Approach to a Lower Body Gait Generation Model Using a Deep Convolutional Generative Adversarial Network
Proceedings of the International Conference on Ubiquitous Computing & Ambient Intelligence (UCAmI 2022)(2022)
摘要
Research over gait analysis has become more relevant in the last years, especially as a tool to detect early frailty signs. However, data gathering is often difficult and requires lots of resources. Synthetic data generation is a great complementary tool for data gathering that enables the augmentation of existing datasets. Despite not being a new concept, it has gained popularity in the last years thanks to Generative Adversarial Networks (GANs), a neural network architecture capable of creating data indistinguishable from the original one. In this article deep-convolutional GANs has been used to artificially expand a gait dataset containing data of the lower part of the body. The synthetic data has been studied through three approaches: looking animations of the points and comparing them to the originals; applying principal component analysis algorithm to both datasets to visually assess how each of them is distributed; and by extracting different features from both datasets to compare their statistical differences. The evaluation showed promising results, which opens a path for using synthetic data generation in the gait analysis domain.
更多查看译文
关键词
Quantitative gait analysis, Generative adversarial network, Convolutional neural network, Xsens, Kinematics synthetic data, Nonpathological gait cycle
AI 理解论文
溯源树
样例
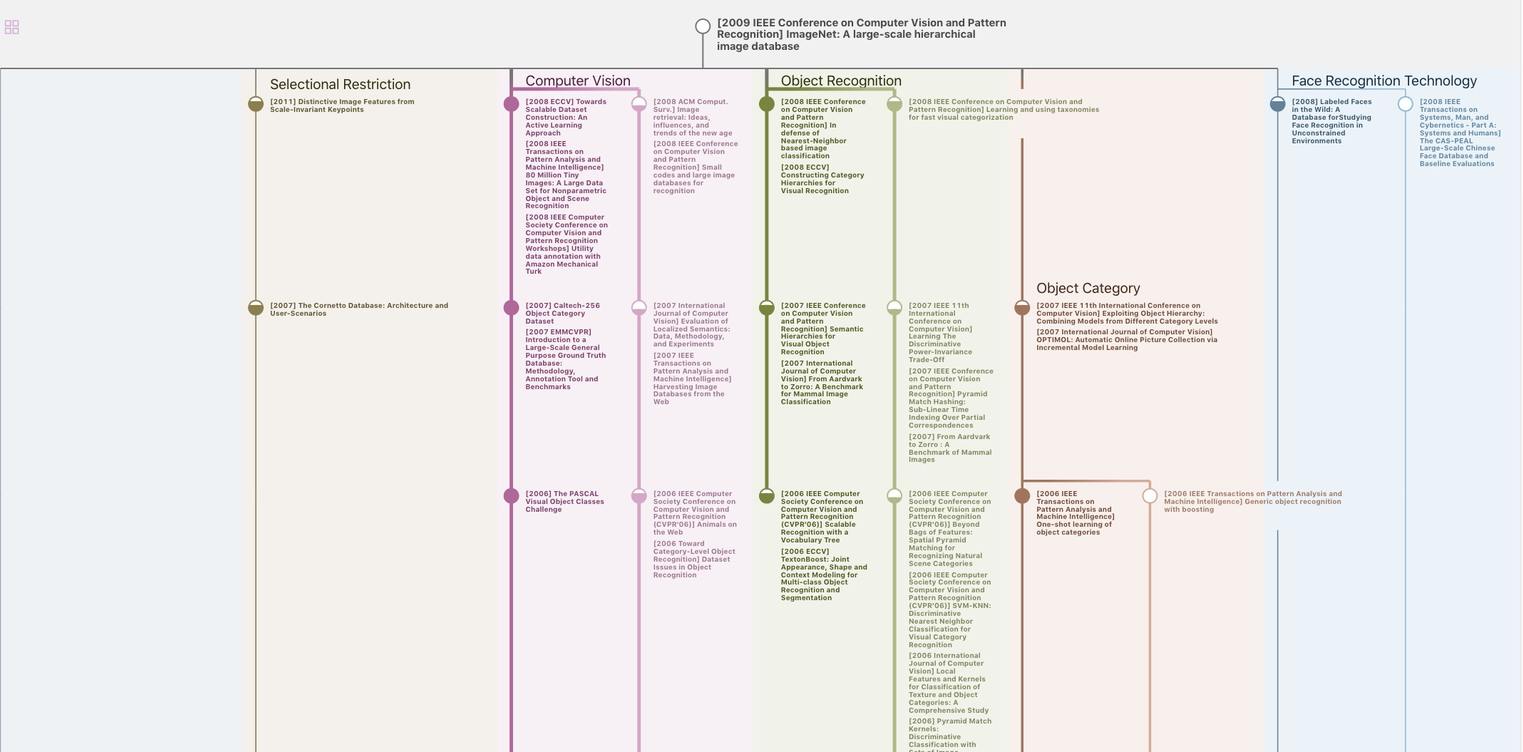
生成溯源树,研究论文发展脉络
Chat Paper
正在生成论文摘要