Which Exogenous Driver is Informative in Forecasting European Carbon Volatility: Bond, Commodity, Stock or Uncertainty?
Energy economics(2023)
Abstract
This study relies on 45 exogenous drivers to improve the accuracy in forecasting EUA volatility. Several popular linear and nonlinear predictive regressions, including individual factor analysis, the combination forecast method, the diffusion index model and the supervised learning method, are used to generate volatility forecasts at the monthly frequency. Our empirical results reveal that the diffusion index model and combination forecast method can hardly drive the EUA volatility in a data-rich world owing to worse forecasting performance of individual factors; however, the supervised learning method can successfully predict the EUA volatility. Additionally, the WilderHill new energy global innovation index, Euro corporate bond return spread, GSCI gold index and Euro Area government bond yield spread can extremely drive EUA volatility in terms of individual factor analysis, frequency of variable selection and factor importance. Our findings provide crucial implications to market participants and emission companies, who should pay more attention to the price movement of European bond market, gold and clean energy.
MoreTranslated text
Key words
European carbon market,Volatility forecasting,Exogenous driver,Supervised learning method
AI Read Science
Must-Reading Tree
Example
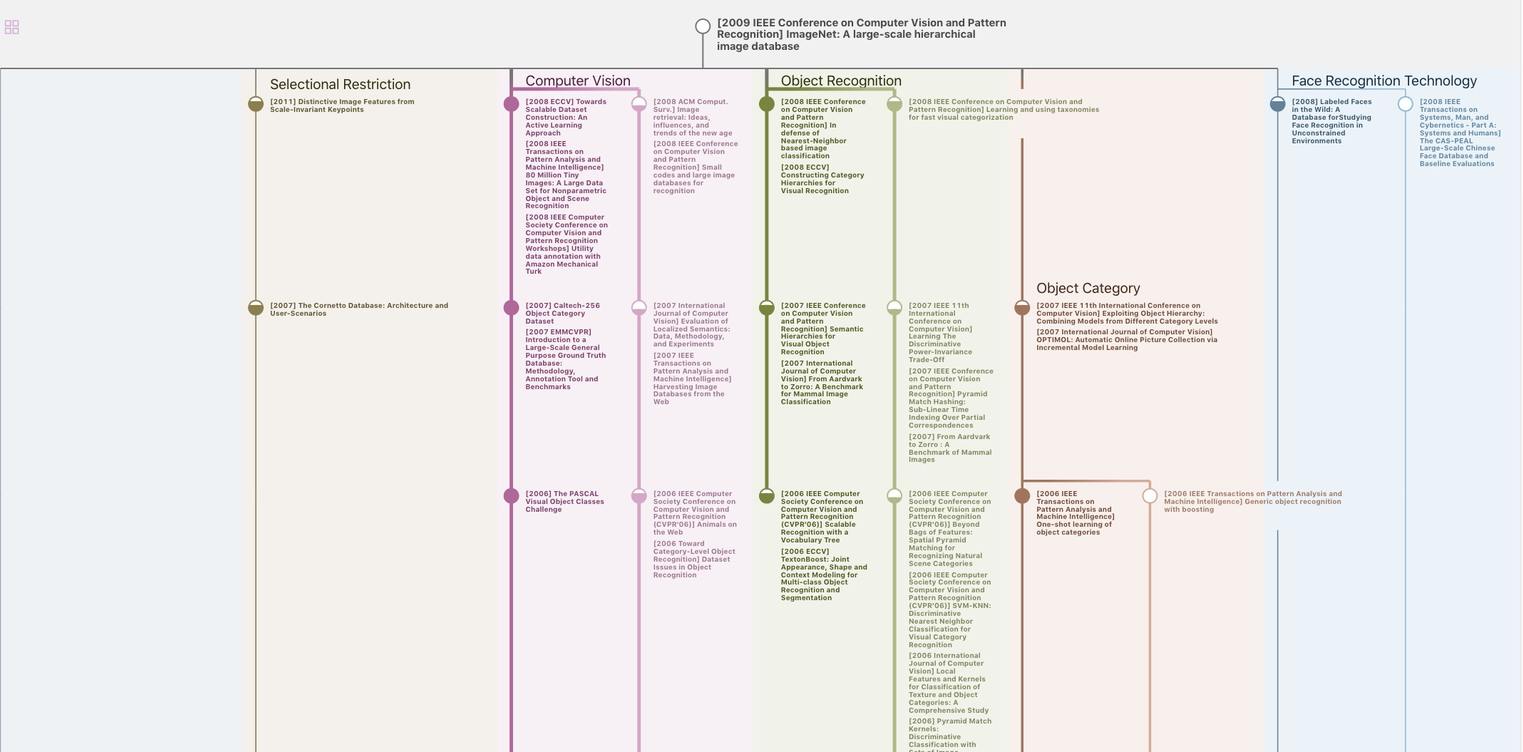
Generate MRT to find the research sequence of this paper
Chat Paper
Summary is being generated by the instructions you defined