An Online Reinforcement Learning Approach for Solving the Dynamic Flexible Job-Shop Scheduling Problem for Multiple Products and Constraints
2021 International Conference on Computational Science and Computational Intelligence (CSCI)(2021)
摘要
In the manufacturing industries, the most challenging problems are mostly related to time efficiency and customer satisfaction. This is mainly translated to how efficient is the frequent task of scheduling jobs to alternative routes on a number of machines. Although scheduling has been studied for decades, there is a shortage to a generalized approach for the production scheduling that can adapt to changes occurring continuously at any production environment. This research work addresses the dynamic production scheduling problem and the optimization techniques that could be applied to the production schedule to increase its efficiency. An algorithm is proposed to apply the Q-learning optimization technique on a dynamic flexible job-shop scheduling problem of a real case study of a pharmaceutical factory with 18 machines and 22 products. Proposed algorithm is shown to be able to achieve an efficient schedule with short make-span in minimal time duration and without requiring any learning process from previous schedules, thus increasing the factory's overall efficiency. In addition, the proposed algorithm operates online as any change occurring in the production environment is signaled automatically to it where it responds be regenerating the most optimal updated production schedule.
更多查看译文
关键词
Artificial Intelligence,Job-Shop,Optimization,Production Scheduling,Reinforcement Learning
AI 理解论文
溯源树
样例
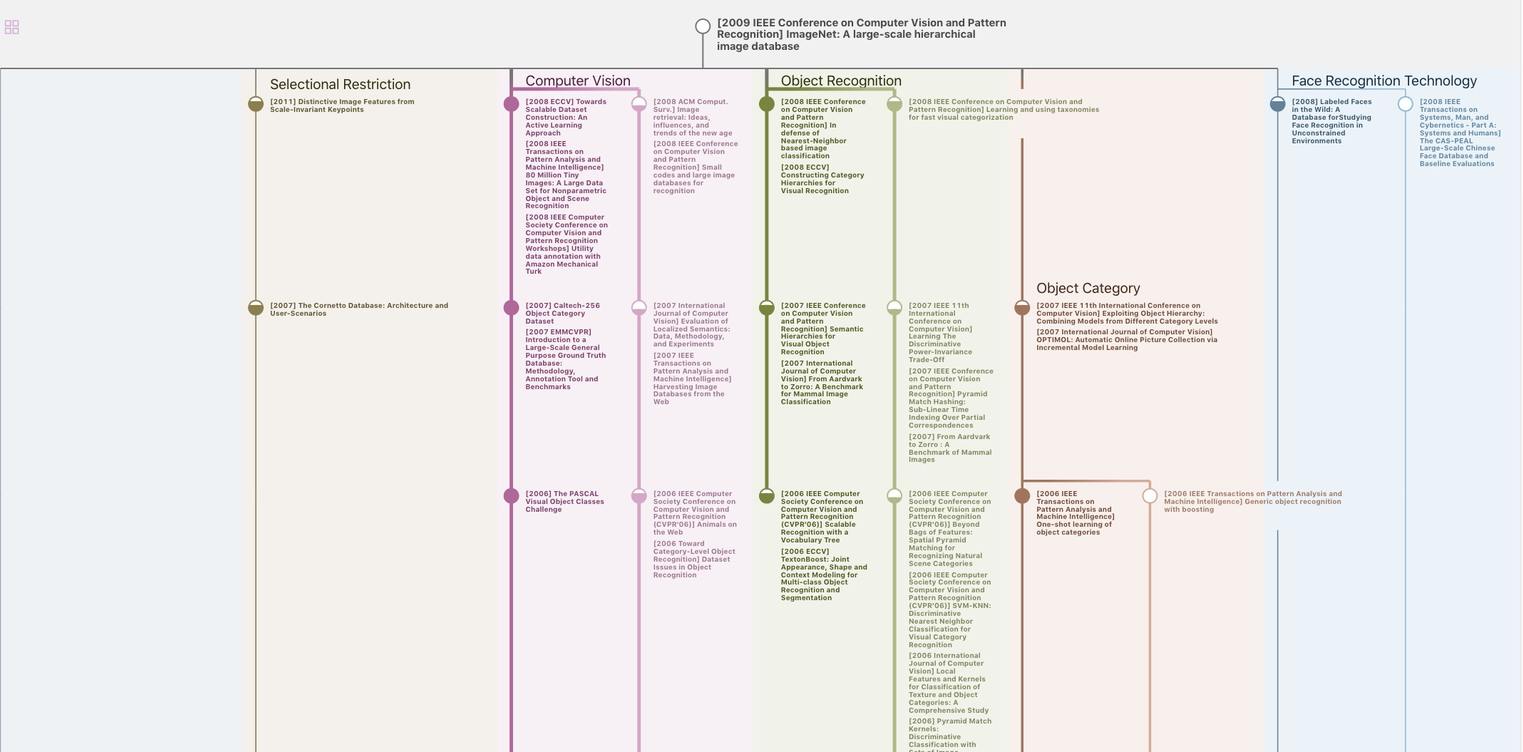
生成溯源树,研究论文发展脉络
Chat Paper
正在生成论文摘要