Combating False Data Injection Attacks on Human-Centric Sensing Applications.
Proceedings of the ACM on Interactive, Mobile, Wearable and Ubiquitous Technologies(2022)
摘要
The recent prevalence of machine learning-based techniques and smart device embedded sensors has enabled widespread human-centric sensing applications. However, these applications are vulnerable to false data injection attacks (FDIA) that alter a portion of the victim's sensory signal with forged data comprising a targeted trait. Such a mixture of forged and valid signals successfully deceives the continuous authentication system (CAS) to accept it as an authentic signal. Simultaneously, introducing a targeted trait in the signal misleads human-centric applications to generate specific targeted inference; that may cause adverse outcomes. This paper evaluates the FDIA's deception efficacy on sensor-based authentication and human-centric sensing applications simultaneously using two modalities - accelerometer, blood volume pulse signals. We identify variations of the FDIA such as different forged signal ratios, smoothed and non-smoothed attack samples. Notably, we present a novel attack detection framework named Siamese-MIL that leverages the Siamese neural networks' generalizable discriminative capability and multiple instance learning paradigms through a unique sensor data representation. Our exhaustive evaluation demonstrates Siamese-MIL's real-time execution capability and high efficacy in different attack variations, sensors, and applications.
更多查看译文
关键词
Injection Attack, False Data Injection Attack, Multiple Instance Learning, Siamese Network, Mobile, Wearable, Authentication, Sensor Attack, Defense, Deep Learning
AI 理解论文
溯源树
样例
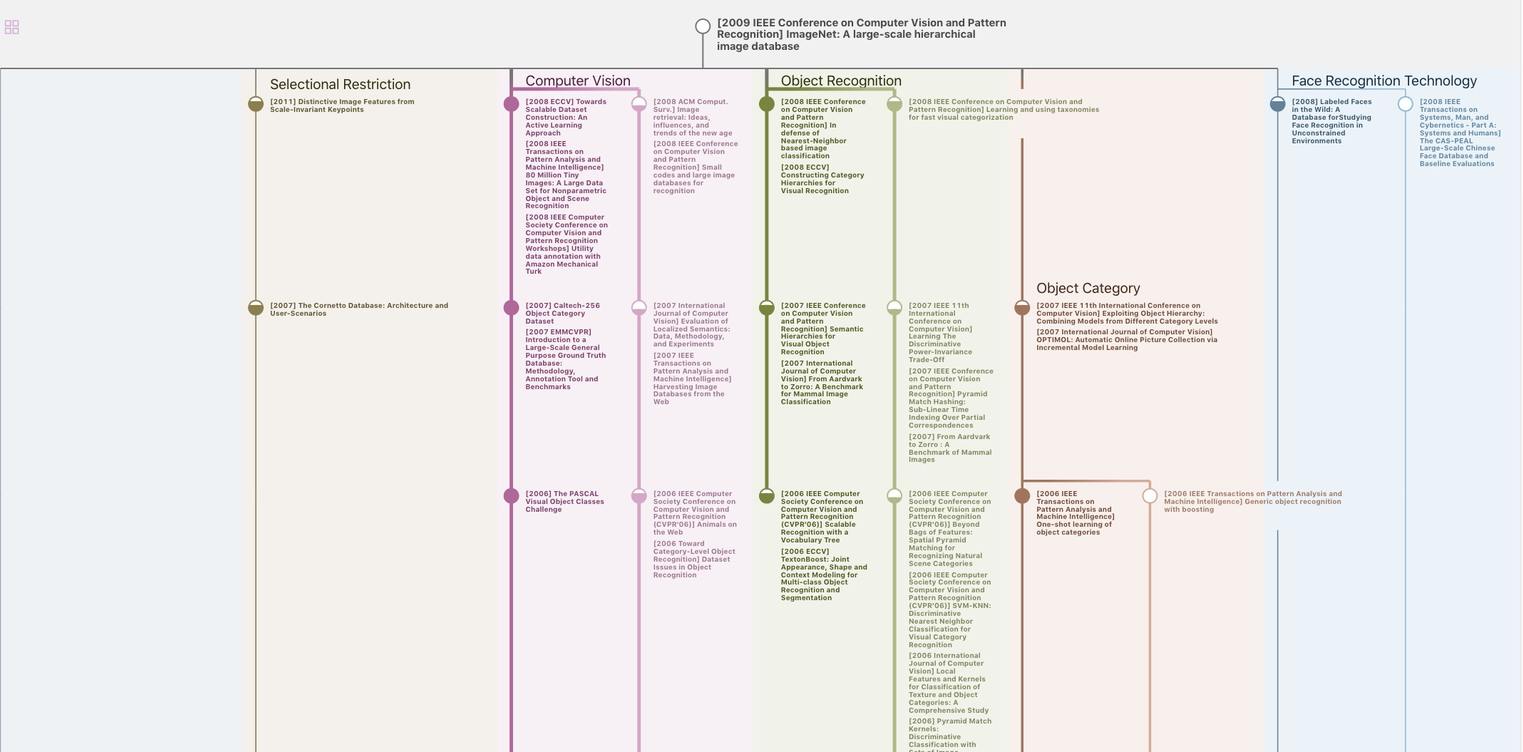
生成溯源树,研究论文发展脉络
Chat Paper
正在生成论文摘要