Crowd counting via Localization Guided Transformer.
Comput. Electr. Eng.(2022)
摘要
The rapidly growing demands on real-world crowd security and commercial applications have drawn widespread attentions to crowd counting, a computer vision task that aims to count all persons that appear in a given image. Recent state-of-the-art crowd counting methods commonly follow the density map regression paradigm, where a density map is estimated from the given image and summed up as the total count. Despite achieving impressive progress, these methods are still significantly challenged by complicated scenarios with severe scale variations of persons and cluttered backgrounds. Considering that localization-based counting methods, though less accurate, are able to learn more discriminative representation of persons through locating their positions, we propose a novel Localization Guided Transformer (LGT) framework in this work. The LGT aims to use the knowledge learned from a leading localization-based method to more accurately guide the estimation on density maps for crowd counting. Specifically, our framework first exploits a point-based model with two output heads, i.e., regression head and classification head, to simultaneously predict the head point proposals and point confidence respectively. Then, an intermediate multi-scale feature map is extracted from the shared backbone network and actively fused with the point location information. Afterwards, the fused features are fed into a Transformer module to explore patch-wise interactions via the self-attention mechanism, yielding a more discriminative representation for high-quality density map estimation. Extensive experiments and comparisons with state-of-the-art methods show the effectiveness of our proposed framework.
更多查看译文
关键词
Crowd counting,Density map,Localization guidance,Transformer,Deep learning
AI 理解论文
溯源树
样例
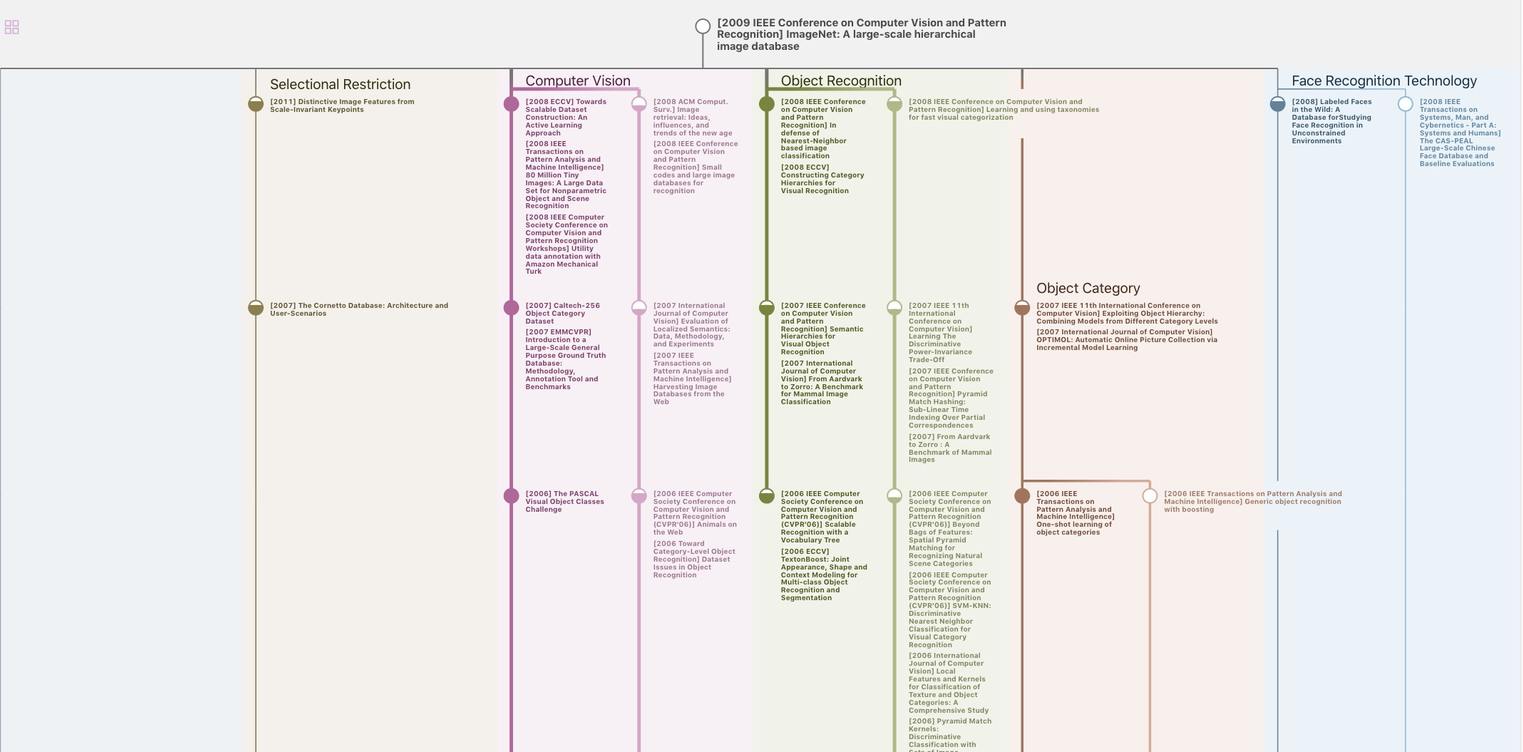
生成溯源树,研究论文发展脉络
Chat Paper
正在生成论文摘要