Worker-Centric Model Allocation for Federated Learning in Mobile Edge Computing
user-5ed732bc4c775e09d87b4c18(2023)
摘要
Federated Learning (FL) is believed as a promising manner of distributed machine learning for 5G and future 6G networks in the context of mobile edge computing (MEC). From the worker's viewpoint, a problem is that no incentive drives them to participate in FL. Thus, different from the conventional server-centric model allocation methods, we study the worker-centric strategy in this paper. Because communicating with the FL server and training an FL model locally are energy-hungry, a dilemma for each worker is how to make the tradeoff between participating in FL training and the volume restriction of its battery. To address this issue, we formulate a WorkerFirst problem. Its NP-hardness is also proved. Next, we devise a DDQN algorithm and a DQL algorithm to strive for near-optimal decisions for each worker. These two algorithms are proposed by leveraging the deep reinforcement learning framework, while considering the energy consumption, training timespan, and the communication overheads of workers, simultaneously. The benefit of adopting such a DRL-based decision-making framework is that workers can execute the algorithms locally and dynamically adapt to the varying MEC environment. The evaluation results show that the proposed DDQN and DQL algorithms can learn good policies without knowing any prior knowledge of network conditions.
更多查看译文
关键词
Federated learning,model allocation,mobile edge computing
AI 理解论文
溯源树
样例
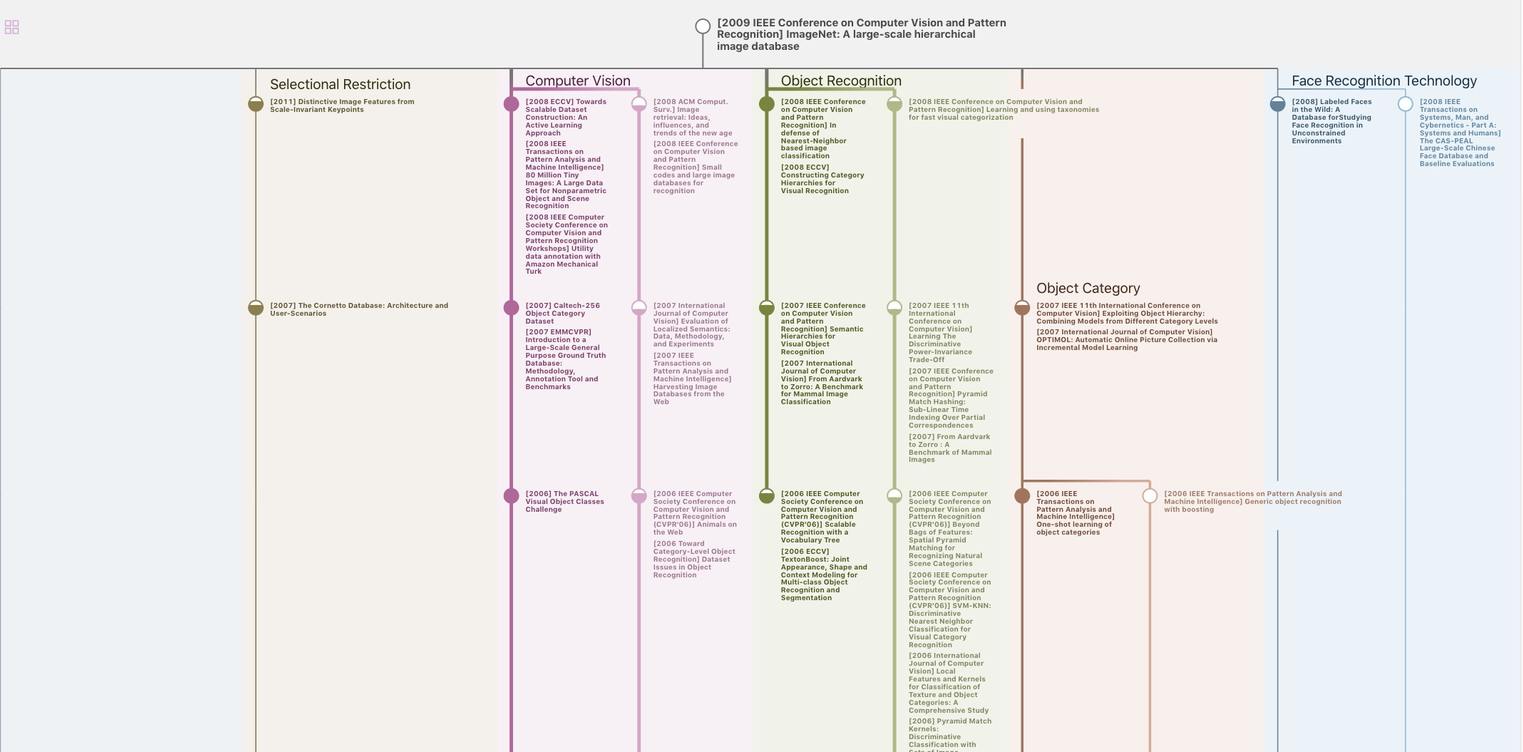
生成溯源树,研究论文发展脉络
Chat Paper
正在生成论文摘要