Deep representation learning determines drug mechanism of action from cell painting images
biorxiv(2023)
摘要
Fluorescent-based microscopy screens carry a broad range of phenotypic information about how compounds affect cellular biology. From changes in cellular morphology observed in these screens, one key area of medicinal interest is determining a compound's mechanism of action. However, much of this phenotypic information is subtle and difficult to quantify. Hence, creating quantitative embeddings that can measure cellular response to compound perturbation has been a key area of research. Here we present a deep learning enabled encoder called MOAProfiler that captures phenotypic features for determining mechanism of action from Cell Painting images. We compared our method with both the traditional gold-standard means of feature encoding via CellProfiler and a deep learning encoder called DeepProfiler. The results, on two independent and biologically different datasets, indicated that MOAProfiler encoded MOA-specific features that allowed for more accurate clustering and classification of compounds over hundreds of different MOAs. Fluorescent-based microscopy screens carry a broad range of phenotypic information about how compounds affect cellular biology.
更多查看译文
关键词
drug,representation,cell,deep,images
AI 理解论文
溯源树
样例
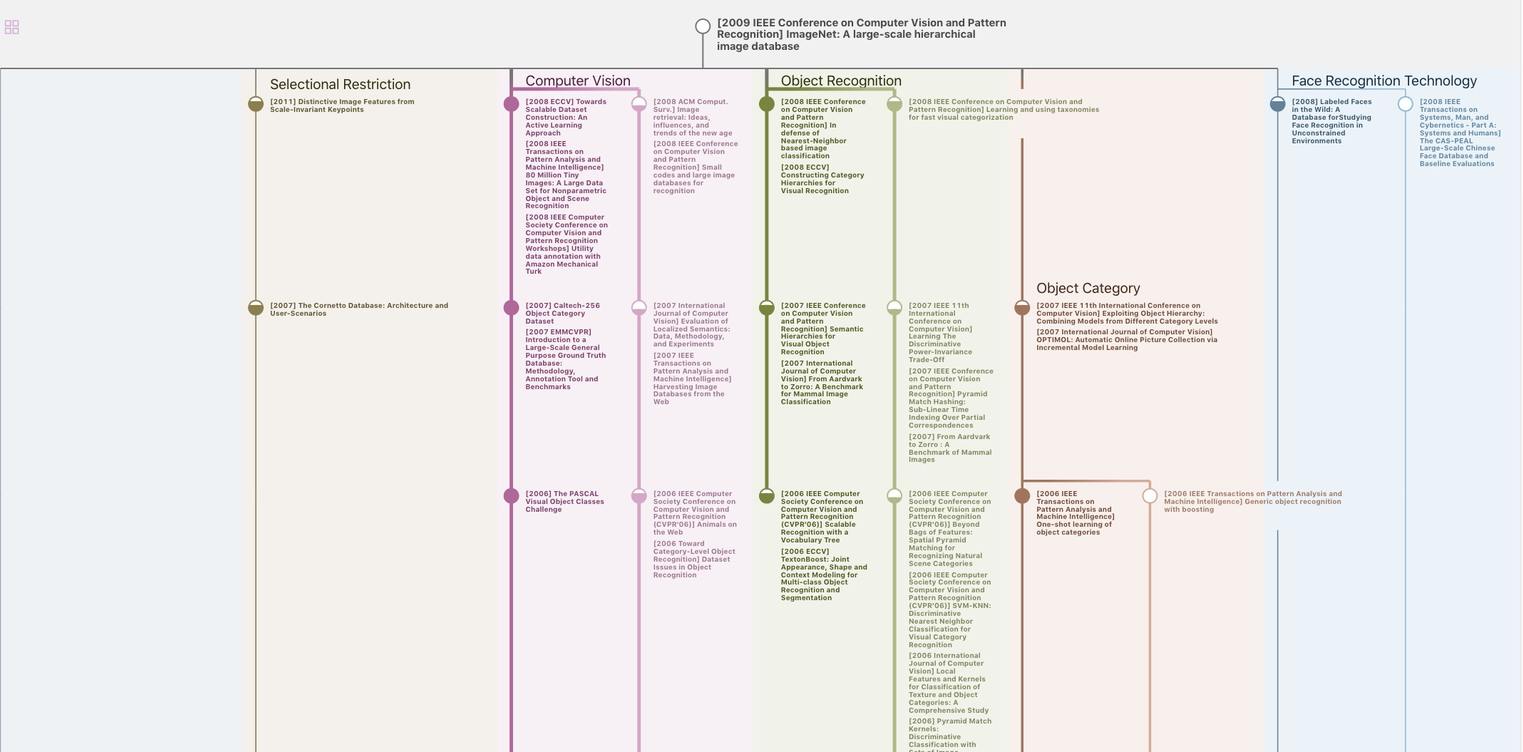
生成溯源树,研究论文发展脉络
Chat Paper
正在生成论文摘要