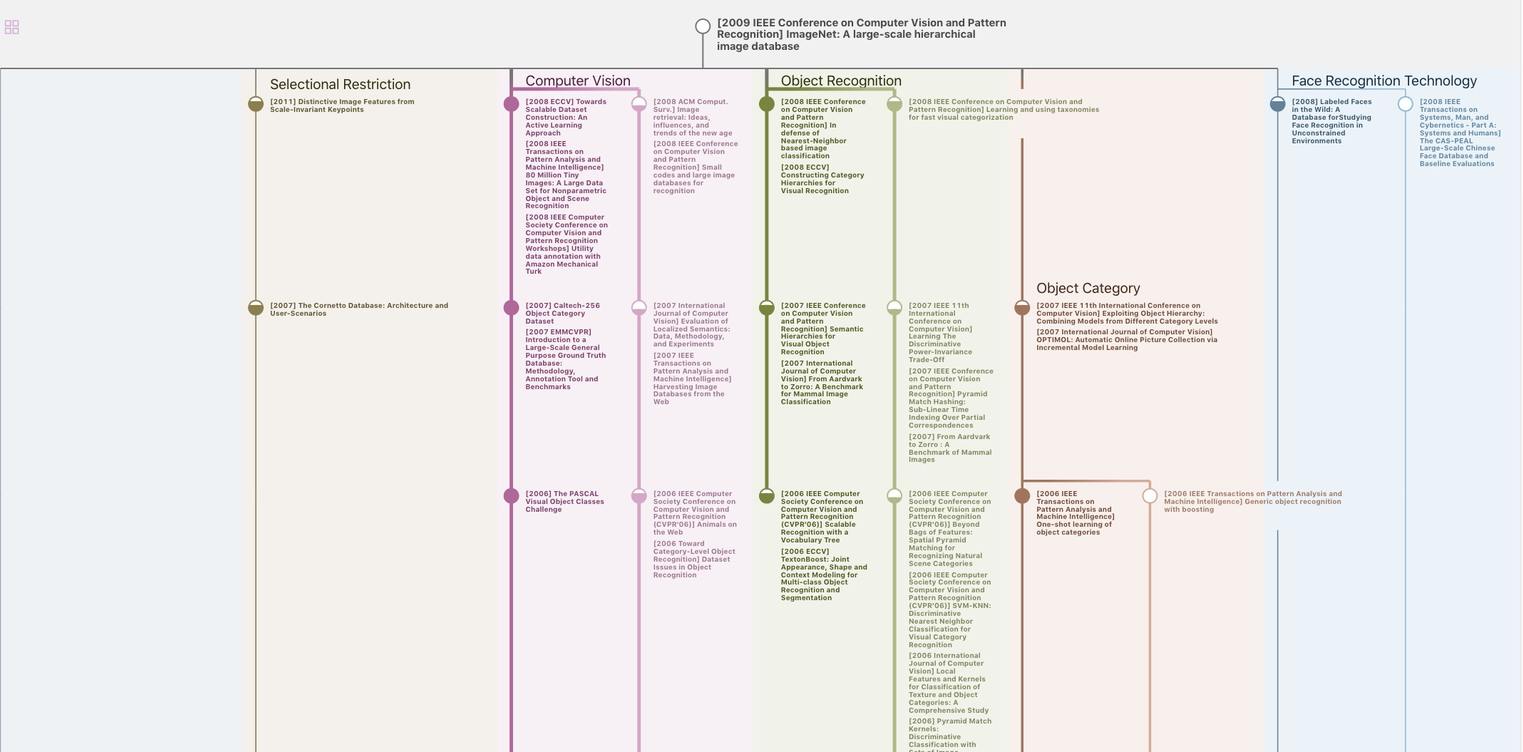
生成溯源树,研究论文发展脉络
Chat Paper
正在生成论文摘要