Deep learning based distributed scatterers acceleration approach: Distributed scatterers prediction Net
INTERNATIONAL JOURNAL OF APPLIED EARTH OBSERVATION AND GEOINFORMATION(2022)
摘要
Distributed scatter (DS) interferometric synthetic aperture radar is a powerful technology for analyzing dis-placements of the earth's surface. Unfortunately, the preparatory step of DS pre-processing is enormously time consuming. The present research puts forward a deep learning-based approach called Distributed Scatterers Prediction Net (DSPN), that can reduce the computational load considerably. DSPN is a convolutional neural network, which generates DS candidate masks based on nine input layers. Masked pixels with low prospect of being DS are omitted during DS pre-processing. Tests on 6 different terrains in North Rhine-Westphalia and Sicily with Sentinel-1 data show that DSPN saves 11% to 87% computation time depending on the scene without significantly reducing coverage with information. Our experiments show that the proposed approach can effectively predict DS candidates and speeds up processing, indicating its potential for analyzing the big data of remote sensing. To the best of our knowledge, this is the first attempt to do a classification in DS candidates and non-DS candidates as a preparatory step to DS pre-processing.
更多查看译文
关键词
Deep Learning,InSAR,Distributed Scatterer,CNN,DSPN
AI 理解论文
溯源树
样例
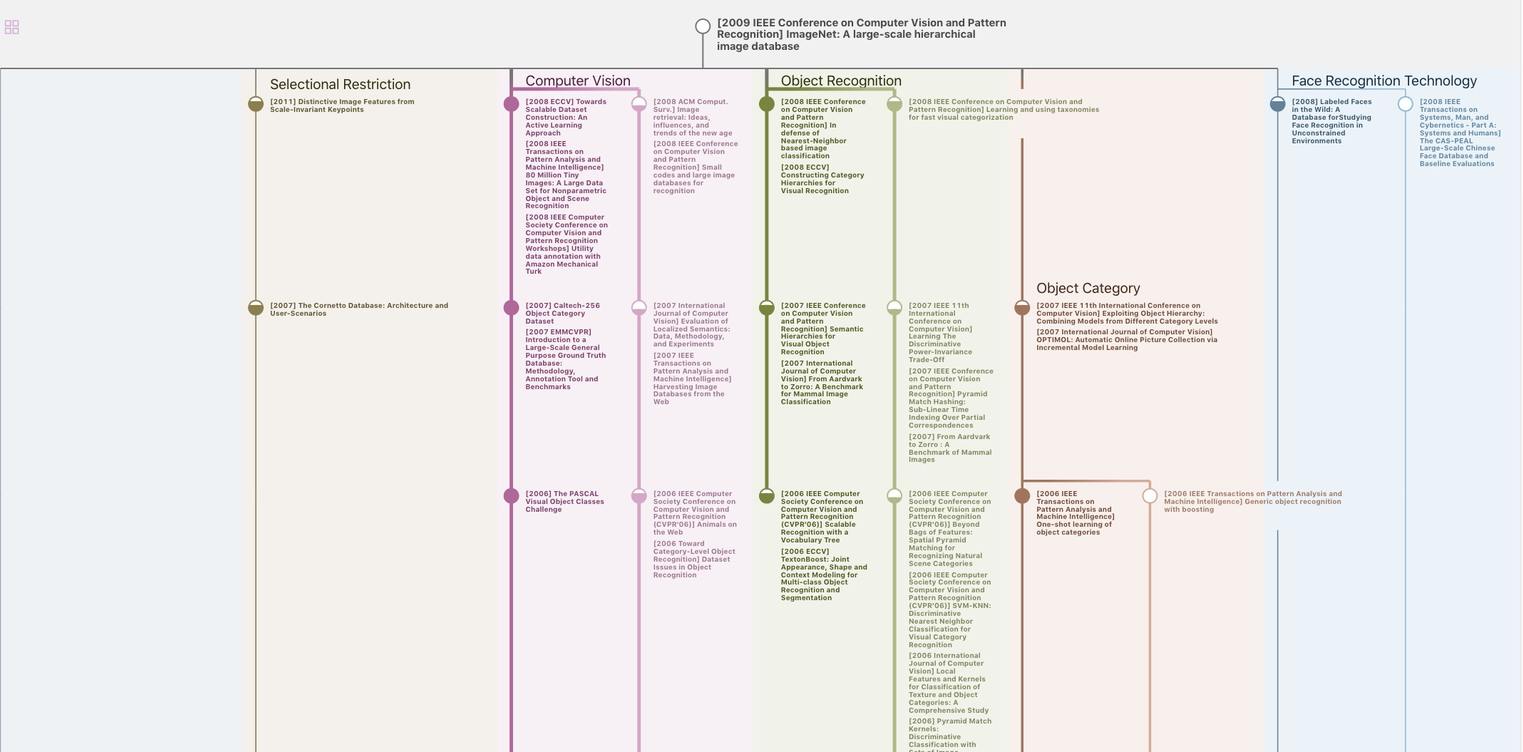
生成溯源树,研究论文发展脉络
Chat Paper
正在生成论文摘要