Hall Effect Sensor Design Optimization With Multi-Physics Informed Gaussian Process Modeling
Volume 3B: 48th Design Automation Conference (DAC)(2022)
摘要
Abstract Magnetic field sensor devices have been widely used to track changes in magnetic flux concentration, and the Hall sensors are promising in many engineering applications. Design optimization of the Hall effect sensor is required to ensure the quality and capability of the device when in service. Even though there has been empirical models established from experiments to guide the design of the Hall effect sensor, the underlying relationship in Hall effect sensor design parameters and corresponding performances has not been looked into thoroughly. This paper presents a physics-informed machine learning technique to optimize the geometry design of Hall magnetic sensors for a low offset and high sensitivity characteristic. Multi-physics based finite element models were first developed to simulate and predict the Hall voltage, offset voltage and sensor sensitivity of different Hall effect sensors with various geometries. In addition, to improve the design efficiency, Gaussian Process (GP) based surrogate models were constructed from multiphysics-based simulation results to effectively investigate the Hall sensor performances with an adaptive sampling strategy. Three types of geometries of Hall sensor were studied and optimized with the proposed physics-informed GP model, the obtained results were consistent with the empirical experimental result.
更多查看译文
AI 理解论文
溯源树
样例
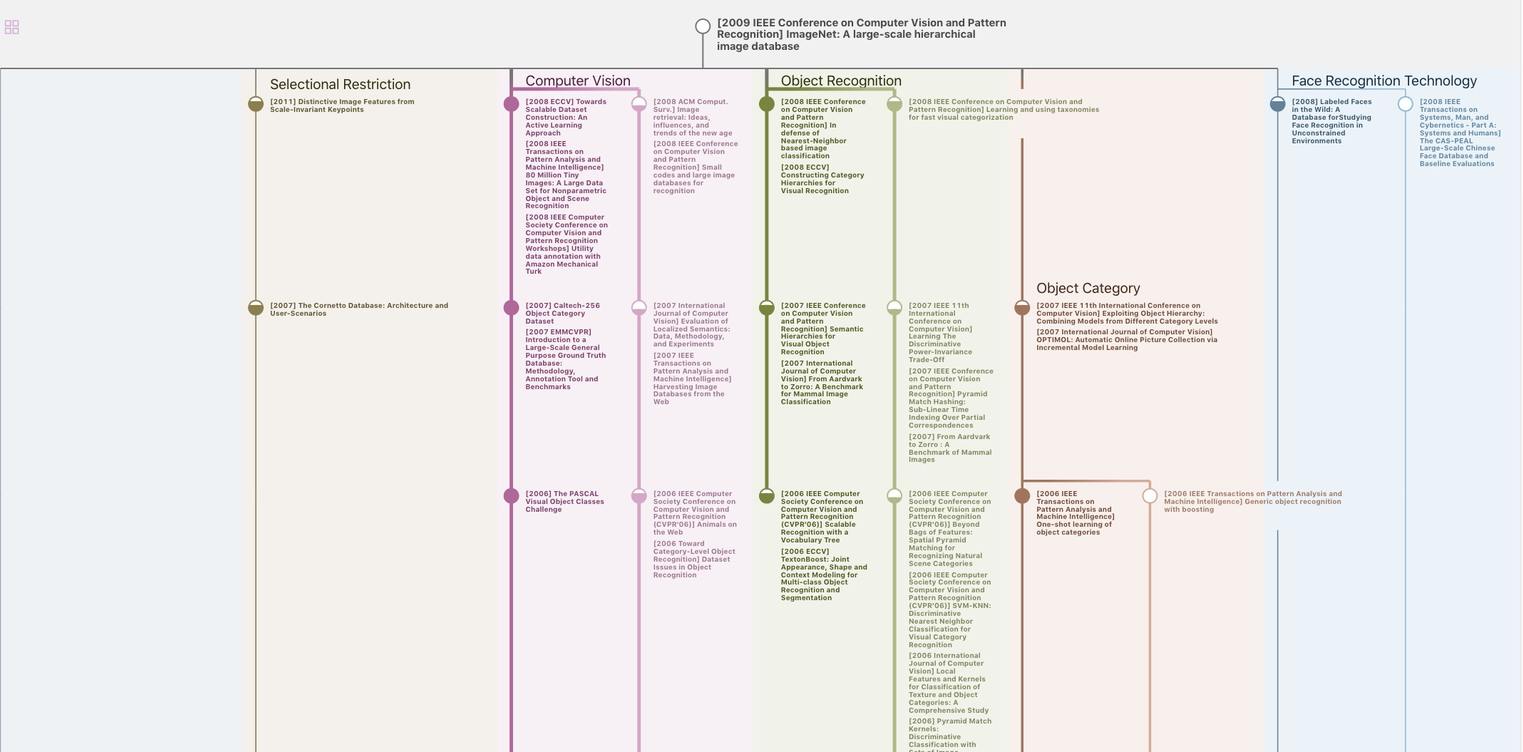
生成溯源树,研究论文发展脉络
Chat Paper
正在生成论文摘要