DWFed: A statistical- heterogeneity-based dynamic weighted model aggregation algorithm for federated learning
FRONTIERS IN NEUROROBOTICS(2022)
摘要
Federated Learning is a distributed machine learning framework that aims to train a global shared model while keeping their data locally, and previous researches have empirically proven the ideal performance of federated learning methods. However, recent researches found the challenge of statistical heterogeneity caused by the non-independent and identically distributed (non-IID), which leads to a significant decline in the performance of federated learning because of the model divergence caused by non-IID data. This statistical heterogeneity is dramatically restricts the application of federated learning and has become one of the critical challenges in federated learning. In this paper, a dynamic weighted model aggregation algorithm based on statistical heterogeneity for federated learning called DWFed is proposed, in which the index of statistical heterogeneity is firstly quantitatively defined through derivation. Then the index is used to calculate the weights of each local model for aggregating federated model, which is to constrain the model divergence caused by non-IID data. Multiple experiments on public benchmark data set reveal the improvements in performance and robustness of the federated models in heterogeneous settings.
更多查看译文
关键词
federated learning,statistical heterogeneity,non-IID data,model aggregation algorithm,earth mover's distance
AI 理解论文
溯源树
样例
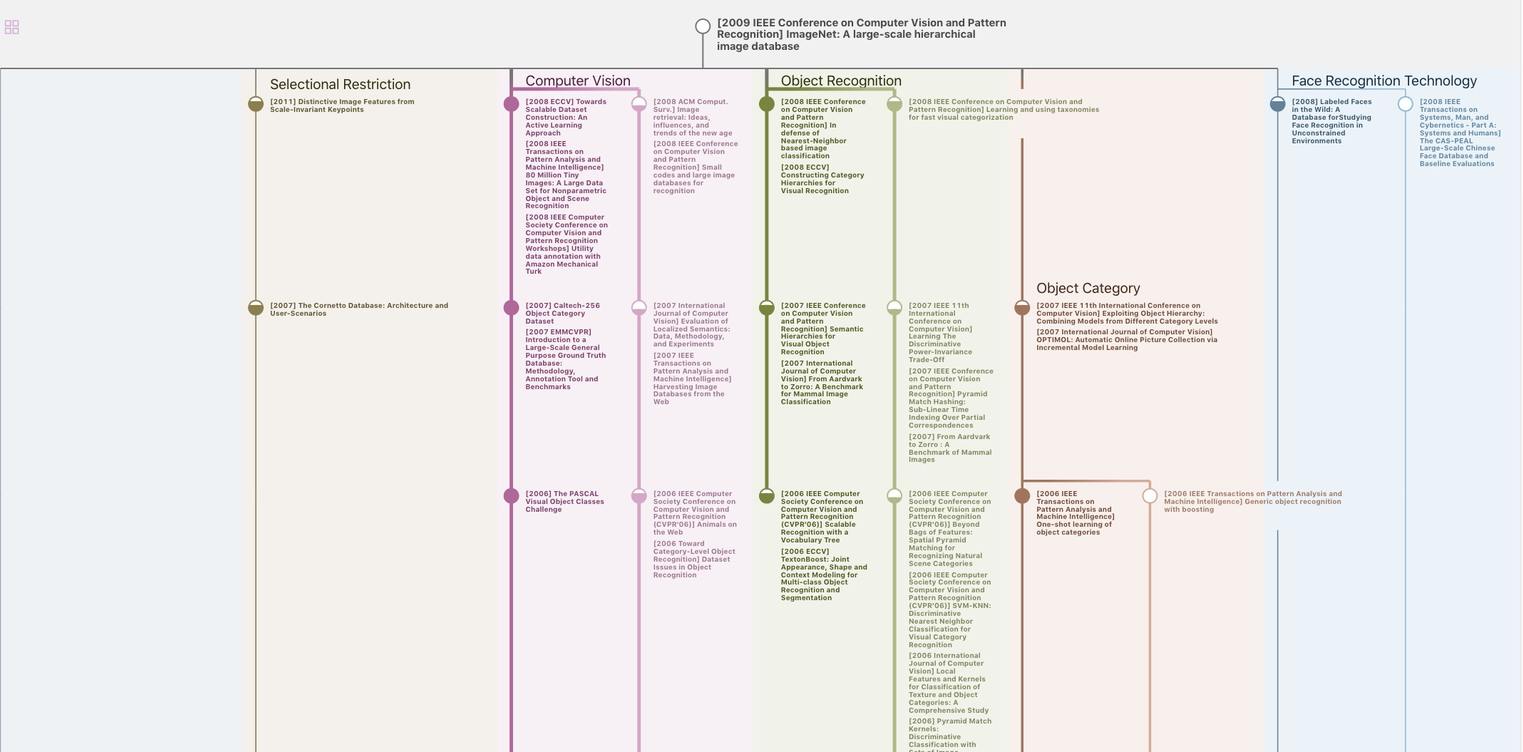
生成溯源树,研究论文发展脉络
Chat Paper
正在生成论文摘要