Penalizing the Hard Example but Not Too Much: A Strong Baseline for Fine-Grained Visual Classification.
IEEE transactions on neural networks and learning systems(2024)
摘要
Though significant progress has been achieved on fine-grained visual classification (FGVC), severe overfitting still hinders model generalization. A recent study shows that hard samples in the training set can be easily fit, but most existing FGVC methods fail to classify some hard examples in the test set. The reason is that the model overfits those hard examples in the training set, but does not learn to generalize to unseen examples in the test set. In this article, we propose a moderate hard example modulation (MHEM) strategy to properly modulate the hard examples. MHEM encourages the model to not overfit hard examples and offers better generalization and discrimination. First, we introduce three conditions and formulate a general form of a modulated loss function. Second, we instantiate the loss function and provide a strong baseline for FGVC, where the performance of a naive backbone can be boosted and be comparable with recent methods. Moreover, we demonstrate that our baseline can be readily incorporated into the existing methods and empower these methods to be more discriminative. Equipped with our strong baseline, we achieve consistent improvements on three typical FGVC datasets, i.e., CUB-200-2011, Stanford Cars, and FGVC-Aircraft. We hope the idea of moderate hard example modulation will inspire future research work toward more effective fine-grained visual recognition.
更多查看译文
关键词
Computer vision,fine-grained classification,loss modulation,model generalization
AI 理解论文
溯源树
样例
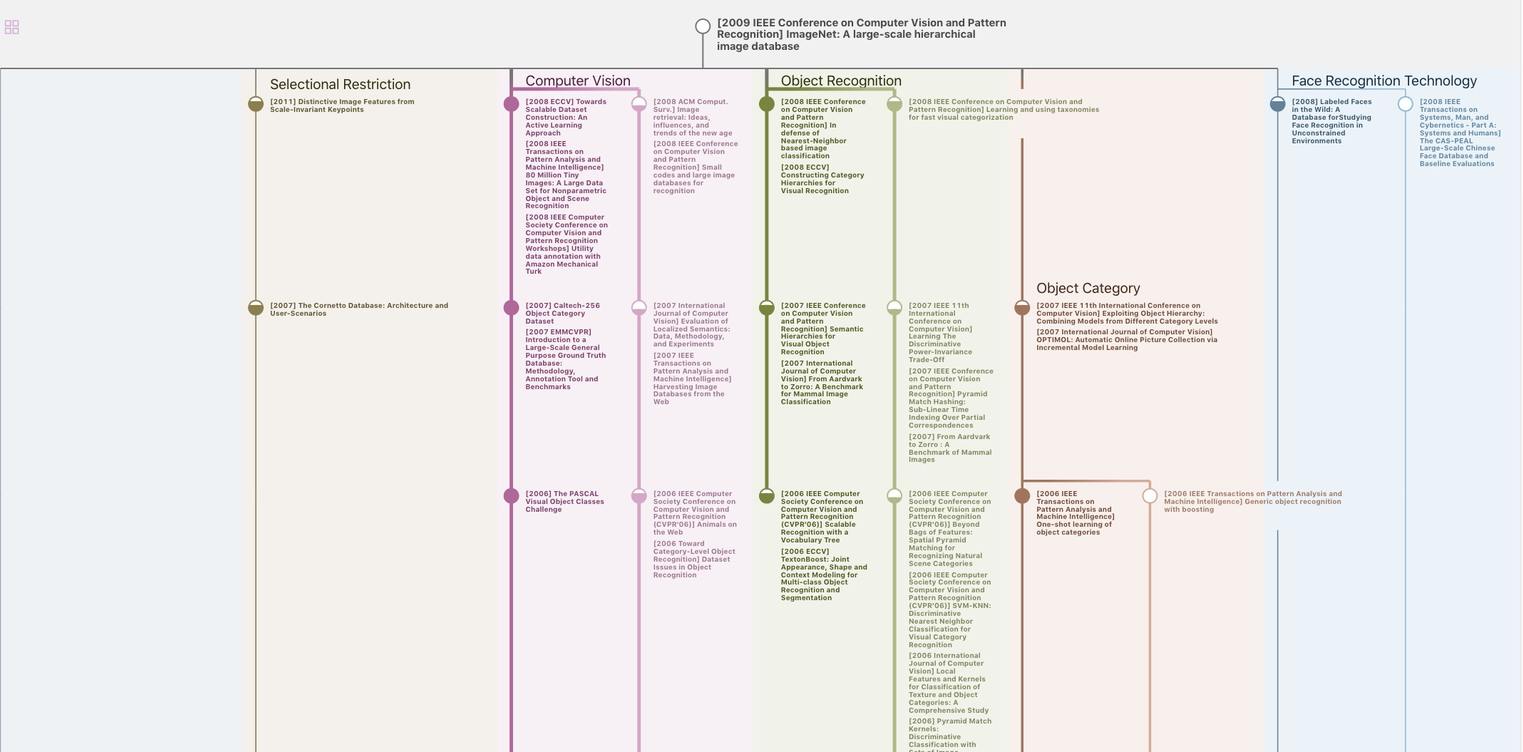
生成溯源树,研究论文发展脉络
Chat Paper
正在生成论文摘要