Transformers for Urban Sound Classification - A Comprehensive Performance Evaluation.
Sensors(2022)
摘要
Many relevant sound events occur in urban scenarios, and robust classification models are required to identify abnormal and relevant events correctly. These models need to identify such events within valuable time, being effective and prompt. It is also essential to determine for how much time these events prevail. This article presents an extensive analysis developed to identify the best-performing model to successfully classify a broad set of sound events occurring in urban scenarios. Analysis and modelling of Transformer models were performed using available public datasets with different sets of sound classes. The Transformer models' performance was compared to the one achieved by the baseline model and end-to-end convolutional models. Furthermore, the benefits of using pre-training from image and sound domains and data augmentation techniques were identified. Additionally, complementary methods that have been used to improve the models' performance and good practices to obtain robust sound classification models were investigated. After an extensive evaluation, it was found that the most promising results were obtained by employing a Transformer model using a novel Adam optimizer with weight decay and transfer learning from the audio domain by reusing the weights from AudioSet, which led to an accuracy score of 89.8% for the UrbanSound8K dataset, 95.8% for the ESC-50 dataset, and 99% for the ESC-10 dataset, respectively.
更多查看译文
关键词
Adam optimizer,convolutional neural network,data augmentation,deep learning,urban sounds’ classification
AI 理解论文
溯源树
样例
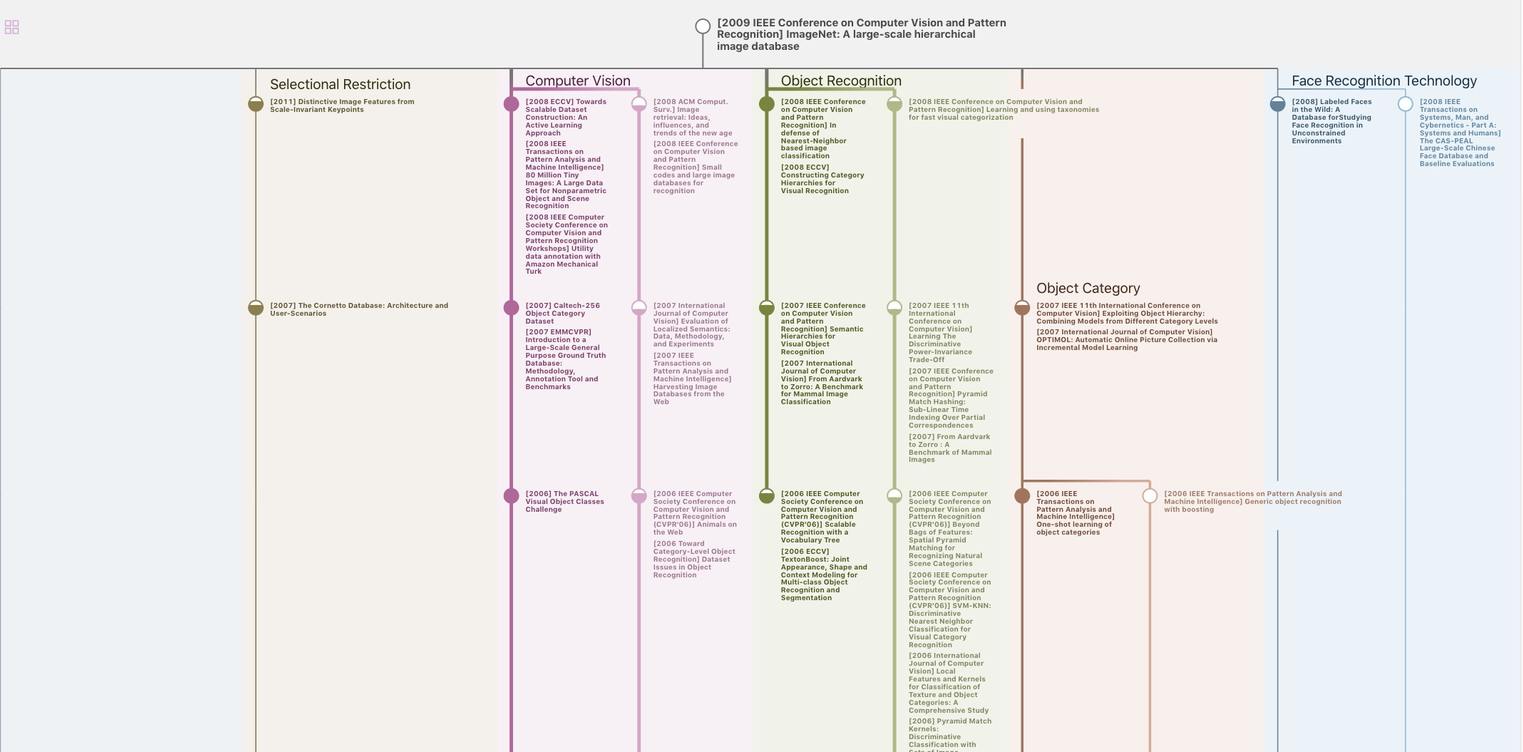
生成溯源树,研究论文发展脉络
Chat Paper
正在生成论文摘要