A network classification method based on density time evolution patterns extracted from network automata
PATTERN RECOGNITION(2024)
摘要
Network modeling has proven to be an efficient tool for many interdisciplinary areas, including social, biological, transportation, and various other complex real-world systems. In addition, cellular automata (CA) are a formalism that has received significant attention in recent decades as a model for investigating patterns in the dynamic spatio-temporal behavior of these systems, based on local rules. Some studies investigate the use of cellular automata to analyze the dynamic behavior of networks and refer to them as network automata (NA). Recently, it has been demonstrated that NA is effective for network classification, as it employs a Time-Evolution Pattern (TEP) for feature extraction. However, the TEPs investigated in previous studies consist of binary values (states) that do not capture the intrinsic details of the analyzed network. Therefore, in this work, we propose alternative sources of information that can be used as descriptors for the classification task, which we refer as Density Time-Evolution Pattern (D-TEP) and State Density Time-Evolution Pattern (SD-TEP). We examine the density of alive neighbors of each node, which is a continuous value, and compute feature vectors based on histograms of TEPs. Our results demonstrate significant improvement over previous studies on five synthetic network datasets, as well as seven real datasets. Our proposed method is not only a promising approach for pattern recognition in networks, but also shows considerable potential for other types of data that can be transformed into network.
更多查看译文
关键词
Complex networks,Cellular automata,Network automata,Pattern recognition
AI 理解论文
溯源树
样例
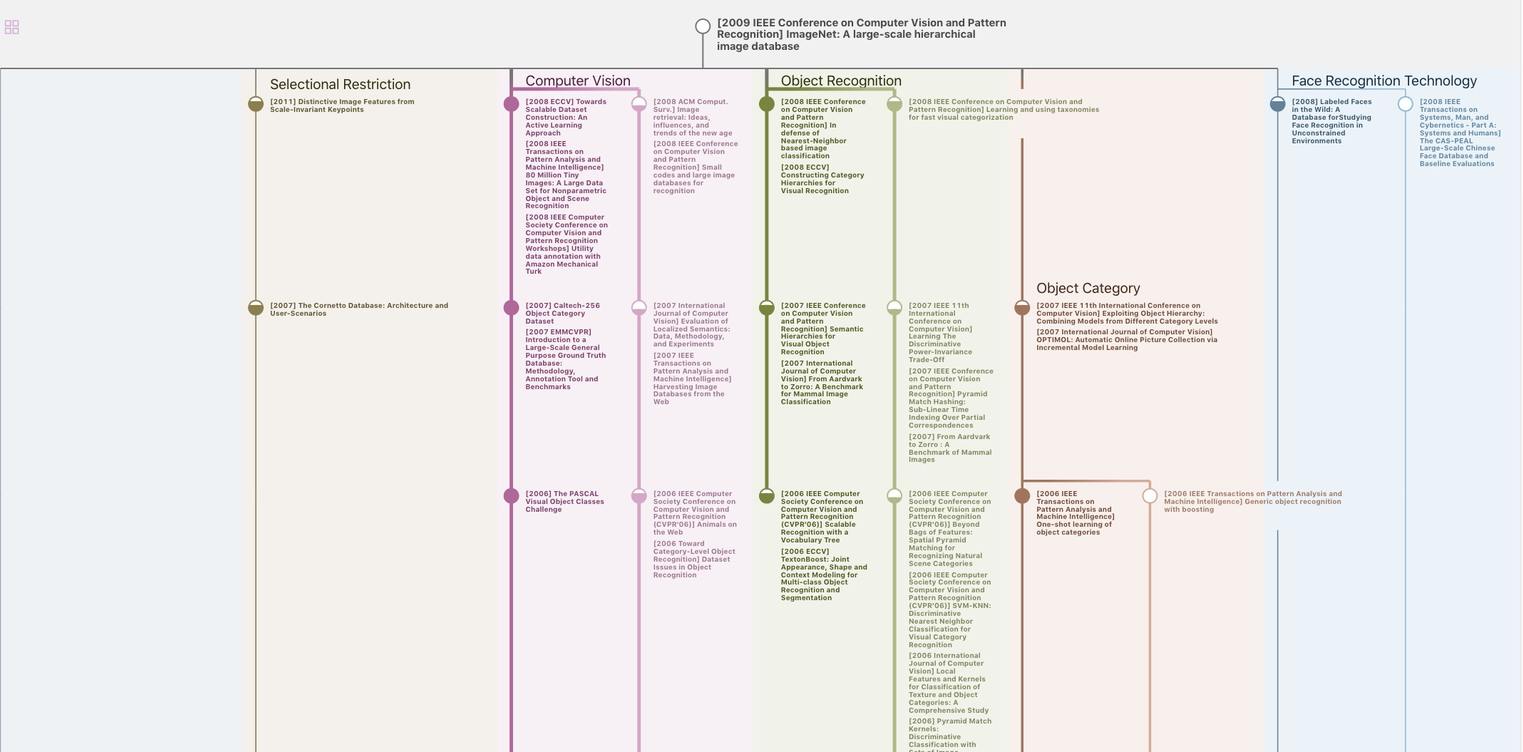
生成溯源树,研究论文发展脉络
Chat Paper
正在生成论文摘要