Sylvester Equation Induced Collaborative Representation Learning for Recommendation
user-5ed732bc4c775e09d87b4c18(2023)
摘要
For an actual recommendation system, it generally involves a variety of heterogeneous interactive relationships, such as the typical user-user (U2U), item-item (I2I), and user-item (U2I) interaction relationships. With the application of graph neural networks (GNNs) in embedding various interactive relations, recommendation technology has made gratifying progress in recent years, which benefits lot from its powerful ability in relation modeling. However, most of the existing GNN-based methods fail to collaboratively explore the above heterogeneous multiple interactive relationships, including the internal correlations among multiple relationships and the intrinsic association behind different relationships. As a consequence, the user's personalized preference for the items to be recommended will not be well captured. In this paper, we propose a
S
ylvester equation induced
C
ollaborative
R
epresentation
L
earning framework (S-CRL) for recommendation system by utilizing the heterogeneous multiple interactive relationships. In particular, we ingeniously define a novel Sylvester equation to associate tactfully the multiple heterogeneous relations together. From the perspective of rating propagation, such Sylvester equation is shown theoretically to be the optimal solution of a local structure sensitive rating propagation function. Additionally, to seek more expressive embeddings about user and item, a layer-wise attention is introduced to aggregate the multi-hop information from U2U and I2I graphs, respectively, so as to promote the aggregation with the corresponding embeddings from the U2I interaction graph. Extensive experiments on three real-world datasets verify that our model achieves more favorable performance over currently representative methods.
更多查看译文
关键词
Recommendation system,graph neural networks,collaborative representation learning,sylvester equation
AI 理解论文
溯源树
样例
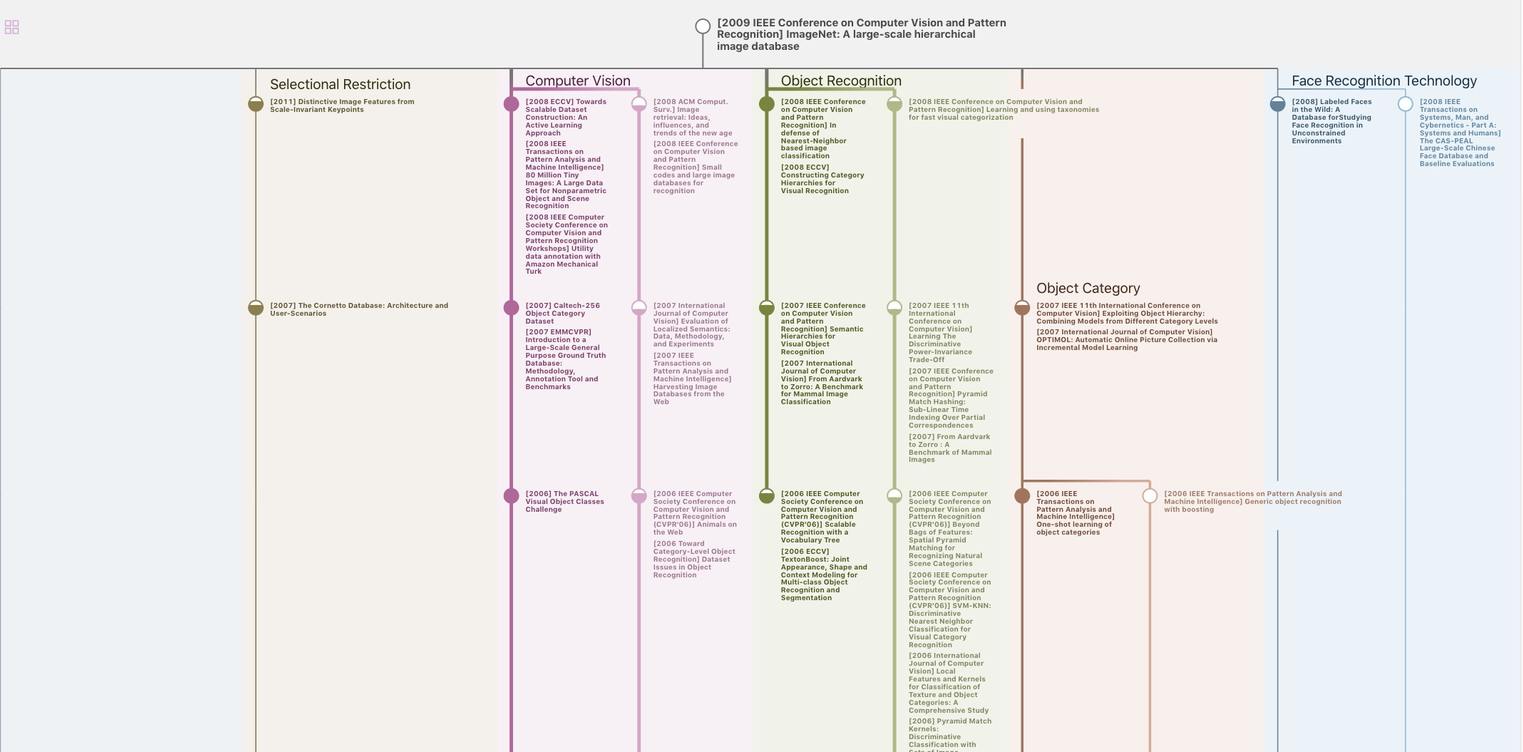
生成溯源树,研究论文发展脉络
Chat Paper
正在生成论文摘要