A big data approach for Fuel Oil Consumption estimation in the maritime industry
2022 IEEE Eighth International Conference on Big Data Computing Service and Applications (BigDataService)(2022)
摘要
Route optimization has been a research topic for many years in the maritime industry and it constitutes one of the key components to improving energy efficiency and sustainability in ship operations. This paper deals with the challenge of estimating Fuel Oil Consumption (FOC) in the context of Weather Routing (WR). Given a plethora of features collected from the vessel’s Automatic Identification System (AIS) or on-board sensor installations, we examine how a predictive FOC scheme can be coupled with WR optimization algorithms in order to reduce the vessel’s FOC, emissions, and the overall cost of a voyage. In order to handle the amount of data required for FOC prediction, we employ a streaming pipeline that harvests data in real-time from different sources and processes them appropriately for visualization, causal analysis, and forecasting purposes. In this direction, we first conduct an exploratory analysis to examine and unveil the importance and inter-association between the various variables related to sea-keeping and weather features, in order to utilize them effectively in the context of a FOC predictive scheme. Furthermore, we introduce a novel recurrent neural network architecture that approximates ideally the underlying function describing the features and the vessel’s FOC by taking into account historical data, and we showcase the results. Finally, we demonstrate how the FOC prediction model can be coupled with a WR algorithm to propose the optimal route for a vessel in terms of FOC efficiency.
更多查看译文
关键词
big data collection,information processing,vessel data,fuel oil consumption,weather routing optimization,recurrent neural networks
AI 理解论文
溯源树
样例
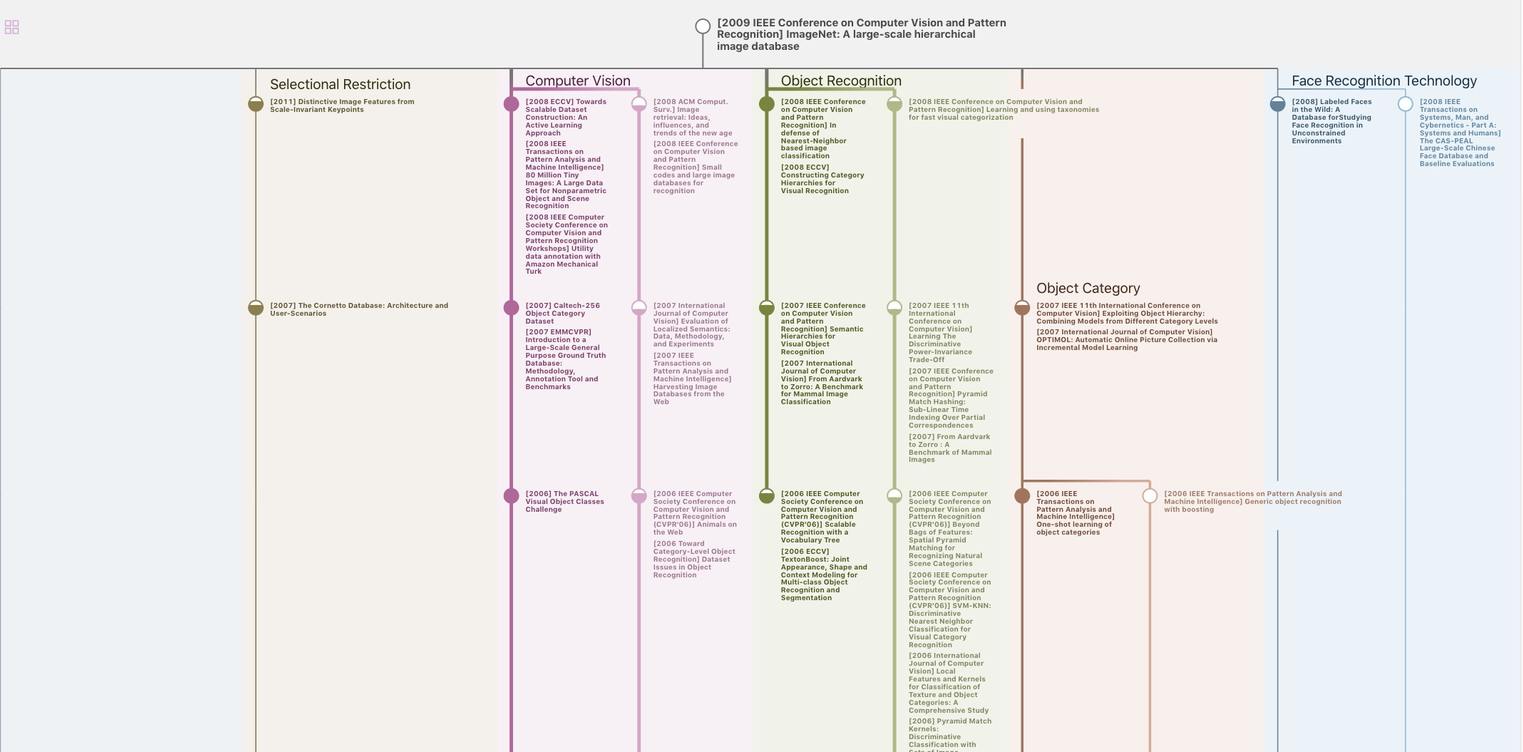
生成溯源树,研究论文发展脉络
Chat Paper
正在生成论文摘要