How to Validate Machine-Learned Interatomic Potentials
Journal of chemical physics online/The Journal of chemical physics/Journal of chemical physics(2023)
摘要
Machine learning (ML) approaches enable large-scale atomistic simulations with near-quantum-mechanical accuracy. With the growing availability of these methods, there arises a need for careful validation, particularly for physically agnostic models—that is, for potentials that extract the nature of atomic interactions from reference data. Here, we review the basic principles behind ML potentials and their validation for atomic-scale material modeling. We discuss the best practice in defining error metrics based on numerical performance, as well as physically guided validation. We give specific recommendations that we hope will be useful for the wider community, including those researchers who intend to use ML potentials for materials “off the shelf.”
更多查看译文
关键词
Materials Informatics
AI 理解论文
溯源树
样例
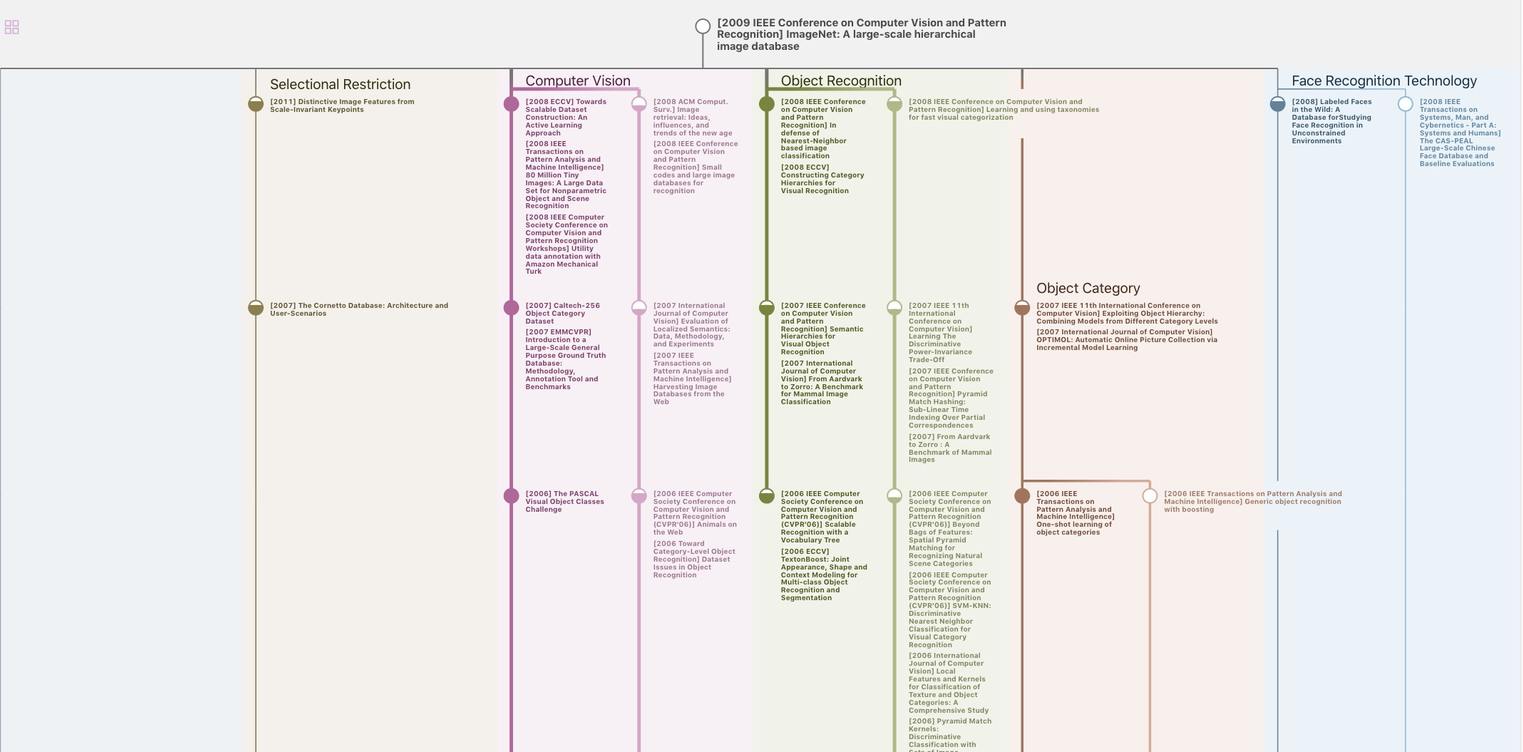
生成溯源树,研究论文发展脉络
Chat Paper
正在生成论文摘要