A 28nm 8-bit Floating-Point Tensor Core based CNN Training Processor with Dynamic Activation/Weight Sparsification
ESSCIRC 2022- IEEE 48th European Solid State Circuits Conference (ESSCIRC)(2022)
摘要
We present an 8-bit floating-point (FP8) training processor which implements (1) highly parallel tensor cores (fused multiply-add trees) that maintain high utilization throughout forward propagation (FP), backward propagation (BP), and weight update (WU) phases of the training process, (2) hardware-efficient channel gating for dynamic output activation sparsity, (3) dynamic weight sparsity based on group Lasso, and (4) gradient skipping based on FP prediction error. We develop a custom ISA to flexibly support different CNN topologies and training parameters. The 28nm prototype chip demonstrates large improvements in FLOPs reduction (7.3 ×), energy efficiency (16.4 TFLOPS/W), and overall training latency speedup (4.7×), for both supervised and self-supervised training tasks.
更多查看译文
关键词
Convolutional neural networks,deep neural network training,structured sparsity,hardware accelerator
AI 理解论文
溯源树
样例
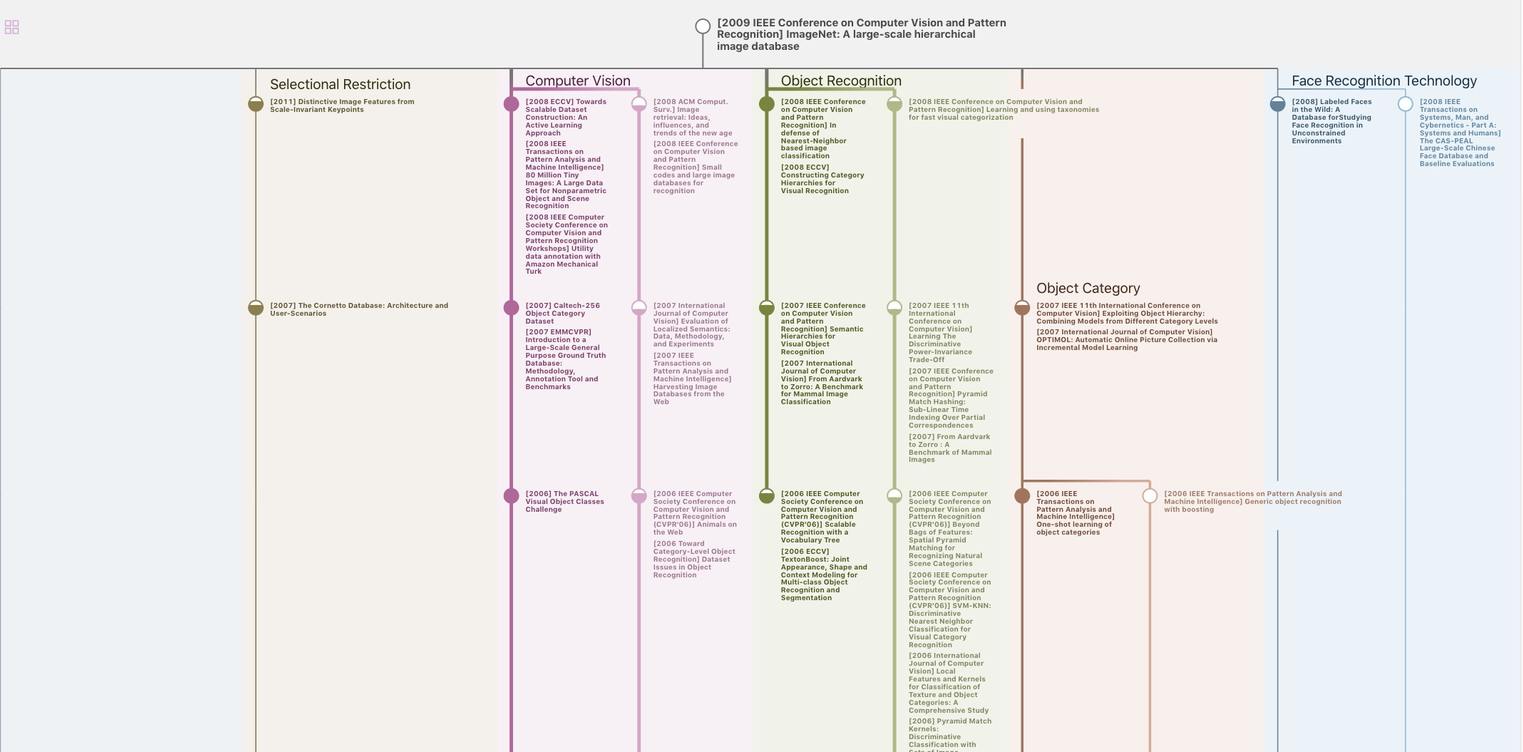
生成溯源树,研究论文发展脉络
Chat Paper
正在生成论文摘要