Towards Computationally Verifiable Semantic Grounding for Language Models
arxiv(2022)
摘要
The paper presents an approach to semantic grounding of language models (LMs) that conceptualizes the LM as a conditional model generating text given a desired semantic message formalized as a set of entity-relationship triples. It embeds the LM in an auto-encoder by feeding its output to a semantic parser whose output is in the same representation domain as the input message. Compared to a baseline that generates text using greedy search, we demonstrate two techniques that improve the fluency and semantic accuracy of the generated text: The first technique samples multiple candidate text sequences from which the semantic parser chooses. The second trains the language model while keeping the semantic parser frozen to improve the semantic accuracy of the auto-encoder. We carry out experiments on the English WebNLG 3.0 data set, using BLEU to measure the fluency of generated text and standard parsing metrics to measure semantic accuracy. We show that our proposed approaches significantly improve on the greedy search baseline. Human evaluation corroborates the results of the automatic evaluation experiments.
更多查看译文
关键词
computationally verifiable semantic grounding,language
AI 理解论文
溯源树
样例
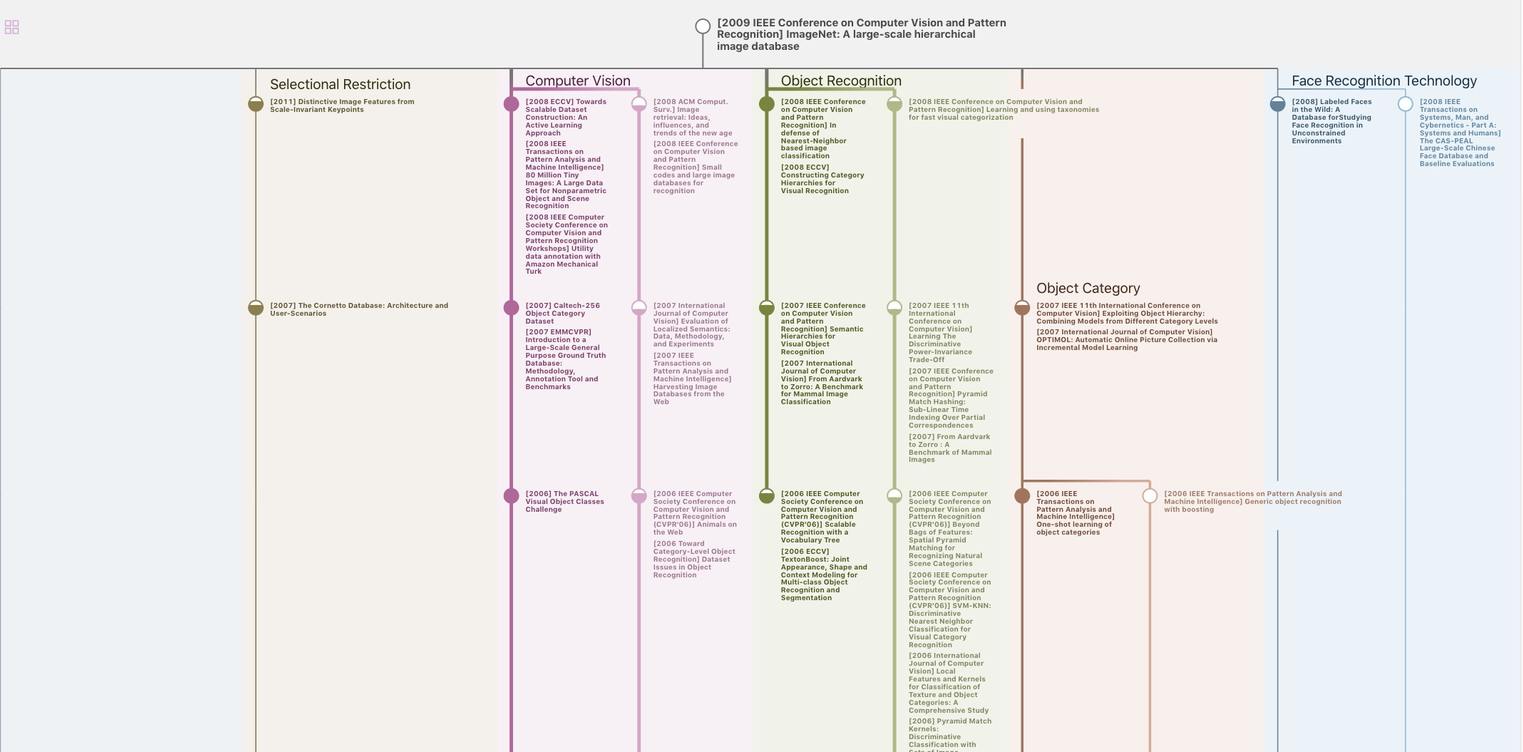
生成溯源树,研究论文发展脉络
Chat Paper
正在生成论文摘要