An evolutionary stacked generalization model based on deep learning and improved grasshopper optimization algorithm for predicting the remaining useful life of PEMFC
APPLIED ENERGY(2023)
摘要
Accurate prediction of the future degradation trend (FDT) and remaining useful life (RUL) of proton exchange membrane fuel cell (PEMFC) is crucial in the prognosis and health management (PHM) of PEMFC. Therefore, this study develops a stacked generalization model (SGM) to predict the FDT and RUL of the PEMFC. Firstly, maximum information coefficient (MIC) and Akaike information criterion (AIC) are used to select input variables to obtain key information and reduce the difficulty of model training. Then use improved grasshopper optimization algorithm (GOA) to optimize the hyperparameters of the SGM to further improve the prediction performance of the model. Finally, according to the dataset after feature selection, improved GOA (IGOA) optimized SGM (M/AIC-IGOA-SGM) is established to predict FDT and RUL. Among them, IGOA is obtained by introducing Chebyshev chaotic mapping initialization, chaotic decreasing factor, and a spiral position update mechanism to GOA. The base models of SGM are support vector regression (SVR) and gated recurrent unit (GRU), and the meta-model is regularized extreme learning machine (RELM). In the comparative experiments under two different current conditions, the superiority of the proposed model is verified, and the effectiveness of M/AIC, IGOA, and SGM is discussed.
更多查看译文
关键词
Proton exchange membrane fuel cell,Future degradation trend,Remaining useful life,Gated recurrent unit,Improved grasshopper optimization algorithm,Stacked generalization
AI 理解论文
溯源树
样例
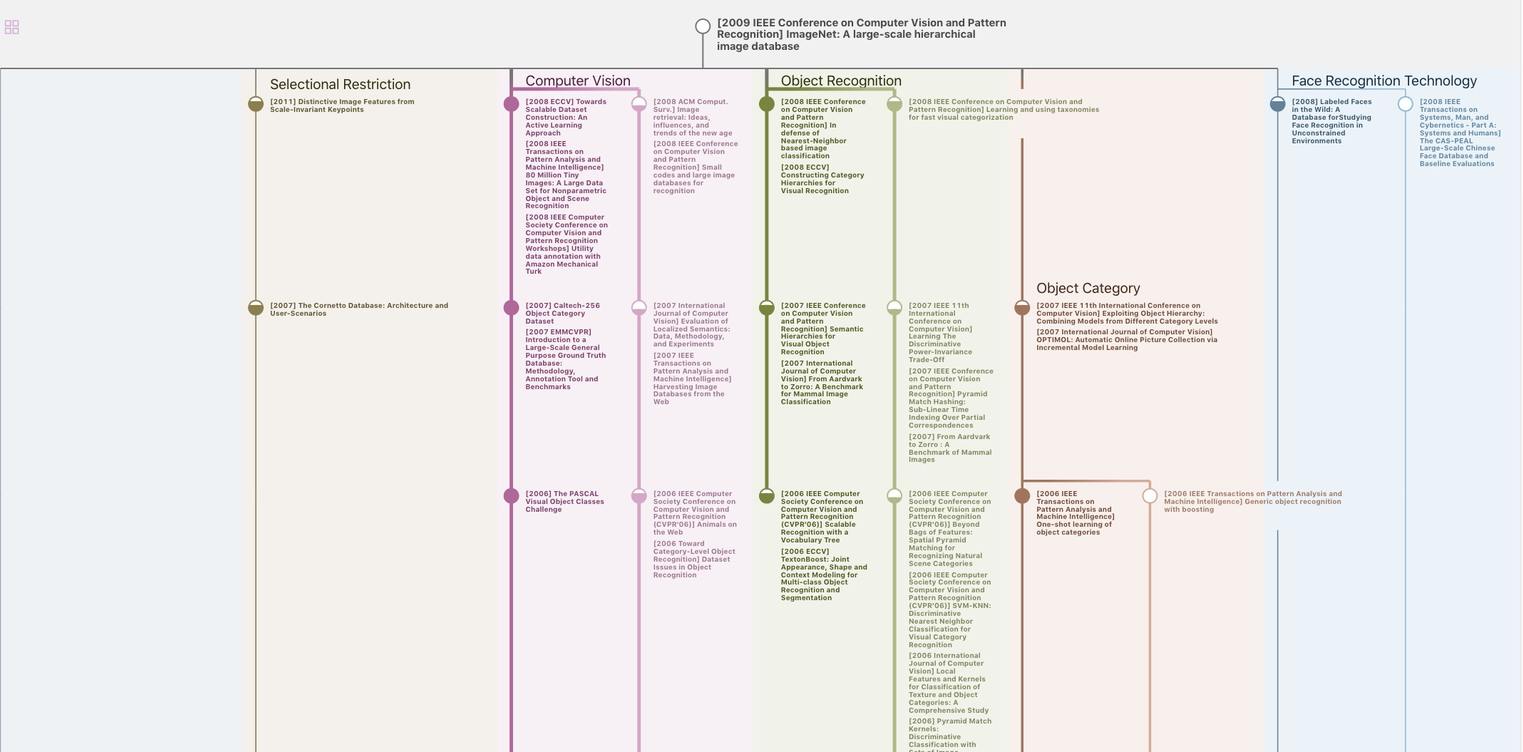
生成溯源树,研究论文发展脉络
Chat Paper
正在生成论文摘要