Reinforcement Learning Based Cross-Layer Congestion Control for Real-Time Communication
2022 IEEE International Symposium on Broadband Multimedia Systems and Broadcasting (BMSB)(2022)
摘要
Congestion control is a crucial part of Real-Time Communication (RTC), because it adjusts the current video bitrate according to the variable network environment, which determines the final Quality of Experience (QoE). Conventional congestion control algorithms only take packet-level delay and throughput into consideration. While in the application of RTC, video frame can provide a lot of reference information, because it is the basic unit of video processing and evaluation. Inspired by this, we propose CLCC, a reinforcement learning based Cross-Layer Congestion Control for WebRTC. CLCC uses not only packet-level information, but also frame-level information to decide the bitrate of the encoder. We evaluate CLCC on both random traces and LTE traces. Results show that CLCC outperforms GCC with improvements in average frame psnr of 0.4-0.6 and decreasing in average frame delay of 17%-23%.
更多查看译文
关键词
Real-time communication,cross-layer,congestion control,reinforcement learning
AI 理解论文
溯源树
样例
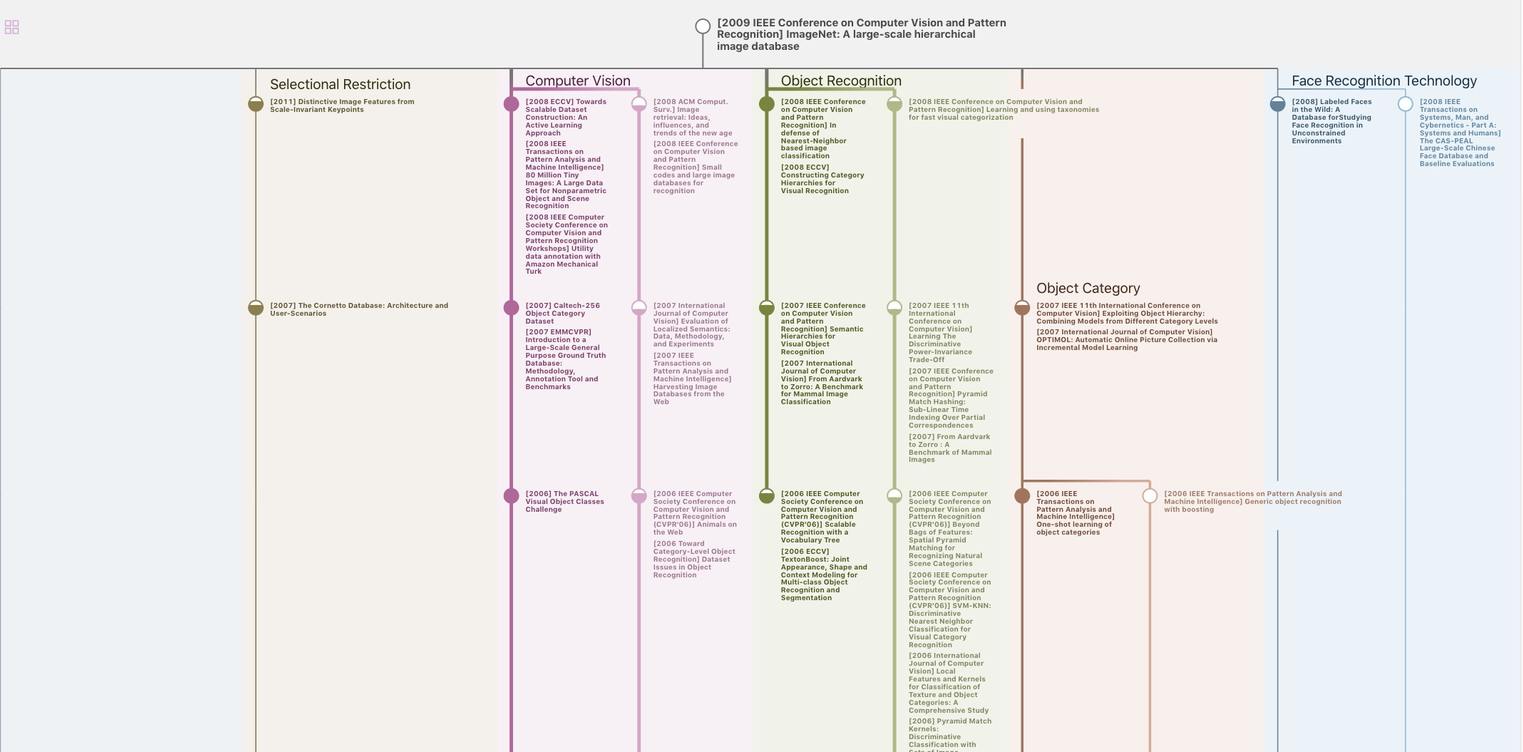
生成溯源树,研究论文发展脉络
Chat Paper
正在生成论文摘要