A symmetric prior knowledge based deep learning model for intracerebral hemorrhage lesion segmentation
FRONTIERS IN PHYSIOLOGY(2022)
摘要
Background: Accurate localization and classification of intracerebral hemorrhage (ICH) lesions are of great significance for the treatment and prognosis of patients with ICH. The purpose of this study is to develop a symmetric prior knowledge based deep learning model to segment ICH lesions in computed tomography (CT). Methods: A novel symmetric Transformer network (Sym-TransNet) is designed to segment ICH lesions in CT images. A cohort of 1,157 patients diagnosed with ICH is established to train (n = 857), validate (n = 100), and test (n = 200) the Sym-TransNet. A healthy cohort of 200 subjects is added, establishing a test set with balanced positive and negative cases (n = 400), to further evaluate the accuracy, sensitivity, and specificity of the diagnosis of ICH. The segmentation results are obtained after data pre-processing and Sym-TransNet. The DICE coefficient is used to evaluate the similarity between the segmentation results and the segmentation gold standard. Furthermore, some recent deep learning methods are reproduced to compare with Sym-TransNet, and statistical analysis is performed to prove the statistical significance of the proposed method. Ablation experiments are conducted to prove that each component in Sym-TransNet could effectively improve the DICE coefficient of ICH lesions. Results: For the segmentation of ICH lesions, the DICE coefficient of Sym-TransNet is 0.716 +/- 0.031 in the test set which contains 200 CT images of ICH. The DICE coefficients of five subtypes of ICH, including intraparenchymal hemorrhage (IPH), intraventricular hemorrhage (IVH), extradural hemorrhage (EDH), subdural hemorrhage (SDH), and subarachnoid hemorrhage (SAH), are 0.784 +/- 0.039, 0.680 +/- 0.049, 0.359 +/- 0.186, 0.534 +/- 0.455, and 0.337 +/- 0.044, respectively. Statistical results show that the proposed Sym-TransNet can significantly improve the DICE coefficient of ICH lesions in most cases. In addition, the accuracy, sensitivity, and specificity of Sym-TransNet in the diagnosis of ICH in 400 CT images are 91.25%, 98.50%, and 84.00%, respectively. Conclusion: Compared with recent mainstream deep learning methods, the proposed Sym-TransNet can segment and identify different types of lesions from CT images of ICH patients more effectively. Moreover, the Sym-TransNet can diagnose ICH more stably and efficiently, which has clinical application prospects.
更多查看译文
关键词
intracerebral hemorrhage,lesion segmentation,deep learning,symmetric knowledge,transformer
AI 理解论文
溯源树
样例
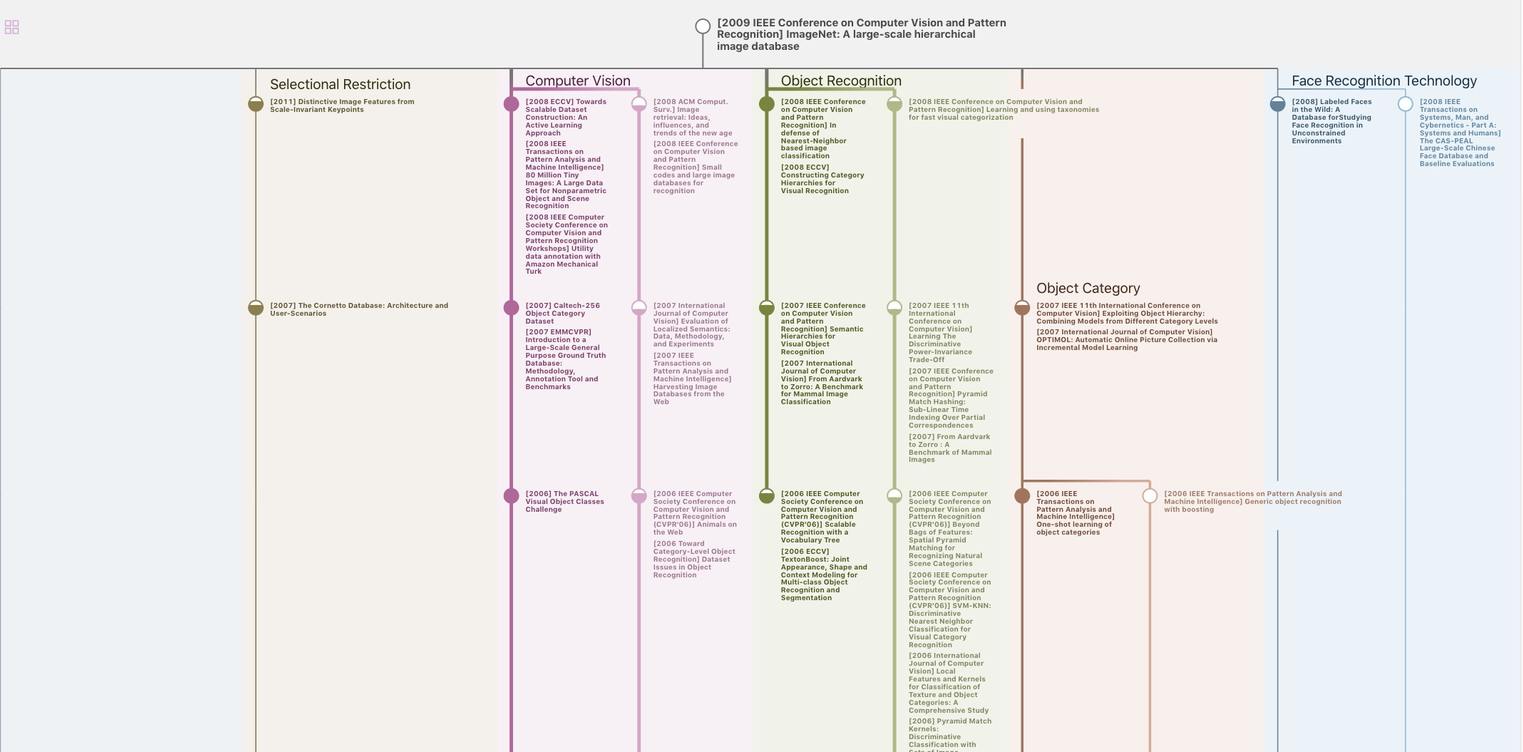
生成溯源树,研究论文发展脉络
Chat Paper
正在生成论文摘要