Clinico-Pathological, Cytogenetic and Molecular Similarities and Differences Between Primary and Secondary Cutaneous Lymphomas
Silvia Uccella,Gaia Goteri,Antonino Maiorana,Valentina Donati,Maria Grazia Tibiletti,Francesca Magnoli,Sofia Facchi, Deborah Merchiori,Erika Morsia,Robel Papotti,Stefania Bettelli,Elisa Forti,Sara Galimberti,Serena Rupoli,Alessandra Filosa,Dimitri Dardanis,Luca Braglia,Samantha Pozzi,Stefano Sacchi Blood(2022)
AI 理解论文
溯源树
样例
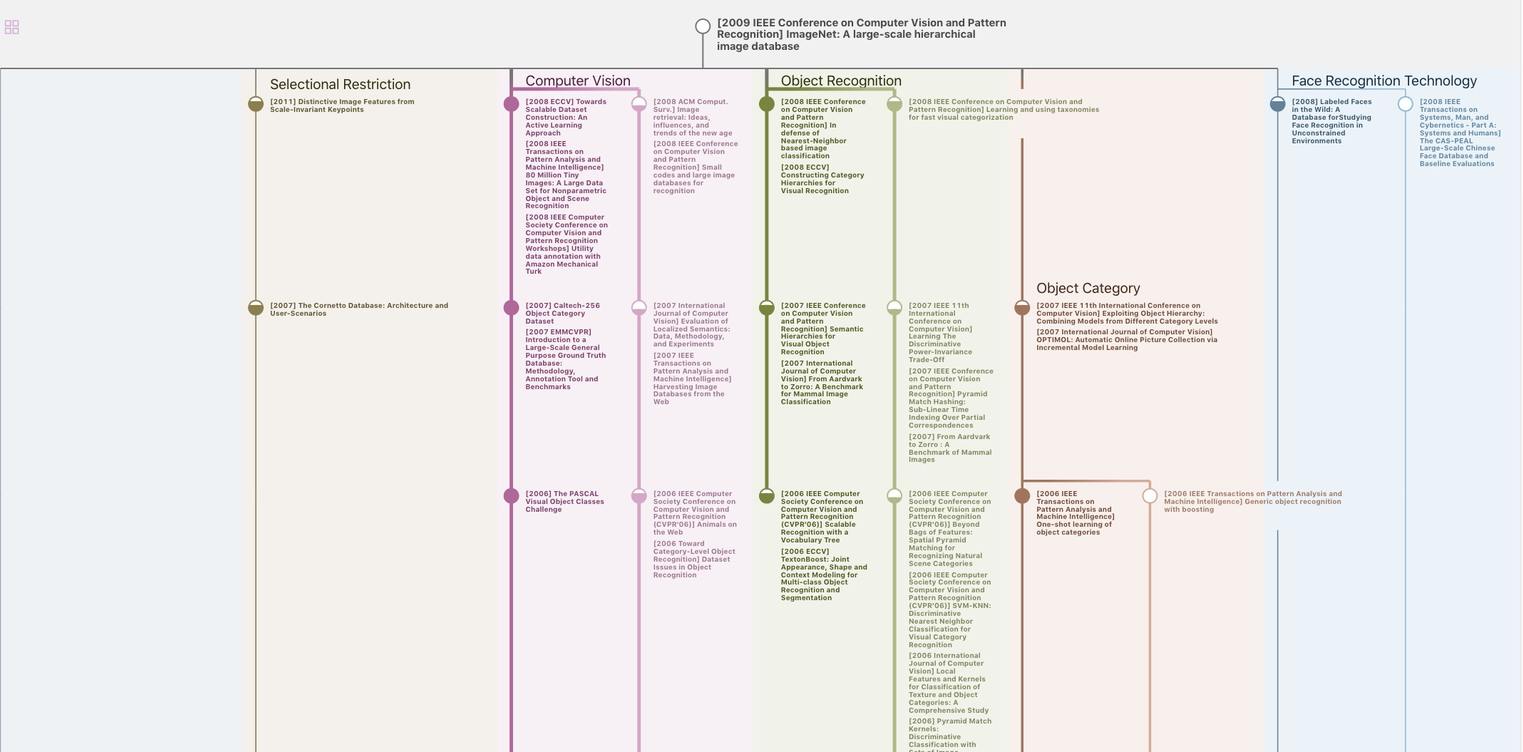