The Biases of Pre-Trained Language Models: an Empirical Study on Prompt-Based Sentiment Analysis and Emotion Detection.
IEEE transactions on affective computing(2023)
摘要
Thanks to the breakthrough of large-scale pre-trained language model (PLM) technology, prompt-based classification tasks, e.g., sentiment analysis and emotion detection, have raised increasing attention. Such tasks are formalized as masked language prediction tasks which are in line with the pre-training objects of most language models. Thus, one can use a PLM to infer the masked words in a downstream task, then obtaining label predictions with manually defined label-word mapping templates. Prompt-based affective computing takes the advantages of both neural network modeling and explainable symbolic representations. However, there still remain many unclear issues related to the mechanisms of PLMs and prompt-based classification. We conduct a systematic empirical study on prompt-based sentiment analysis and emotion detection to study the biases of PLMs towards affective computing. We find that PLMs are biased in sentiment analysis and emotion detection tasks with respect to the number of label classes, emotional label-word selections, prompt templates and positions, and the word forms of emotion lexicons.
更多查看译文
关键词
Task analysis,Emotion recognition,Sentiment analysis,Computational modeling,Affective computing,Taxonomy,Analytical models,Emotion detection,pre-trained language model,prompt,sentiment analysis
AI 理解论文
溯源树
样例
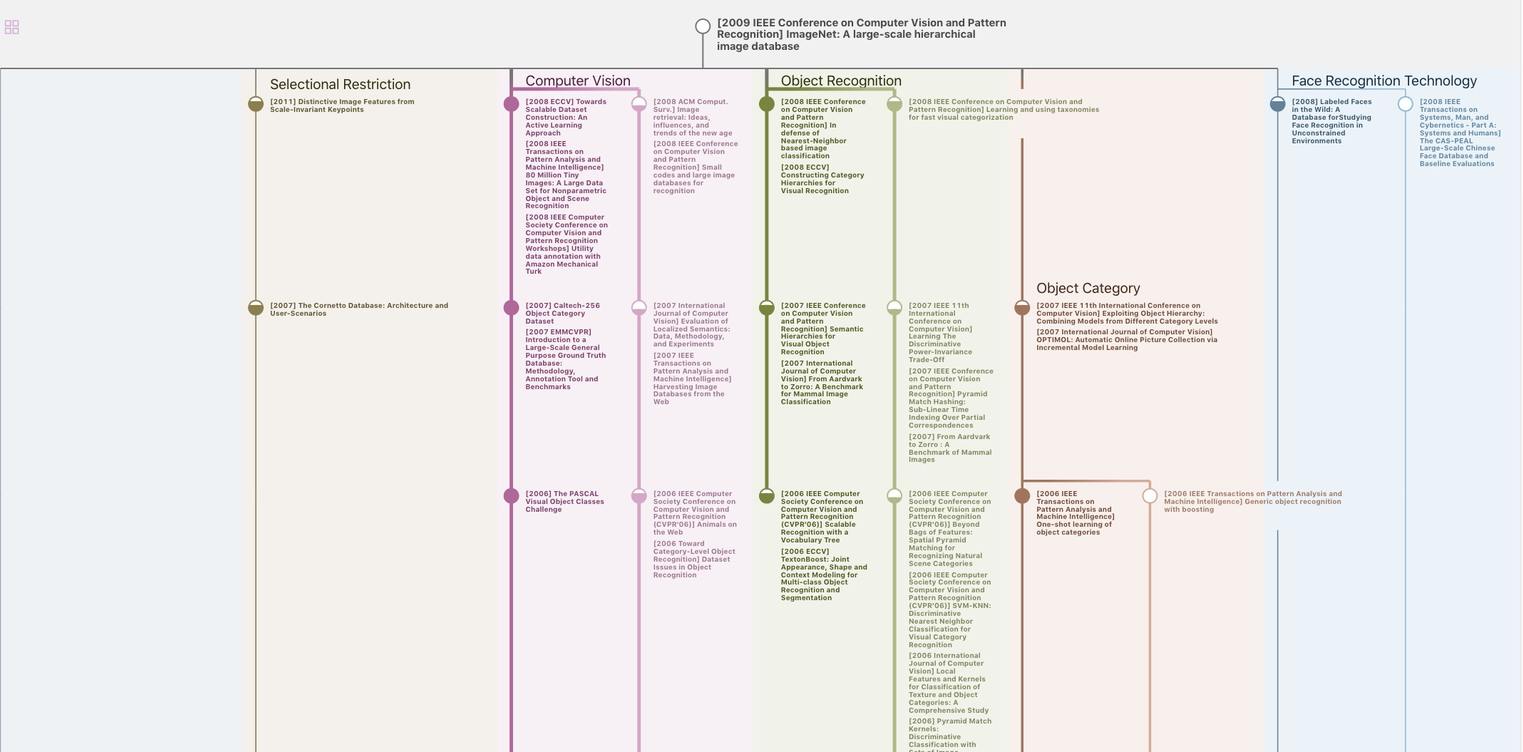
生成溯源树,研究论文发展脉络
Chat Paper
正在生成论文摘要