Predictive Modeling of Above-Ground Biomass in Brachiaria Pastures from Satellite and UAV Imagery Using Machine Learning Approaches
REMOTE SENSING(2022)
摘要
Grassland pastures are crucial for the global food supply through their milk and meat production; hence, forage species monitoring is essential for cattle feed. Therefore, knowledge of pasture above-ground canopy features help understand the crop status. This paper finds how to construct machine learning models to predict above-ground canopy features in Brachiaria pasture from ground truth data (GTD) and remote sensing at larger (satellite data on the cloud) and smaller (unmanned aerial vehicles (UAV)) scales. First, we used above-ground biomass (AGB) data obtained from Brachiaria to evaluate the relationship between vegetation indices (VIs) with the dry matter (DM). Next, the performance of machine learning algorithms was used for predicting AGB based on VIs obtained from ground truth and satellite and UAV imagery. When comparing more than twenty-five machine learning models using an Auto Machine Learning Python API, the results show that the best algorithms were the Huber with R-2 = 0.60, Linear with R-2 = 0.54, and Extra Trees with R-2 = 0.45 to large scales using satellite. On the other hand, short-scale best regressions are K Neighbors with an R-2 of 0.76, Extra Trees with an R-2 of 0.75, and Bayesian Ridge with an R-2 of 0.70, demonstrating a high potential to predict AGB and DM. This study is the first prediction model approach that assesses the rotational grazing system and pasture above-ground canopy features to predict the quality and quantity of cattle feed to support pasture management in Colombia.
更多查看译文
关键词
above-ground biomass,precision agriculture,UAV,remote sensing,machine learning prediction
AI 理解论文
溯源树
样例
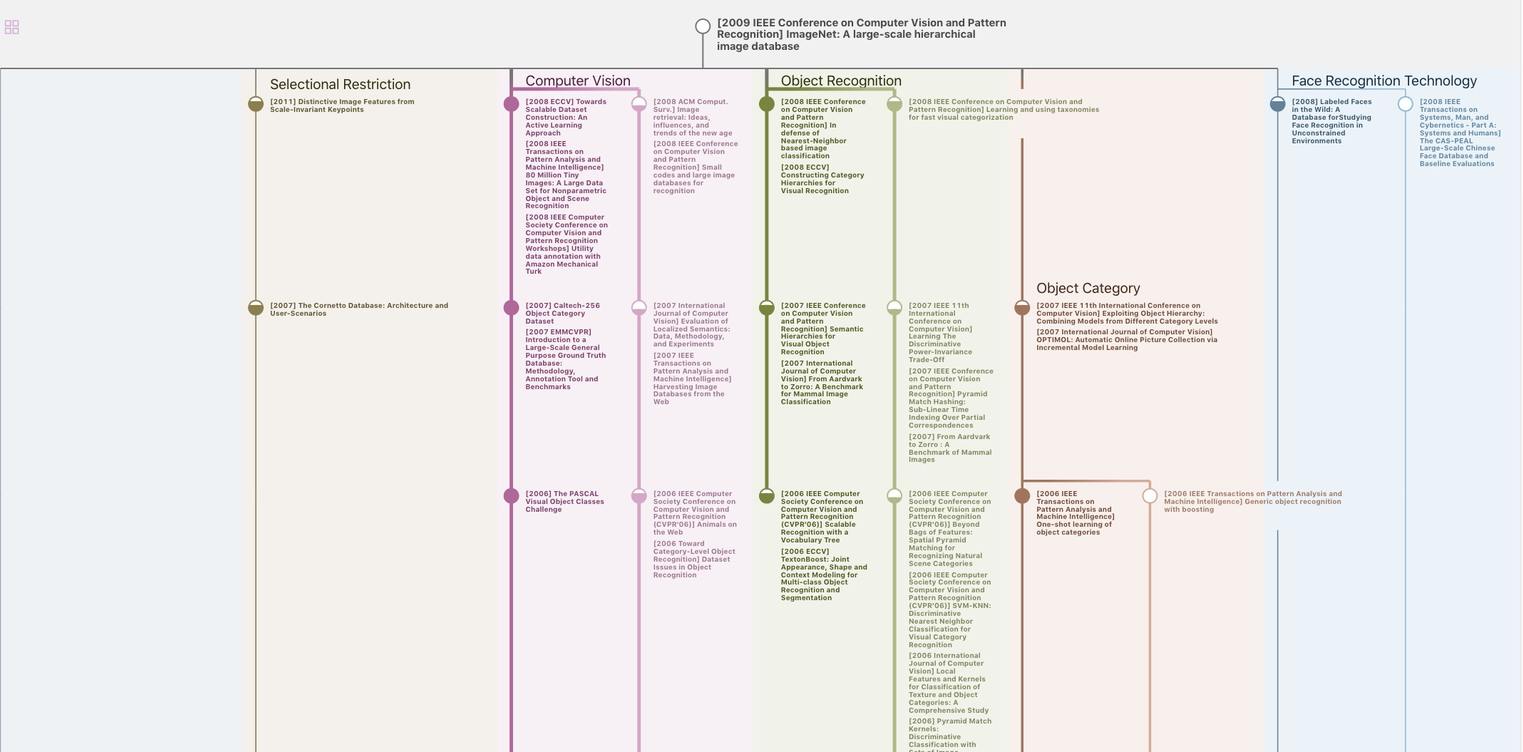
生成溯源树,研究论文发展脉络
Chat Paper
正在生成论文摘要