Decentralized GAN Training Through Diffusion Learning
2022 IEEE 32nd International Workshop on Machine Learning for Signal Processing (MLSP)(2022)
摘要
Most available studies on distributed GAN architectures focus on implementations with a fusion center. In this work, we propose a fully decentralized scheme by employing a diffusion strategy to train a network of GANs. We interpret the training procedure as a team competition problem and use the paradigm of competing adaptive networks to solve it. We explain that the local discriminators and generators will cluster around their respective centroids. We present simulation results to illustrate that the proposed strategy allows local agents to match the performance of the centralized GAN. More importantly, we also illustrate that local GANs are able to generate different types of images from a dataset, even when they are locally trained with a subset that does not contain all image types.
更多查看译文
关键词
Generative adversarial networks,competing diffusion,decentralized training,distributed optimization
AI 理解论文
溯源树
样例
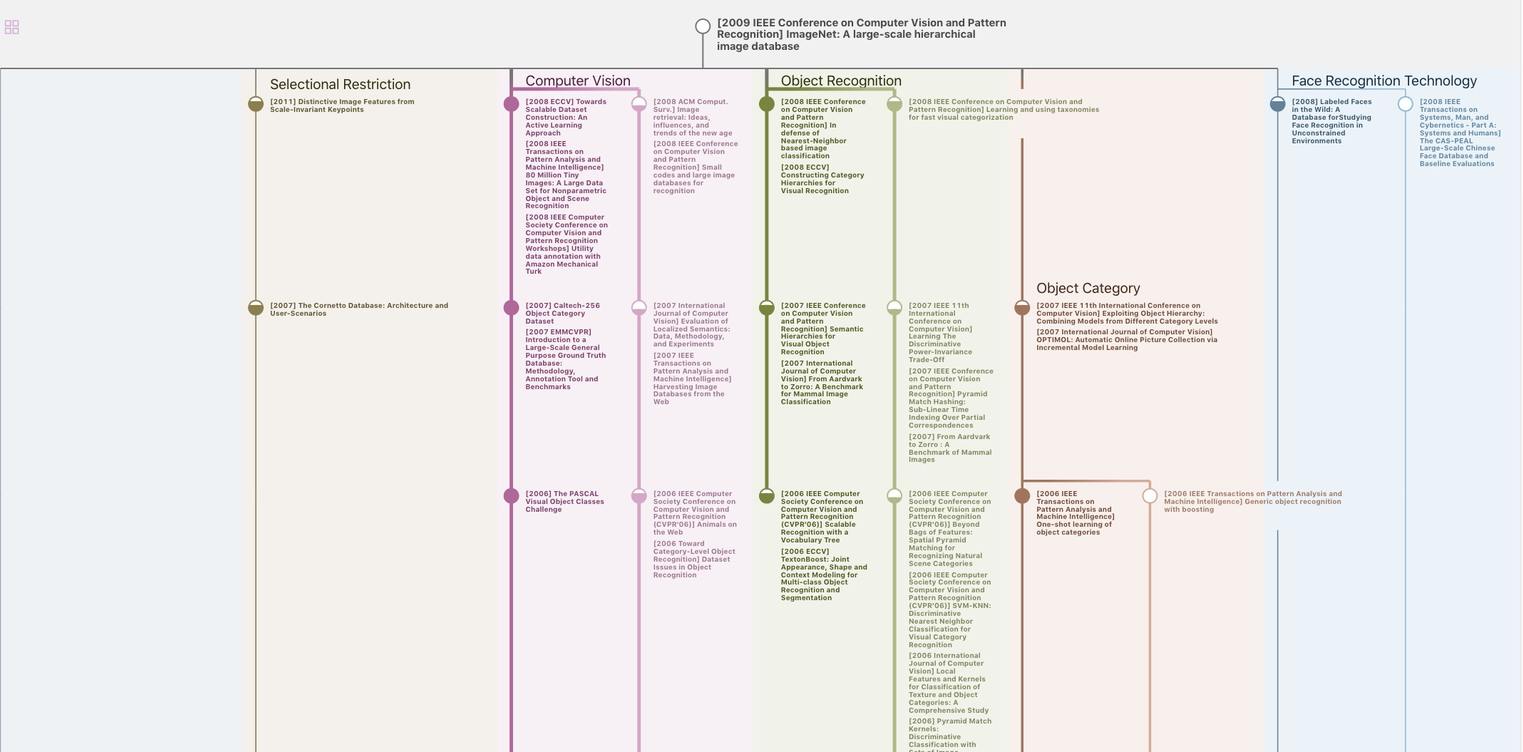
生成溯源树,研究论文发展脉络
Chat Paper
正在生成论文摘要