Considering various aspects of models’ quality in the ML pipeline - application in the logistics sector
2022 17th Conference on Computer Science and Intelligence Systems (FedCSIS)(2022)
摘要
The industrial machine learning applications today involve developing and deploying MLOps pipelines to ensure the versatile quality of forecasting models over an extended period, simultaneously assuring the model’s accuracy, stability, short training time, and resilience. In this study, we present the ML pipeline conforming to all the abovementioned aspects of models’ quality formulated as a constrained multi-objective optimization problem. We also provide the reference implementation on state-of-the-art methods for data preprocessing, feature extraction, dimensionality reduction, feature and instance selection, model fitting, and ensemble blending. The experimental study on the real data set from the logistics industry confirmed the qualities of the proposed approach, as the successful participation in an international data competition did.
更多查看译文
关键词
XGBoost,Dimensionality reduction,Ensemble blending,Feature selection,Feature extraction,MOO,MCDA,Logistics
AI 理解论文
溯源树
样例
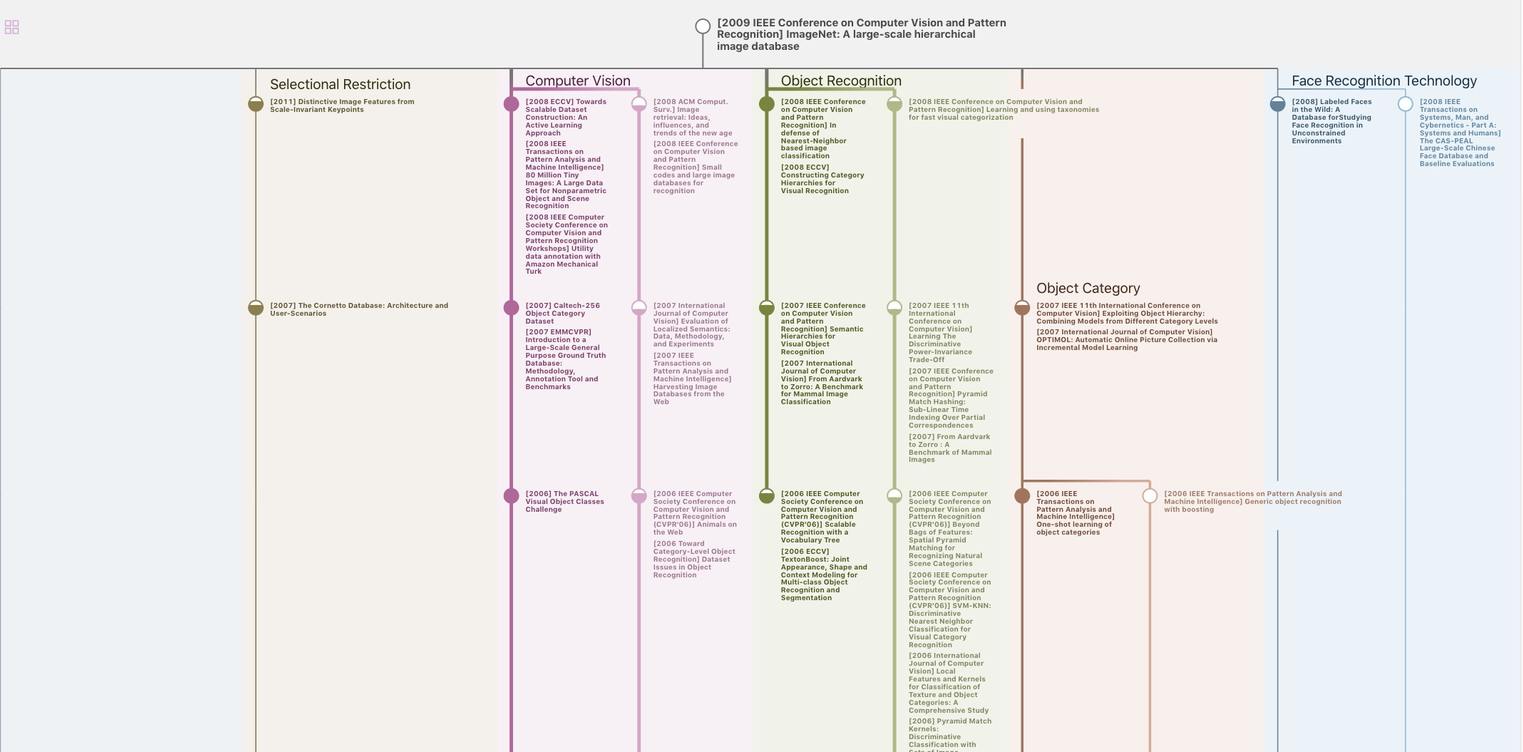
生成溯源树,研究论文发展脉络
Chat Paper
正在生成论文摘要