You Need Multiple Exiting: Dynamic Early Exiting for Accelerating Unified Vision Language Model
CVPR 2023(2023)
摘要
Large-scale transformer models bring significant improvements for various downstream vision language tasks with a unified architecture. The performance improvements come with increasing model size, resulting in slow inference speed and increased cost for severing. While some certain predictions benefit from the full complexity of the large-scale model, not all of input need the same amount of computation to conduct, potentially leading to computation resource waste. To handle this challenge, early exiting is proposed to adaptively allocate computational power in term of input complexity to improve inference efficiency. The existing early exiting strategies usually adopt output confidence based on intermediate layers as a proxy of input complexity to incur the decision of skipping following layers. However, such strategies cannot apply to encoder in the widely-used unified architecture with both encoder and decoder due to difficulty of output confidence estimation in the encoder. It is suboptimal in term of saving computation power to ignore the early exiting in encoder component. To handle this challenge, we propose a novel early exiting strategy for unified visual language models, which allows dynamically skip the layers in encoder and decoder simultaneously in term of input layer-wise similarities with multiple times of early exiting, namely MuE. By decomposing the image and text modalities in the encoder, MuE is flexible and can skip different layers in term of modalities, advancing the inference efficiency while minimizing performance drop. Experiments on the SNLI-VE and MS COCO datasets show that the proposed approach MuE can reduce inference time by up to 50% and 40% while maintaining 99% and 96% performance respectively.
更多查看译文
关键词
Efficient and scalable vision
AI 理解论文
溯源树
样例
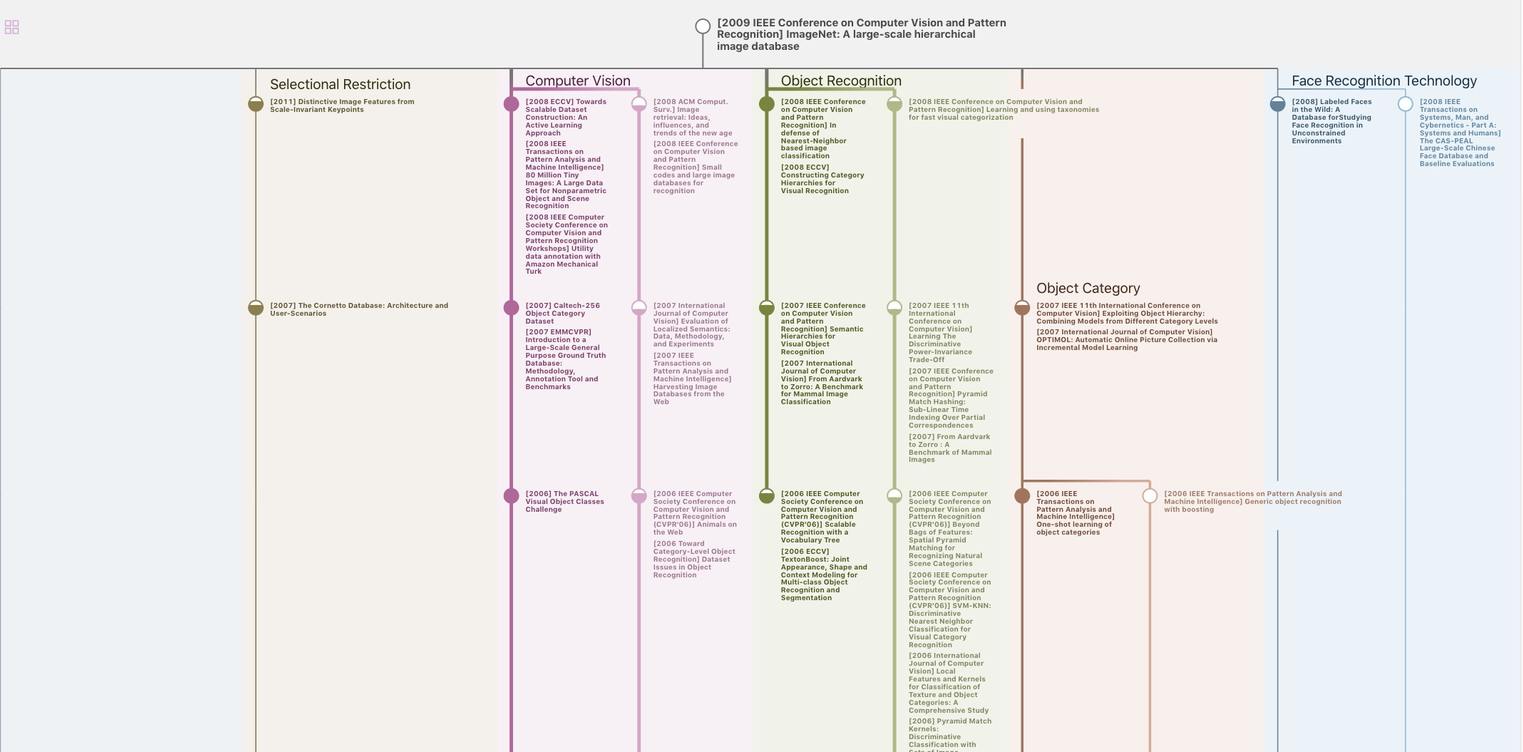
生成溯源树,研究论文发展脉络
Chat Paper
正在生成论文摘要