Large-scale signal and noise correlations configure multi-task coding in human brain networks
biorxiv(2022)
摘要
The brain is a complex system with dynamic network changes. Prior studies in theoretical neuroscience have demonstrated that state-dependent neural correlations can be understood from a neural coding framework. These so-called noise correlations – the trial-to-trial or moment-to-moment co-variability – can be interpreted only if the underlying signal correlation – the similarity of task selectivity between pairs of neural units – is known. While the impact of these correlations on task coding have been widely investigated in local spiking circuits, it remains unclear how this coding framework applies to large-scale brain networks. Here we investigate the relationship between large-scale noise correlations and their underlying signal correlations in a multi-task human fMRI dataset. We found that state-dependent noise correlation changes do not typically align in the same direction as their underlying signal correlation, suggesting that 1) trial-by-trial noise is typically reduced between similarly tuned regions, and 2) stimulus-driven activity does not linearly superimpose atop the network’s underlying background activity. Crucially, we discovered that noise correlations that changed in the opposite direction as their signal correlation (i.e., anti-aligned correlations) improved the information coding of these brain regions. In contrast, noise correlation changes that were aligned with their signal correlation did not. These aligned noise correlations were primarily correlation increases, which have been commonly (yet incorrectly) assumed to increase information communication between brain regions in human neuroimaging studies. These findings illustrate that state-dependent noise correlations contribute to the information coding of functional brain networks, but interpretation of these correlation changes requires knowledge of the underlying signal correlations.
### Competing Interest Statement
The authors have declared no competing interest.
更多查看译文
AI 理解论文
溯源树
样例
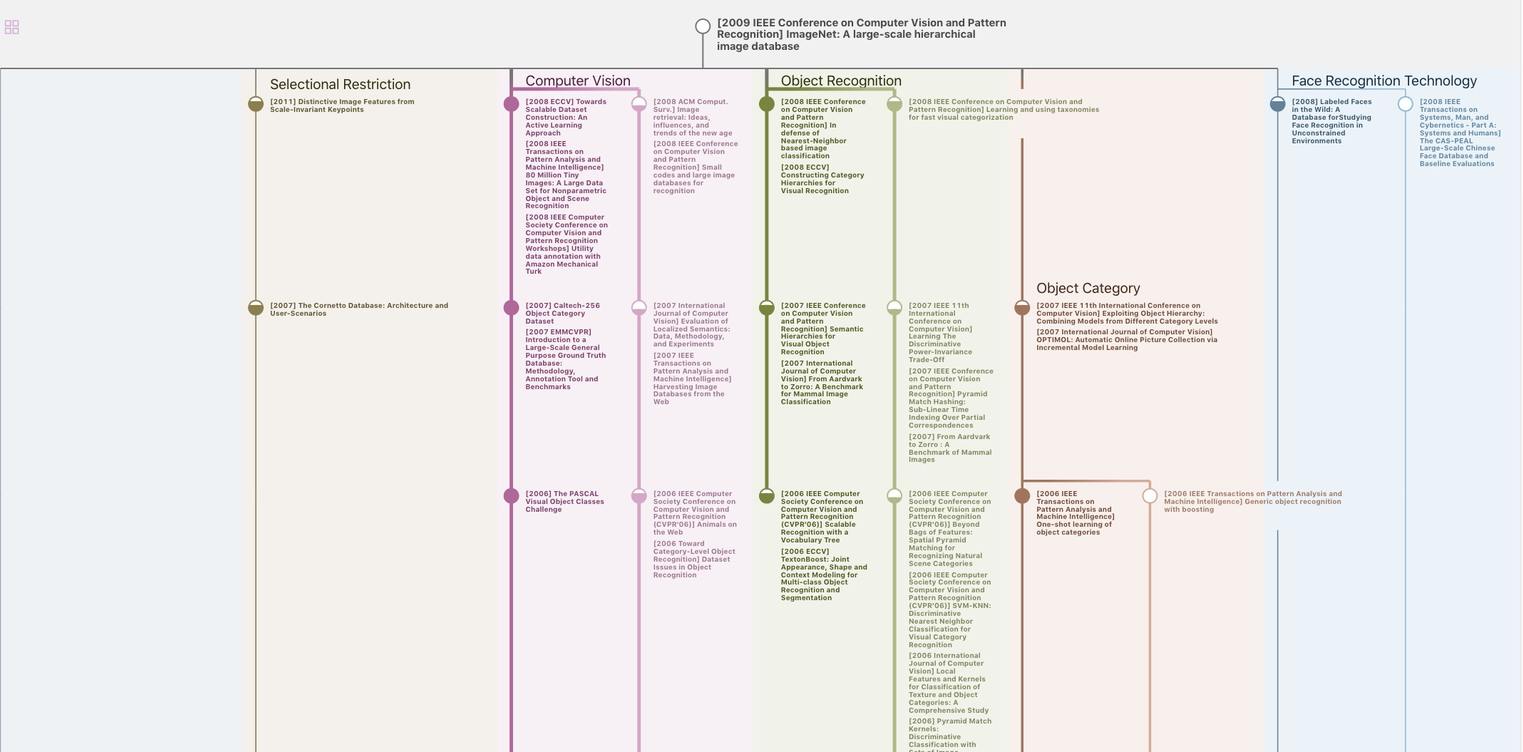
生成溯源树,研究论文发展脉络
Chat Paper
正在生成论文摘要