Tight Bound for Sum of Heterogeneous Random Variables: Application to Chance Constrained Programming
arxiv(2022)
摘要
We study a tight Bennett-type concentration inequality for sums of heterogeneous and independent variables, defined as a one-dimensional minimization. We show that this refinement, which outperforms the standard known bounds, remains computationally tractable: we develop a polynomial-time algorithm to compute confidence bounds, proved to terminate with an epsilon-solution. From the proposed inequality, we deduce tight distributionally robust bounds to Chance-Constrained Programming problems. To illustrate the efficiency of our approach, we consider two use cases. First, we study the chance-constrained binary knapsack problem and highlight the efficiency of our cutting-plane approach by obtaining stronger solution than classical inequalities (such as Chebyshev-Cantelli or Hoeffding). Second, we deal with the Support Vector Machine problem, where the convex conservative approximation we obtain improves the robustness of the separation hyperplane, while staying computationally tractable.
更多查看译文
关键词
chance constrained programming,heterogeneous random variables,bound
AI 理解论文
溯源树
样例
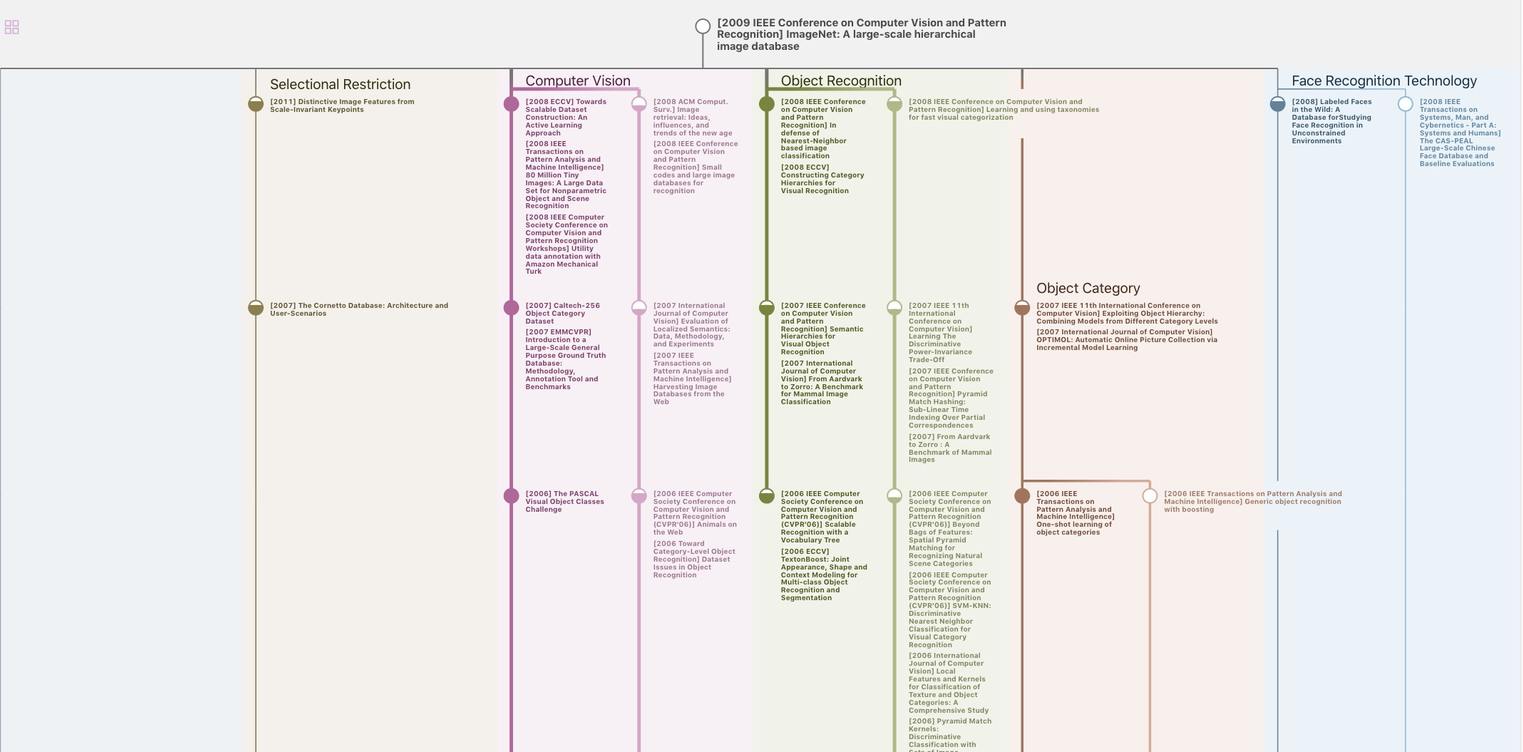
生成溯源树,研究论文发展脉络
Chat Paper
正在生成论文摘要