Generating Daily Land Surface Temperature Downscaling Data Based on Sentinel-3 Images
REMOTE SENSING(2022)
摘要
The land surface temperature (LST) images obtained by thermal infrared remote sensing sensors are of great significance for numerous fields of research. However, the low spatial resolution is a drawback of LST images. Downscaling is an effective way to solve this problem. The traditional downscaling methods, however, have various drawbacks, including their low temporal and spectral resolutions, difficult processes, numerous errors, and single downscaling factor. They also rely on two or more separate satellite platforms. These drawbacks can be partially compensated for by the Sentinel-3 satellite's ability to acquire LST and multispectral images simultaneously. This paper proposes a downscaling model based on Sentinel-3 satellite and ASTER GDEM images-D-DisTrad-and compares the effects of the D-DisTrad model with DisTrad model and TsHARP model over four sites and four seasons. The mean bias (MB) range of the D-DisTrad model is -0.001-0.017 K, the mean absolute error (MAE) range is 0.103-0.891 K, and the root mean square error (RMSE) range is 0.220-1.235 K. The Pearson correlation coefficient (PCC) and R-2 ranges are 0.938-0.994 and 0.889-0.989, respectively. The D-DisTrad model has the smallest error, the highest correlation, and the best visual effect, and can eliminate some "mosaic" effects in the original image. This paper shows that the D-DisTrad model can improve the spatial resolution and visual effects of LST images while maintaining high temporal resolution, and discusses the influence of the terrain and land cover on LST data.
更多查看译文
关键词
thermal remote sensing,land surface temperature,downscaling,Sentinel-3,ASTER GDEM,D-DisTrad,DisTrad,TsHARP
AI 理解论文
溯源树
样例
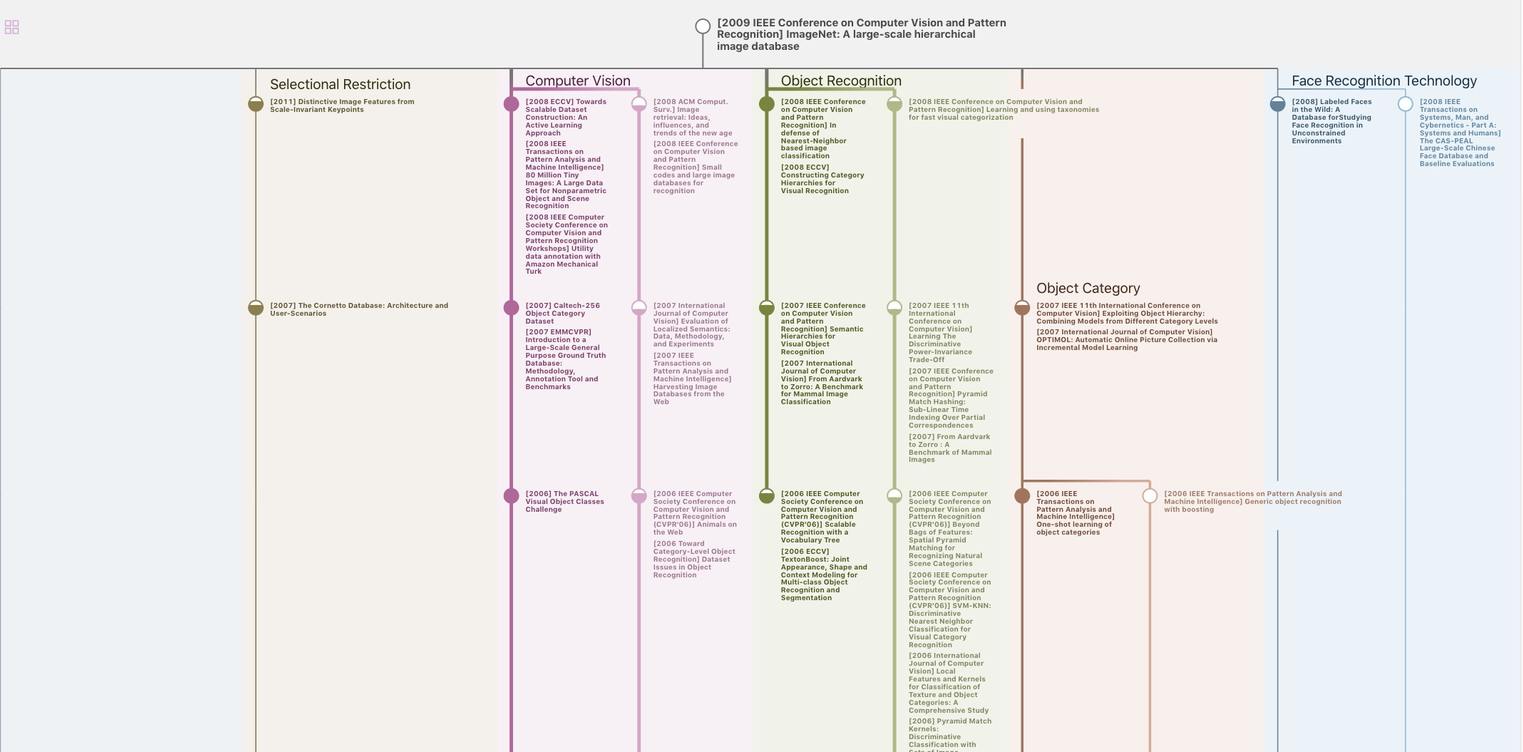
生成溯源树,研究论文发展脉络
Chat Paper
正在生成论文摘要