Identification of Optimal Starting Time Instance to Forecast Net Blotch Density in Spring Barley with Meteorological Data in Finland
Agriculture(2022)
摘要
The performance of meteorological data-based methods to forecast plant diseases strongly depends on temporal weather information. In this paper, a data analysis procedure is presented for finding the optimal starting time for forecasting net blotch density in spring barley based on meteorological data. For this purpose, changes in the information content of typically measured weather variables were systemically quantified in sliding time windows and with additionally generated mathematical transformations, namely with features. Signal-to-noise statistics were applied in a novel way as a metric for identifying the optimal starting time instance and the most important features to successfully distinguish between two net blotch densities during springtime itself. According to the results, the information content of meteorological data used in classifying between nine years with and four years without net blotch reached its maximum in Finnish weather conditions on the 41st day from the beginning of the growing season. Specifically, utilising weather data at 41–55 days from the beginning of the growing season maximises successful forecasting potential of net blotch density. It also seems that this time instance enables a linear classification task with a selected feature subset, since the averages of the metrics in two data groups differ statistically with a minimum 68% confidence level for nine days in a 14-day time window.
更多查看译文
关键词
advanced data analysis,feature generation,plant disease prediction,signal-to-noise statistics,modern agriculture
AI 理解论文
溯源树
样例
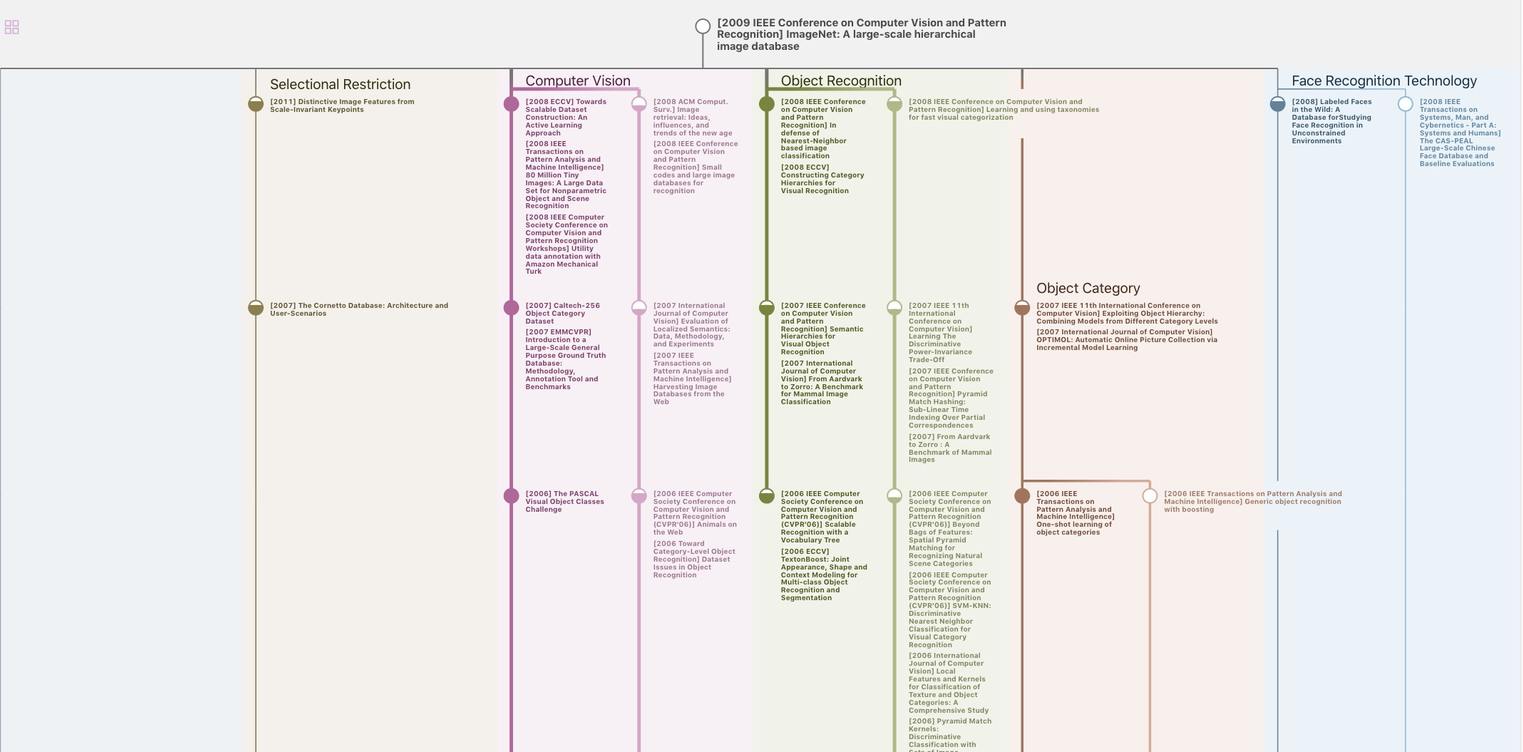
生成溯源树,研究论文发展脉络
Chat Paper
正在生成论文摘要