WilDect-YOLO: An efficient and robust computer vision-based accurate object localization model for automated endangered wildlife detection
Ecological Informatics(2023)
Abstract
Objective. With climatic instability, various ecological disturbances, and human actions threaten the existence of various endangered wildlife species. Therefore, an up-to-date accurate and detailed detection process plays an important role in protecting biodiversity losses, conservation, and ecosystem management. Current state-of-the-art wildlife detection models, however, often lack superior feature extraction capability in complex environ-ments, limiting the development of accurate and reliable detection models. Method. To this end, we present WilDect-YOLO, a deep learning (DL)-based automated high-performance detection model for real-time endan-gered wildlife detection. In the model, we introduce a residual block in the CSPDarknet53 backbone for strong and discriminating deep spatial features extraction and integrate DenseNet blocks to improve in preserving critical feature information. To enhance receptive field representation, preserve fine-grain localized information, and improve feature fusion, a Spatial Pyramid Pooling (SPP) and modified Path Aggregation Network (PANet) have been implemented that results in superior detection under various challenging environments. Results. Evaluating the model performance in a custom endangered wildlife dataset considering high variability and complex backgrounds, WilDect-YOLO obtains a mean average precision (mAP) value of 96.89%, F1-score of 97.87%, and precision value of 97.18% at a detection rate of 59.20 FPS outperforming current state-of-the-art models. Significance. The present research provides an effective and efficient detection framework addressing the shortcoming of existing DL-based wildlife detection models by providing highly accurate species-level localized bounding box prediction. Current work constitutes a step toward a non-invasive, fully automated an-imal observation system in real-time in-field applications.
MoreTranslated text
Key words
Endangered wildlife detection,You only look once (YOLOv4) algorithm,Object detection (OD),Computer vision,Deep learning (DL),Wildlife preservation
AI Read Science
Must-Reading Tree
Example
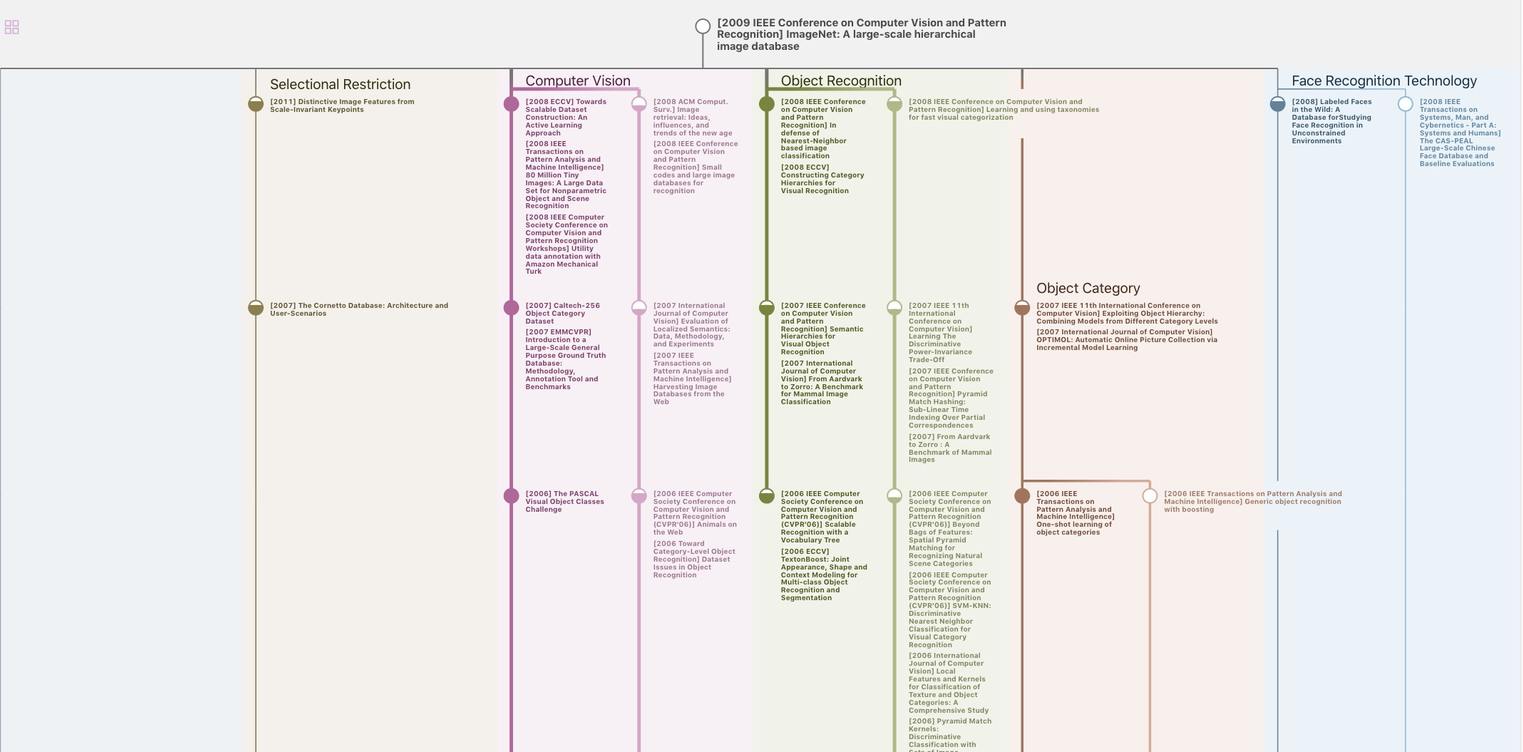
Generate MRT to find the research sequence of this paper
Chat Paper
Summary is being generated by the instructions you defined