Joint Optimization of IRS-assisted MU-MIMO Communication Systems through a DRL-based Twin Delayed DDPG Approach
2022 IEEE International Symposium on Broadband Multimedia Systems and Broadcasting (BMSB)(2022)
摘要
Intelligent reflecting surface (IRS)-assisted multiple-input multiple-output (MIMO) systems are foreseen as key enablers of beyond 5G (B5G) and 6G wireless communications. By properly designing the MIMO precoding matrices and the IRS phase-shift matrix, the system performance significantly improves in terms of higher transmission rates, lower power consumption and delays, and improved communication security. To overcome the high dimensionality of the joint optimization of the precoders and the IRS phase shift matrix, we propose an innovative deep reinforcement learning (DRL)-based approach. We aim at maximizing the system sum-rate by considering an adaptation of the deep deterministic policy gradient (DDPG) framework, namely twin delayed DDPG (TD3). Hence, the optimization problem is formulated in terms of continuous action and state spaces, while artificial neural networks (ANNs) are used for the function approximations. The simulation results show that the proposed solution reaches a competitive performance when compared with other state-of-the-art algorithms.
更多查看译文
关键词
deep reinforcement learning,TD3,MU-MIMO,intelligent reflecting surfaces
AI 理解论文
溯源树
样例
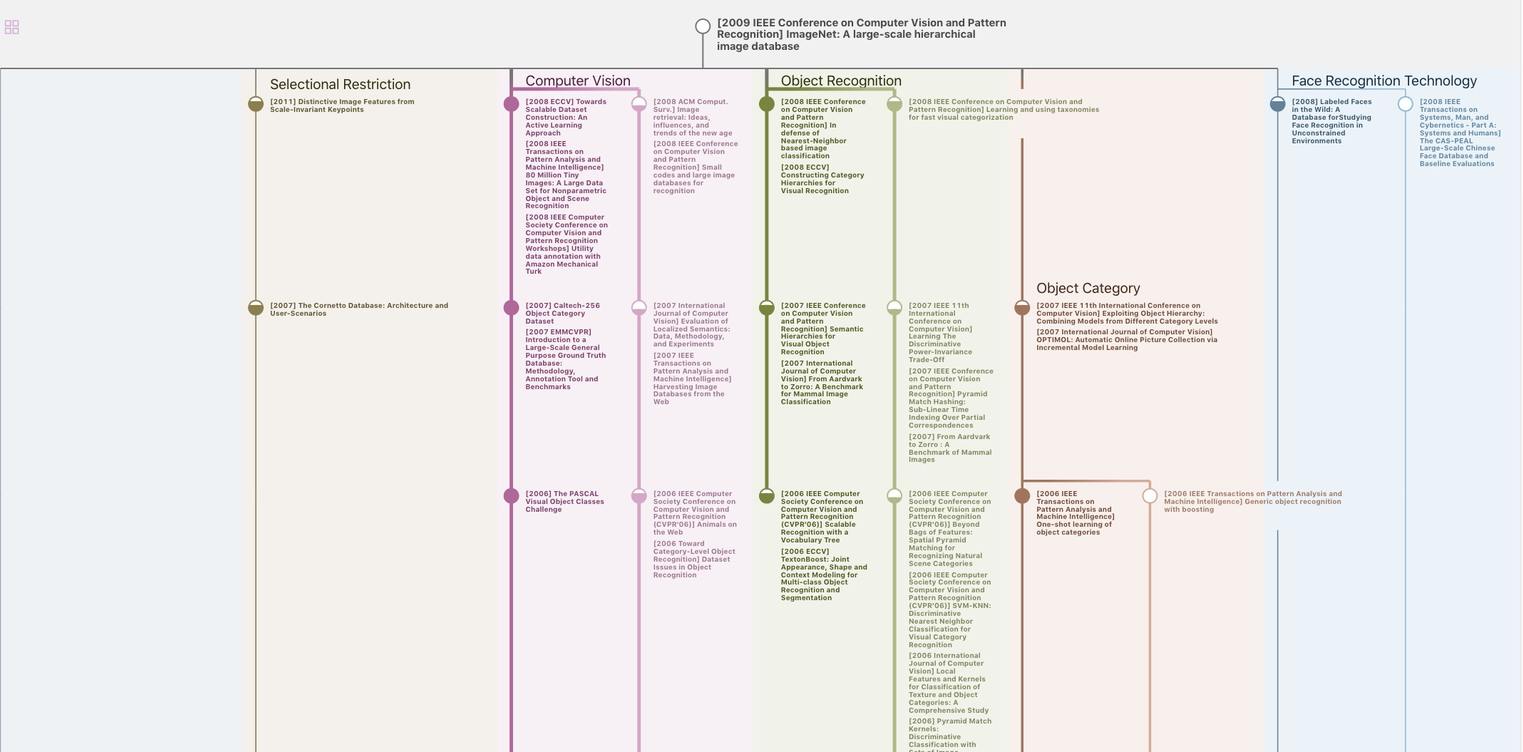
生成溯源树,研究论文发展脉络
Chat Paper
正在生成论文摘要