Blockchain-Based Secure and Efficient Federated Learning with Three-phase Consensus and Unknown Device Selection.
WASA (1)(2022)
摘要
Blockchain-based decentralized federated learning (BCFL) protects data privacy and avoids the single point of failure, which has become a key technology in the Intelligent Internet of Things application. However, the BCFL is still challenged by model attacks from malicious devices. In addition, although the BCFL has many schemes for selecting candidate devices, most of them either assume that devices qualities are known in advance or cannot ensure devices to report information honestly. This will lead to inefficient training in the face of unknown candidate device. To address these issues, we consider two types of model attacks and design "Proof of Model Quality" (PoMQ) to protect the security of BCFL. The PoMQ is a three-phase consensus algorithm, which combines the FedAvg and Multi-Krum algorithms to defend against model update attacks. The PoMQ is also based on the model verification process to resist model aggregation attacks. Moreover, we define the unknown device selection problem as a Combinatorial Multi-Armed Bandit problem (CMAB) to improve the training efficiency, and propose an online learning algorithm based on PoMQ (OLAC) to solve CMAB. Finally, through analysis and extensive experiments, we prove that PoMQ and OLAC can better improve the robustness and training efficiency of BCFL.
更多查看译文
关键词
Blockchain,Federated learning,Consensus algorithm,Multi-armed bandit
AI 理解论文
溯源树
样例
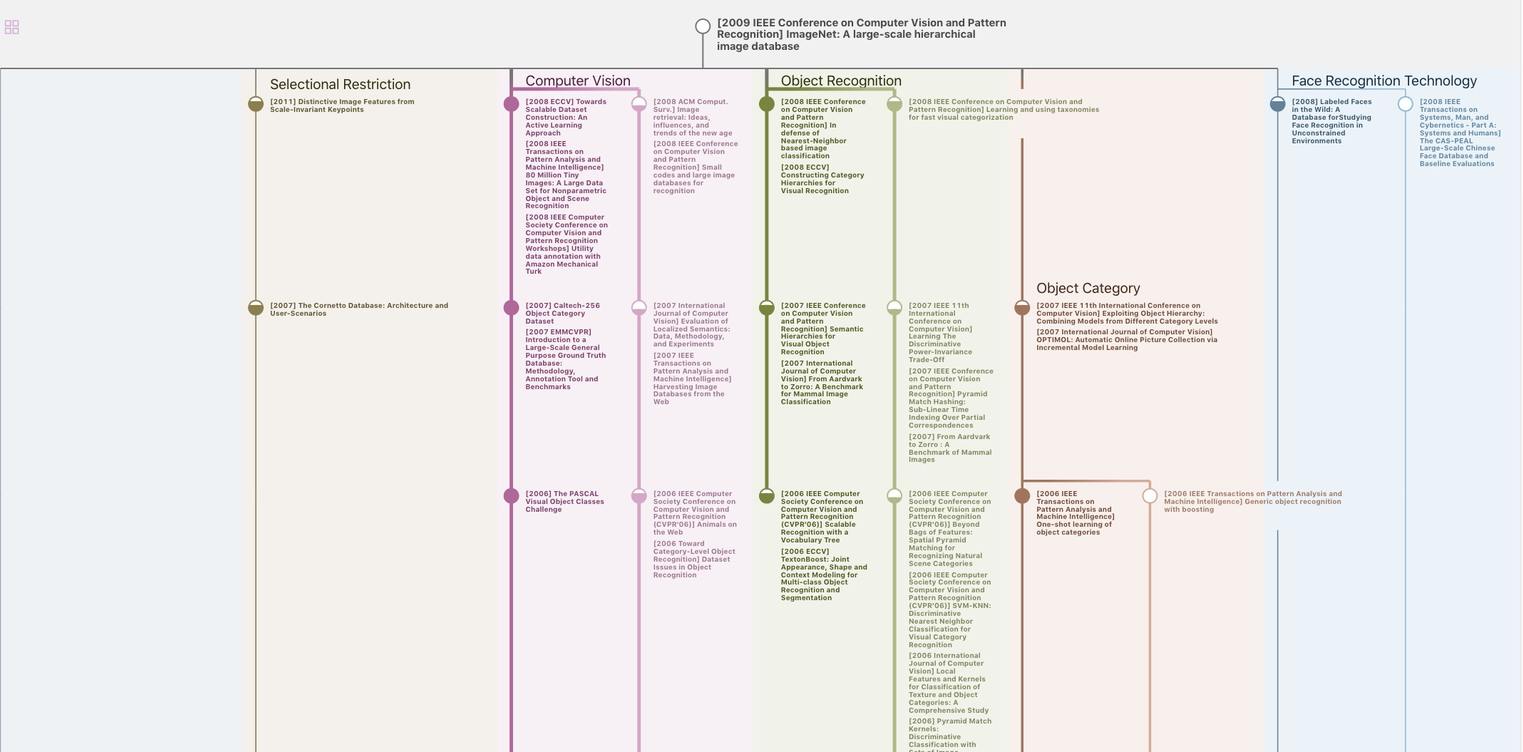
生成溯源树,研究论文发展脉络
Chat Paper
正在生成论文摘要