A Point in the Right Direction: Vector Prediction for Spatially-aware Self-supervised Volumetric Representation Learning
arxiv(2022)
摘要
High annotation costs and limited labels for dense 3D medical imaging tasks have recently motivated an assortment of 3D self-supervised pretraining methods that improve transfer learning performance. However, these methods commonly lack spatial awareness despite its centrality in enabling effective 3D image analysis. More specifically, position, scale, and orientation are not only informative but also automatically available when generating image crops for training. Yet, to date, no work has proposed a pretext task that distills all key spatial features. To fulfill this need, we develop a new self-supervised method, VectorPOSE, which promotes better spatial understanding with two novel pretext tasks: Vector Prediction (VP) and Boundary-Focused Reconstruction (BFR). VP focuses on global spatial concepts (i.e., properties of 3D patches) while BFR addresses weaknesses of recent reconstruction methods to learn more effective local representations. We evaluate VectorPOSE on three 3D medical image segmentation tasks, showing that it often outperforms state-of-the-art methods, especially in limited annotation settings.
更多查看译文
关键词
3D Self-Supervised Learning, Representation Learning, Volumetric Medical Image Segmentation
AI 理解论文
溯源树
样例
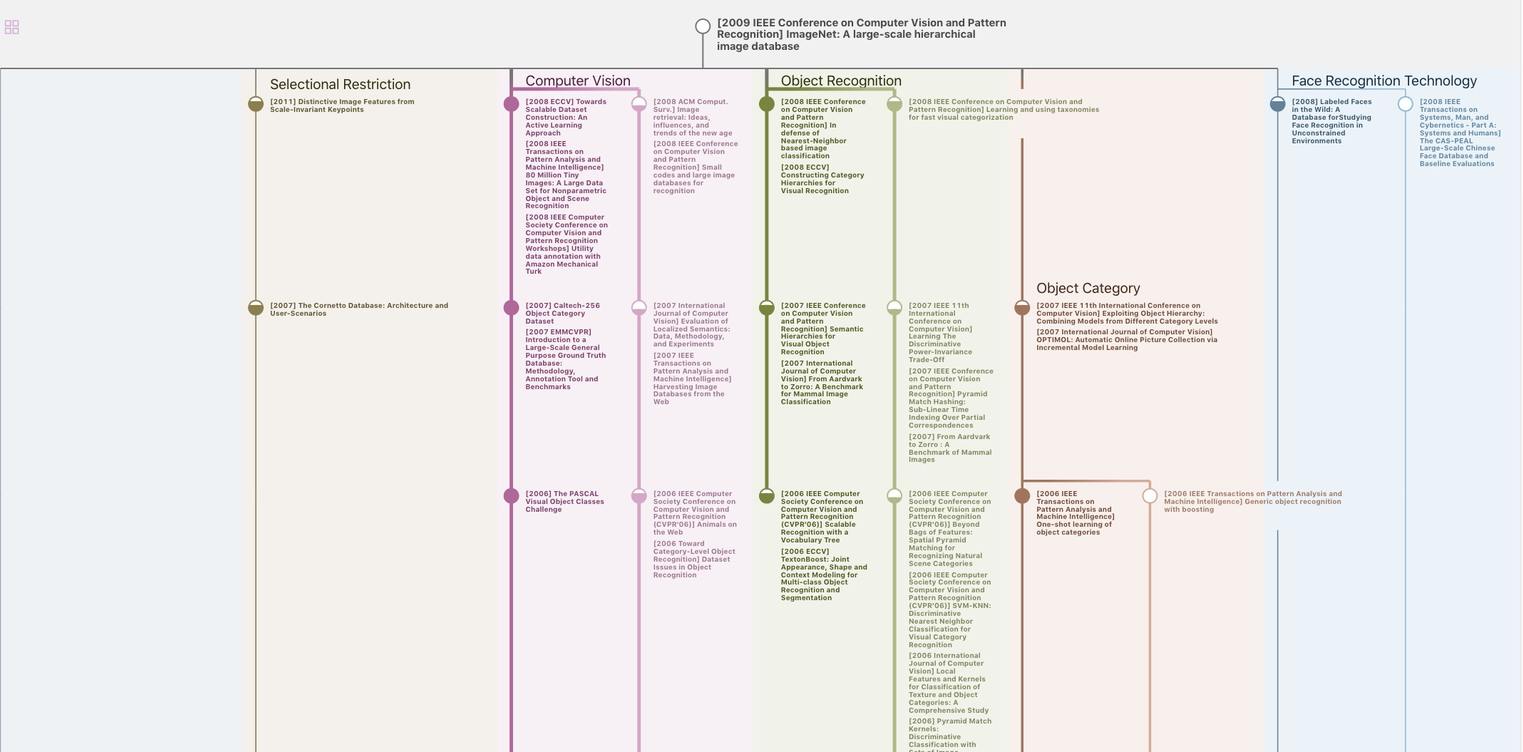
生成溯源树,研究论文发展脉络
Chat Paper
正在生成论文摘要