Adaptive Deep Feature Fusion for Continuous Authentication With Data Augmentation
user-5ed732bc4c775e09d87b4c18(2023)
摘要
Mobile devices are becoming increasingly popular and are playing significant roles in our daily lives. Insufficient security and weak protection mechanisms, however, cause serious privacy leakage of the unattended devices. To fully protect mobile device privacy, we propose ADFFDA, a novel mobile continuous authentication system using an Adaptive Deep Feature Fusion scheme for effective feature representation, and a transformer-based GAN for Data Augmentation, by leveraging smartphone built-in sensors of the accelerometer, gyroscope and magnetometer. Given the normalized sensor data, ADFFDA utilizes the transformer-based GAN consisting of a transformer-based generator and a CNN-based discriminator to augment the training data for CNN training. With the augmented data and the especially-designed CNN based on the ghost module and ghost bottleneck, ADFFDA extracts deep features from the three sensors by the trained CNN, and exploits an adaptive-weighted concatenation method to adaptively fuse the CNN-extracted features. Based on the fused features, ADFFDA authenticates users by using the one-class SVM (OC-SVM) classifier. We evaluate the authentication performance of ADFFDA in terms of the efficiency of the transformer-based GAN, GAN-based data augmentation, CNN architecture, adaptive-weighted feature fusion, OC-SVM classifier, and security analysis. The experimental results show that ADFFDA obtains the best authentication performance w.r.t representative approaches, by achieving a mean equal error rate of 0.01%.
更多查看译文
关键词
continuous authentication,adaptive deep feature fusion,data augmentation
AI 理解论文
溯源树
样例
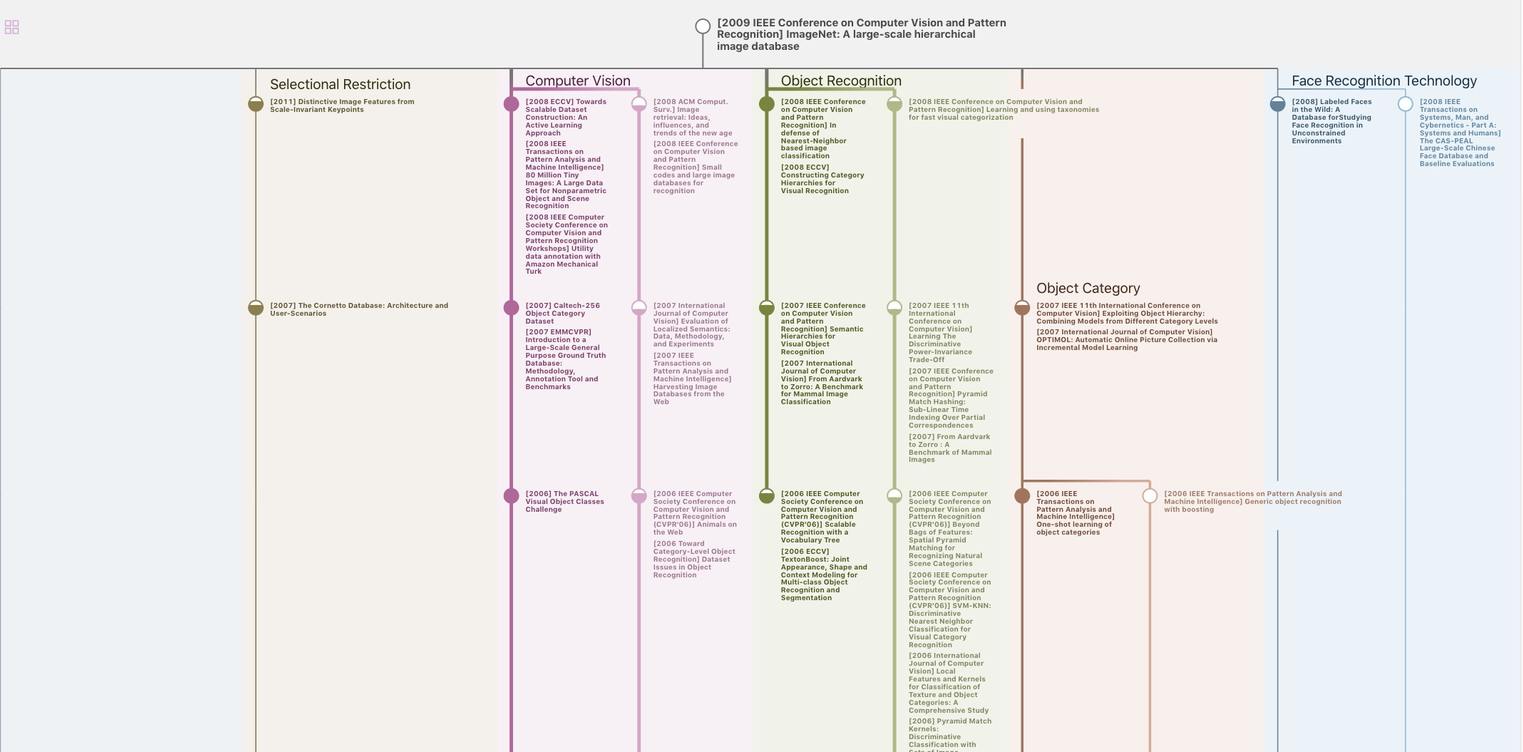
生成溯源树,研究论文发展脉络
Chat Paper
正在生成论文摘要