In-depth investigation of the bioethanol and biogas production from organic and mineral acid pretreated sugarcane bagasse: Comparative and optimization studies
Biocatalysis and Agricultural Biotechnology(2022)
Abstract
Organic acid pretreatment has generated much interest as one of the high potential methods for promoting enzymatic saccharification of lignocellulosic materials. Organic acids are preferred during pretreatment because they are less hazardous than conventional ones and produce less inhibitory fermentation by-products. In the present study, three organic acids (oxalic acid, citric acid, and acetic acid) and inorganic acid (hydrochloric acid) were studied to produce bioethanol and biogas from sugarcane bagasse. An optimization design (Box–Behnken Design (BBD)) among different optimization designs of response surface methodology (RSM) was considered to determine the pretreatment optimum conditions. On this basis, citric acid (CA)pretreatment resulted in maximum glucose yield (7.93 mg/mL) during saccharification. FTIR analysis of the pretreated samples showed that the structure of lignocellulose was changed in higher proportions for the sample pretreated with an organic acid. An increase in cellulose portion and maximum removal of hemicellulose with lower furan generations was observed in organic acid samples. In this study, the maximum solubilization of hemicellulose in hydrochloric acid was also observed due to the abundance of free H+ ions to catalyze the reaction. The ethanol and biogas yield increased by 1.96 and 1.85-fold for citric acid and oxalic acid pretreated sugarcane bagasse, respectively than untreated samples. The present study concluded that oxalic acid and citric acid pretreatment showed the potential to enhance the yield of bioethanol and biogas, respectively. An organic acid in the pretreatment of lignocellulosic biomass can replace traditional methods of using inorganic acids in biorefineries.
MoreTranslated text
Key words
Organic acid,Mineral acid,Fermentation,Anaerobic digestion,Optimization,Saccharification
AI Read Science
Must-Reading Tree
Example
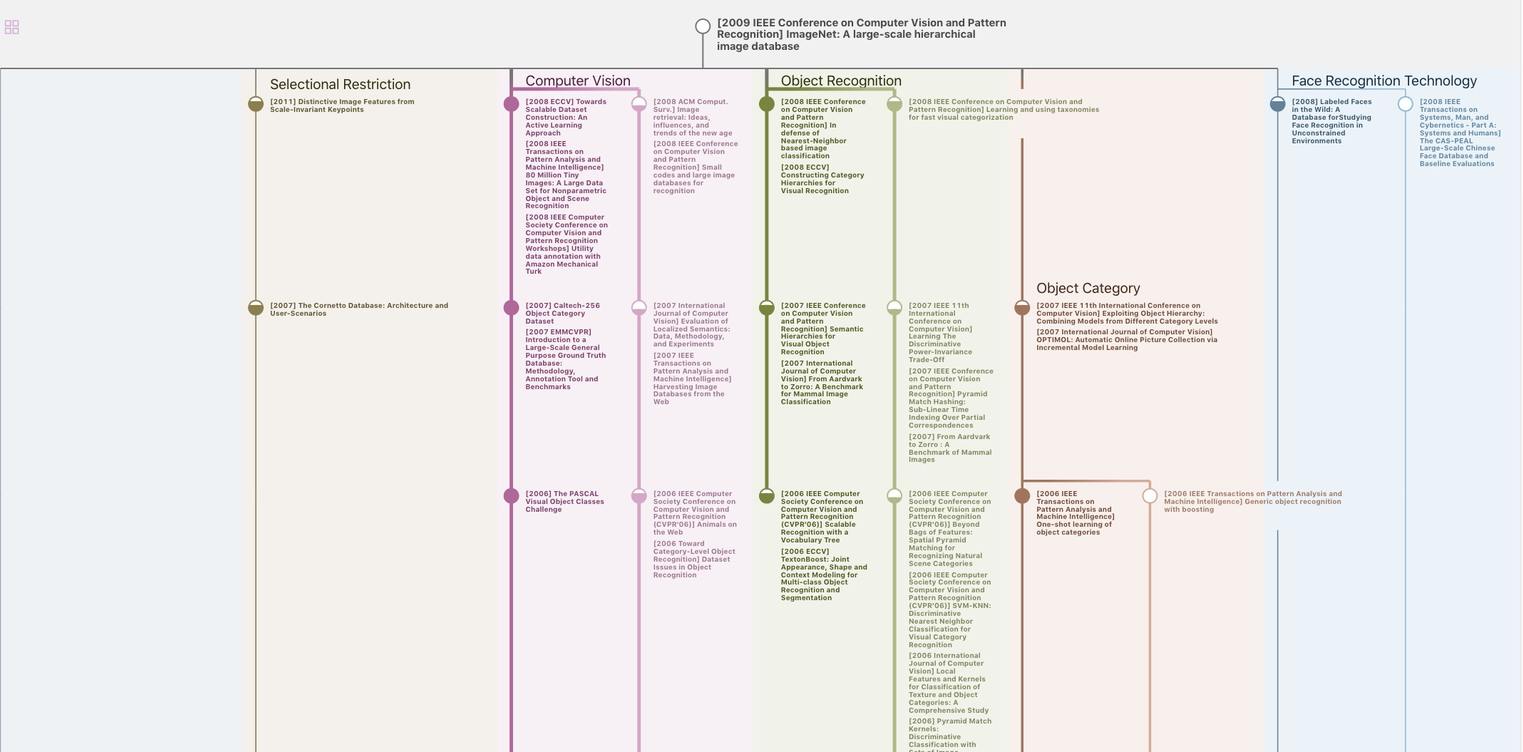
Generate MRT to find the research sequence of this paper
Chat Paper
Summary is being generated by the instructions you defined