Explaining COVID-19 diagnosis with Taylor decompositions
NEURAL COMPUTING & APPLICATIONS(2022)
摘要
The COVID-19 pandemic has devastated the entire globe since its first appearance at the end of 2019. Although vaccines are now in production, the number of contaminations remains high, thus increasing the number of specialized personnel that can analyze clinical exams and points out the final diagnosis. Computed tomography and X-ray images are the primary sources for computer-aided COVID-19 diagnosis, but we still lack better interpretability of such automated decision-making mechanisms. This manuscript presents an insightful comparison of three approaches based on explainable artificial intelligence (XAI) to light up interpretability in the context of COVID-19 diagnosis using deep networks: Composite Layer-wise Propagation, Single Taylor Decomposition, and Deep Taylor Decomposition. Two deep networks have been used as the backbones to assess the explanation skills of the XAI approaches mentioned above: VGG11 and VGG16. We hope that such work can be used as a basis for further research on XAI and COVID-19 diagnosis for each approach figures its own positive and negative points.
更多查看译文
关键词
Explainable artificial intelligence,Deep Taylor expansion,COVID-19,Machine learning
AI 理解论文
溯源树
样例
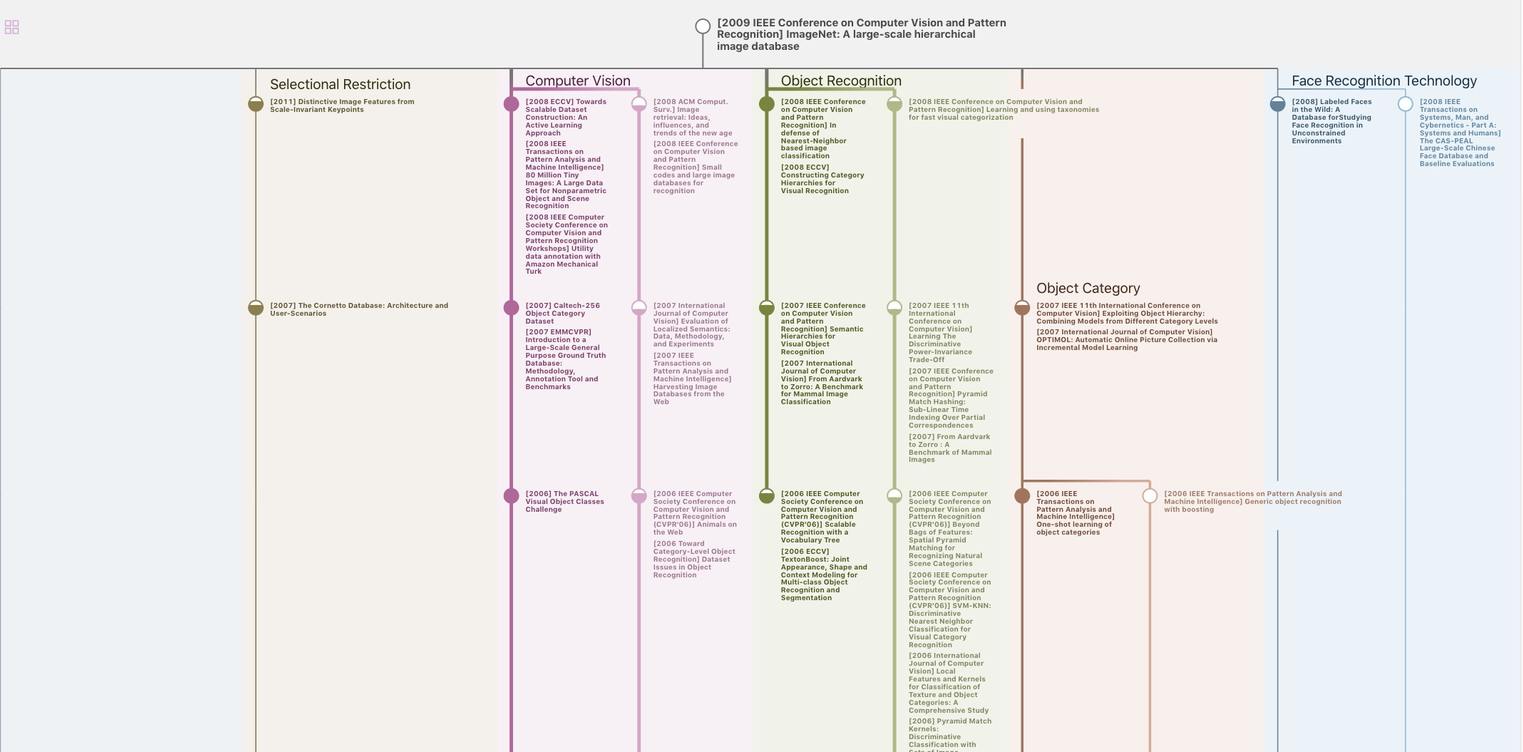
生成溯源树,研究论文发展脉络
Chat Paper
正在生成论文摘要