Reconstructing the exit wave of 2D materials in high-resolution transmission electron microscopy using machine learning.
Ultramicroscopy(2022)
摘要
Reconstruction of the exit wave function is an important route to interpreting high-resolution transmission electron microscopy (HRTEM) images. Here we demonstrate that convolutional neural networks can be used to reconstruct the exit wave from a short focal series of HRTEM images, with a fidelity comparable to conventional exit wave reconstruction. We use a fully convolutional neural network based on the U-Net architecture, and demonstrate that we can train it on simulated exit waves and simulated HRTEM images of graphene-supported molybdenum disulphide (an industrial desulfurization catalyst). We then apply the trained network to analyse experimentally obtained images from similar samples, and obtain exit waves that clearly show the atomically resolved structure of both the MoS nanoparticles and the graphene support. We also show that it is possible to successfully train the neural networks to reconstruct exit waves for 3400 different two-dimensional materials taken from the Computational 2D Materials Database of known and proposed two-dimensional materials.
更多查看译文
关键词
2D materials,Exit wave reconstruction,HRTEM,Machine learning
AI 理解论文
溯源树
样例
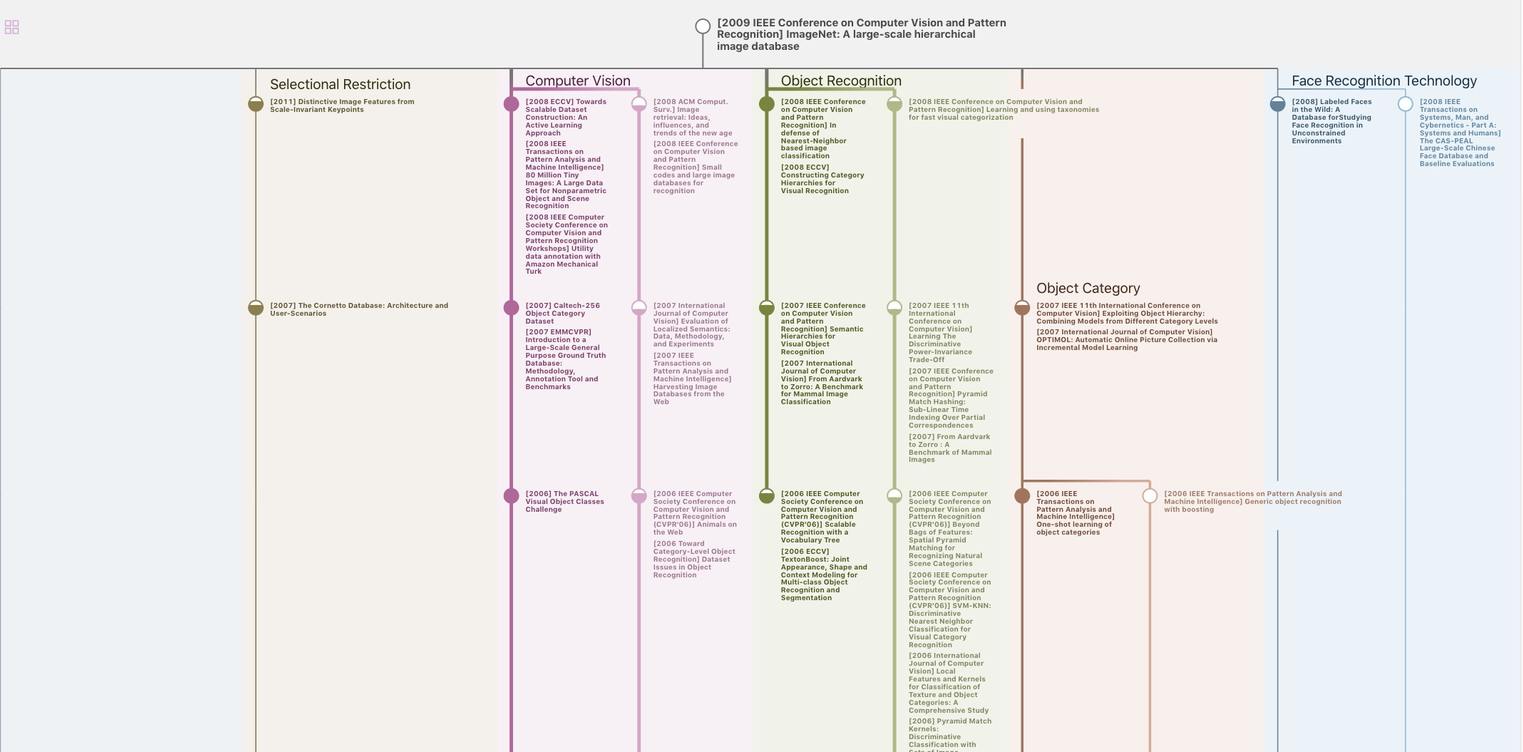
生成溯源树,研究论文发展脉络
Chat Paper
正在生成论文摘要