Computer Vision Enabled Segmentation of Kidney Stones During Ureteroscopy and Laser Lithotripsy.
Journal of endourology(2023)
摘要
Objective: To evaluate the performance of computer vision models for automated kidney stone segmentation during flexible ureteroscopy and laser lithotripsy.Materials and Methods: We collected 20 ureteroscopy videos of intrarenal kidney stone treatment and extracted frames (N = 578) from these videos. We manually annotated kidney stones on each frame. Eighty percent of the data were used to train three standard computer vision models (U-Net, U-Net++, and DenseNet) for automatic stone segmentation during flexible ureteroscopy. The remaining data (20%) were used to compare performance of the three models after optimization through Dice coefficients and binary cross entropy. We identified the highest performing model and evaluated automatic segmentation performance during ureteroscopy for both stone localization and treatment using a separate set of endoscopic videos. We evaluated performance of the pixel-based analysis using area under the receiver operating characteristic curve (AUC-ROC), accuracy, sensitivity, and positive predictive value both in previously recorded videos and in real time.Results: A computer vision model (U-Net++) was evaluated, trained, and optimized for kidney stone segmentation during ureteroscopy using 20 surgical videos (mean video duration of 22 seconds, standard deviation +/- 13 seconds). The model showed good performance for stone localization with both digital ureteroscopes (AUC-ROC: 0.98) and fiberoptic ureteroscopes (AUC-ROC: 0.93). Furthermore, the model was able to accurately segment stones and stone fragments <270 mu m in diameter during laser fragmentation (AUC-ROC: 0.87) and dusting (AUC-ROC: 0.77). The model automatically annotated videos intraoperatively in three cases and could do so in real time at 30 frames per second (FPS).Conclusion: Computer vision models demonstrate strong performance for automatic stone segmentation during ureteroscopy. Automatically annotating new videos at 30 FPS demonstrate the feasibility of real-time application during surgery, which could facilitate tracking tools for stone treatment.
更多查看译文
关键词
ureteroscopy,machine learning,computer vision,nephrolithiasis
AI 理解论文
溯源树
样例
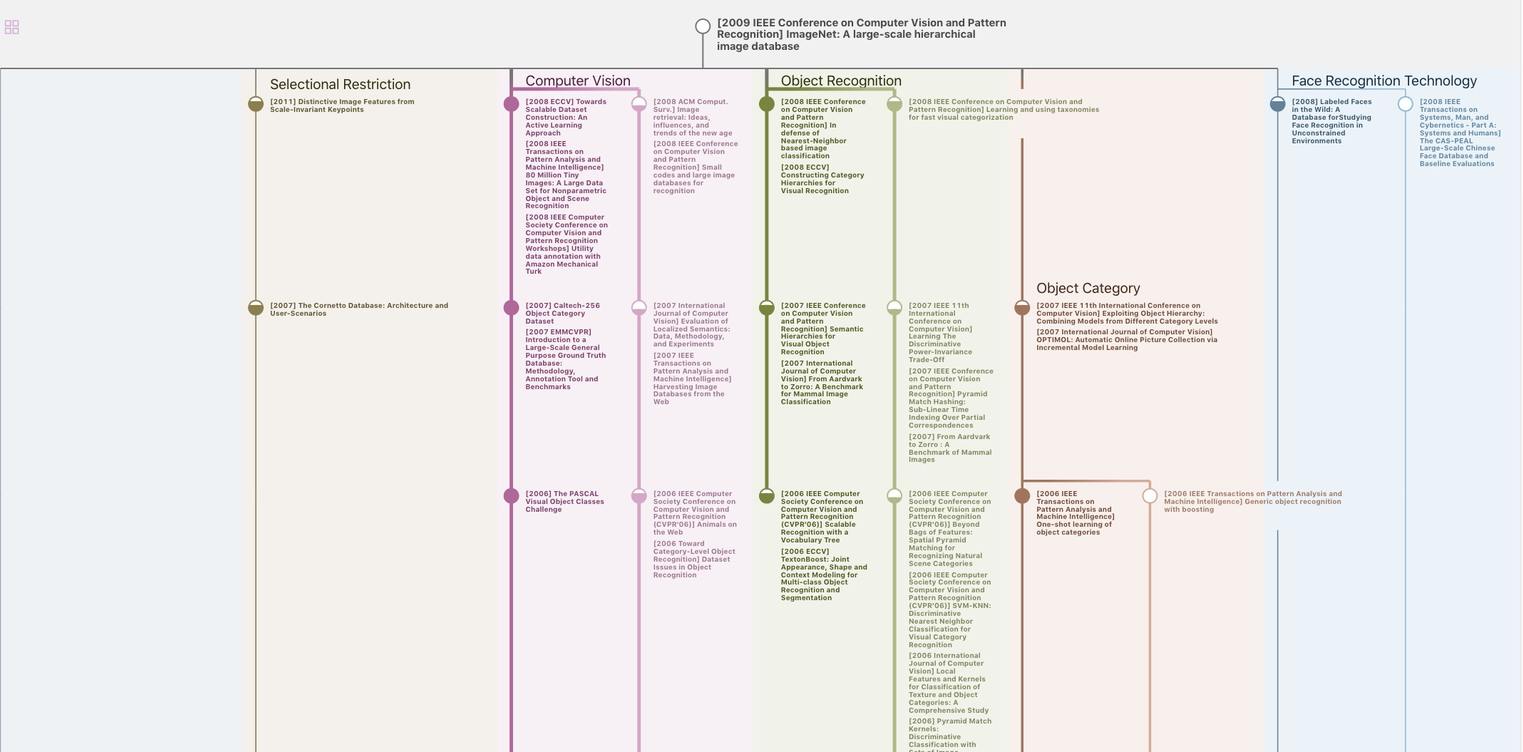
生成溯源树,研究论文发展脉络
Chat Paper
正在生成论文摘要