Training Quantum Boltzmann Machines with Coresets
2022 IEEE International Conference on Quantum Computing and Engineering (QCE)(2022)
摘要
Recent work has proposed and explored using coreset techniques for quantum algorithms that operate on classical data sets to accelerate the applicability of these algorithms on near-term quantum devices. We apply these ideas to Quantum Boltzmann Machines (QBM) where gradient-based steps which require Gibbs state sampling are the main computational bottle-neck during training. By using a coreset in place of the full data set, we try to minimize the number of steps needed and accelerate the overall training time. In a regime where computational time on quantum computers is a precious resource, we propose this might lead to substantial practical savings. We evaluate this approach on 6x6 binary images from an augmented bars and stripes data set using a QBM with 36 visible units and 8 hidden units. Using an Inception score inspired metric, we compare QBM training times with and without using coresets.
更多查看译文
关键词
Coreset,Quantum Boltzmann Machine,Gibbs,NISQ,Machine Learning
AI 理解论文
溯源树
样例
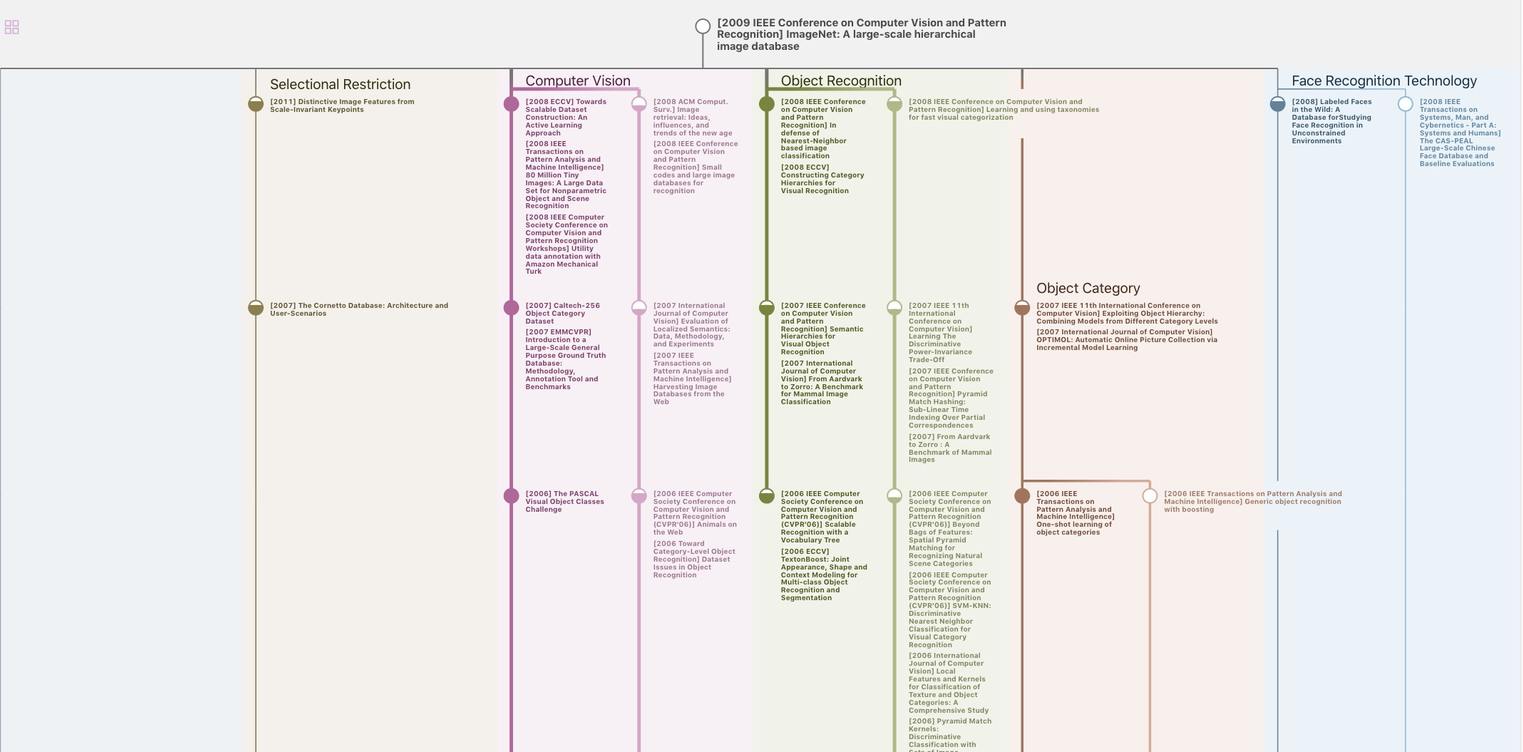
生成溯源树,研究论文发展脉络
Chat Paper
正在生成论文摘要