A novel rumor detection with multi-objective loss functions in online social networks
Expert Systems with Applications(2023)
摘要
COVID-19 quickly swept across the world, causing the consequent infodemic represented by the rumors that have brought immeasurable losses to the world. It is imminent to achieve rumor detection as quickly and accurately as possible. However, the existing methods either focus on the accuracy of rumor detection or set a fixed threshold to attain early detection that unfortunately cannot adapt to various rumors. In this paper, we focus on textual rumors in online social networks and propose a novel rumor detection method. We treat the detection time, accuracy and stability as the three training objectives, and continuously adjust and optimize this objective instead of using a fixed value during the entire training process, thereby enhancing its adaptability and universality. To improve the efficiency, we design a sliding interval to intercept the required data rather than using the entire sequence data. To solve the problem of hyperparameter selection brought by integration of multiple optimization objectives, a convex optimization method is utilized to avoid the huge computational cost of enumerations. Extensive experimental results demonstrate the effectiveness of the proposed method. Compared with state-of-art counterparts in three different datasets, the recognition accuracy is increased by an average of 7%, and the stability is improved by an average of 50%.
更多查看译文
关键词
Online social networks,Rumor detection,Neural network,Multi-objective optimization,Sliding interval
AI 理解论文
溯源树
样例
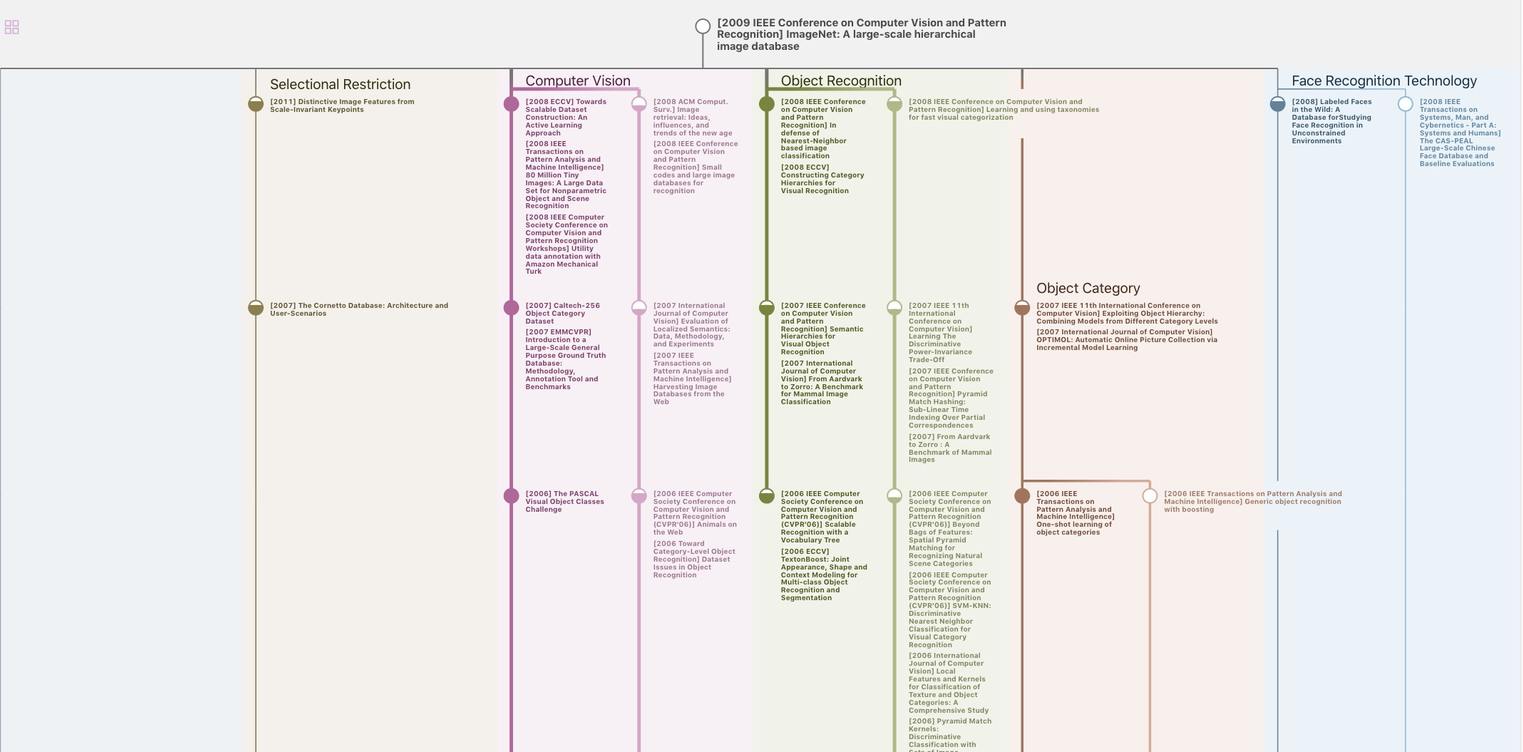
生成溯源树,研究论文发展脉络
Chat Paper
正在生成论文摘要