A STL-GALSTM Model to Predict the Track Irregularity of High-Speed Railway
2021 IEEE 15th International Conference on Big Data Science and Engineering (BigDataSE)(2021)
摘要
The accurate prediction of track irregularity has great practical significance for high-speed railway maintenance, train safety, and comfortable operation. In this paper, we propose a STL-GALSTM model to predict the track irregularity for high-speed railway. First, the seasonal-trend decomposition using loess (STL) method is utilized to decompose the track irregularity time series into the trend, seasonal, and remainder components. Then the Long short-term memory (LSTM) model is used to predict the decomposed components. And then the genetic algorithm (GA) optimizes the structure of LSTM. The experiment results show the STL-GALSTM can obtain an accurate track irregularity prediction value.
更多查看译文
关键词
track irregularity prediction,genetic algorithm,long short-term memory network,time series decomposition,high-speed railway
AI 理解论文
溯源树
样例
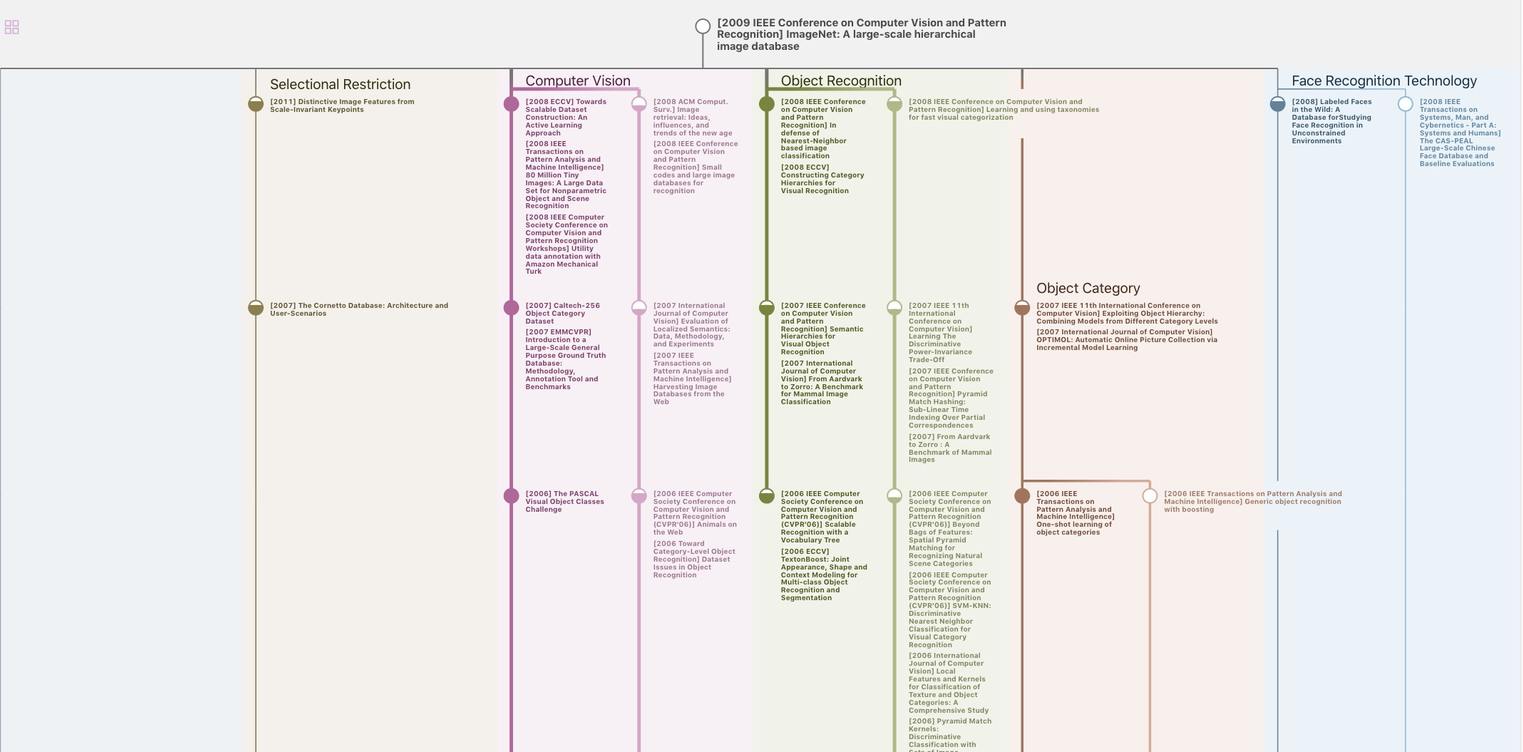
生成溯源树,研究论文发展脉络
Chat Paper
正在生成论文摘要