A Unified Approach to Differentially Private Bayes Point Estimation
IFAC PAPERSONLINE(2023)
摘要
Parameter estimation in statistics and system identification relies on data that may contain sensitive information. To protect this sensitive information, the notion of differential privacy (DP) has been proposed, which enforces confidentiality by introducing randomization in the estimates. Standard algorithms for differentially private estimation are based on adding an appropriate amount of noise to the output of a traditional point estimation method. This leads to an accuracy-privacy trade off, as adding more noise reduces the accuracy while increasing privacy. In this paper, we propose a new Unified Bayes Private Point (UBaPP) approach to Bayes point estimation of the unknown parameters of a data generating mechanism under a DP constraint, that achieves a better accuracy-privacy trade off than traditional approaches. We verify the performance of our approach on a simple numerical example. Copyright (c) 2023 The Authors. This is an open access article under the CC BY-NC-ND license (https://creativecommons.org/licenses/by-nc-nd/4.0/)
更多查看译文
关键词
Differential privacy,Parameter estimation,Bayes point estimation
AI 理解论文
溯源树
样例
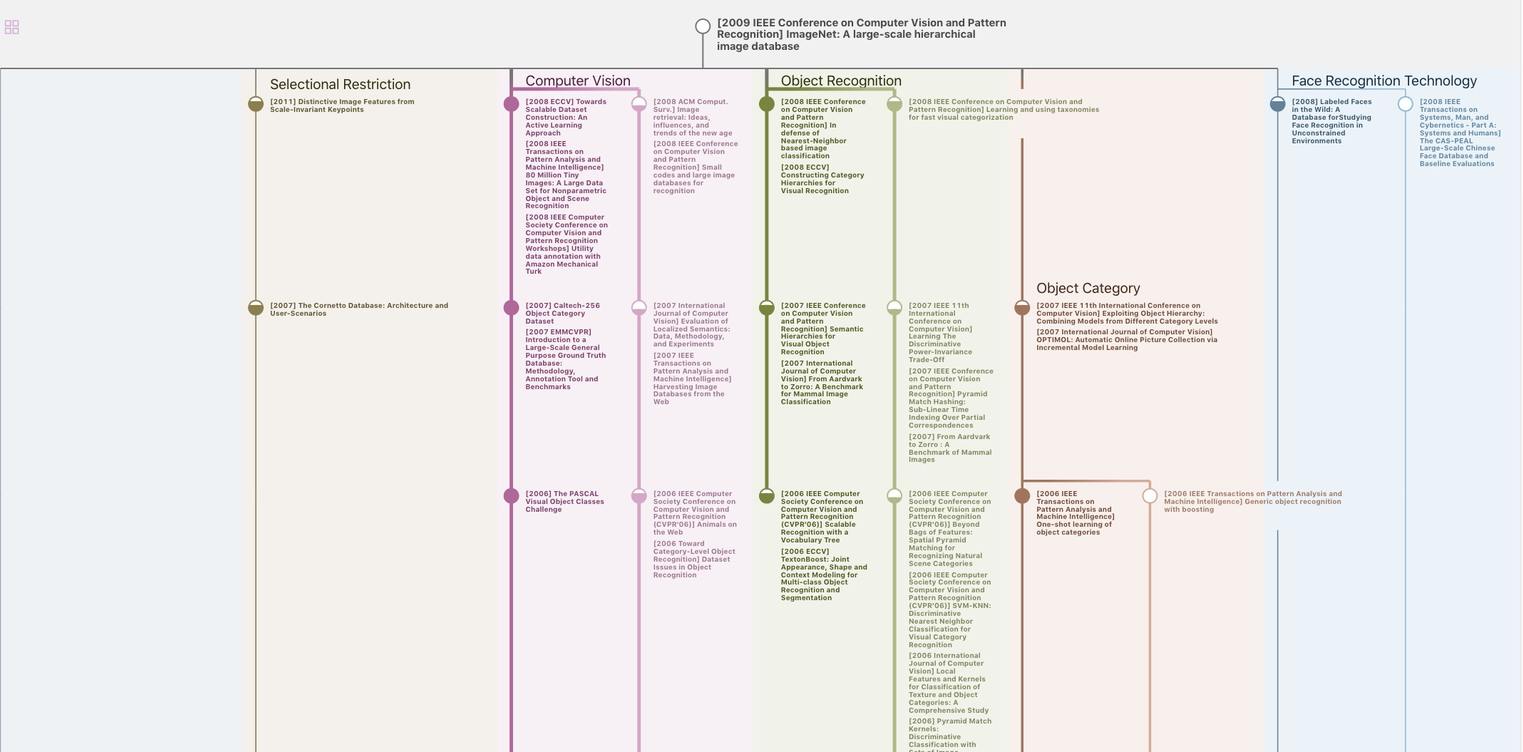
生成溯源树,研究论文发展脉络
Chat Paper
正在生成论文摘要