Local polynomial regression for spatial data on $\mathbb{R}^d$
arxiv(2022)
摘要
This paper develops a general asymptotic theory of local polynomial (LP) regression for spatial data observed at irregularly spaced locations in a sampling region $R_n \subset \mathbb{R}^d$. We adopt a stochastic sampling design that can generate irregularly spaced sampling sites in a flexible manner including both pure increasing and mixed increasing domain frameworks. We first introduce a nonparametric regression model for spatial data defined on $\mathbb{R}^d$ and then establish the asymptotic normality of LP estimators with general order $p \geq 1$. We also propose methods for constructing confidence intervals and establish uniform convergence rates of LP estimators. Our dependence structure conditions on the underlying processes cover a wide class of random fields such as L\'evy-driven continuous autoregressive moving average random fields. As an application of our main results, we discuss a two-sample testing problem for mean functions and their partial derivatives.
更多查看译文
AI 理解论文
溯源树
样例
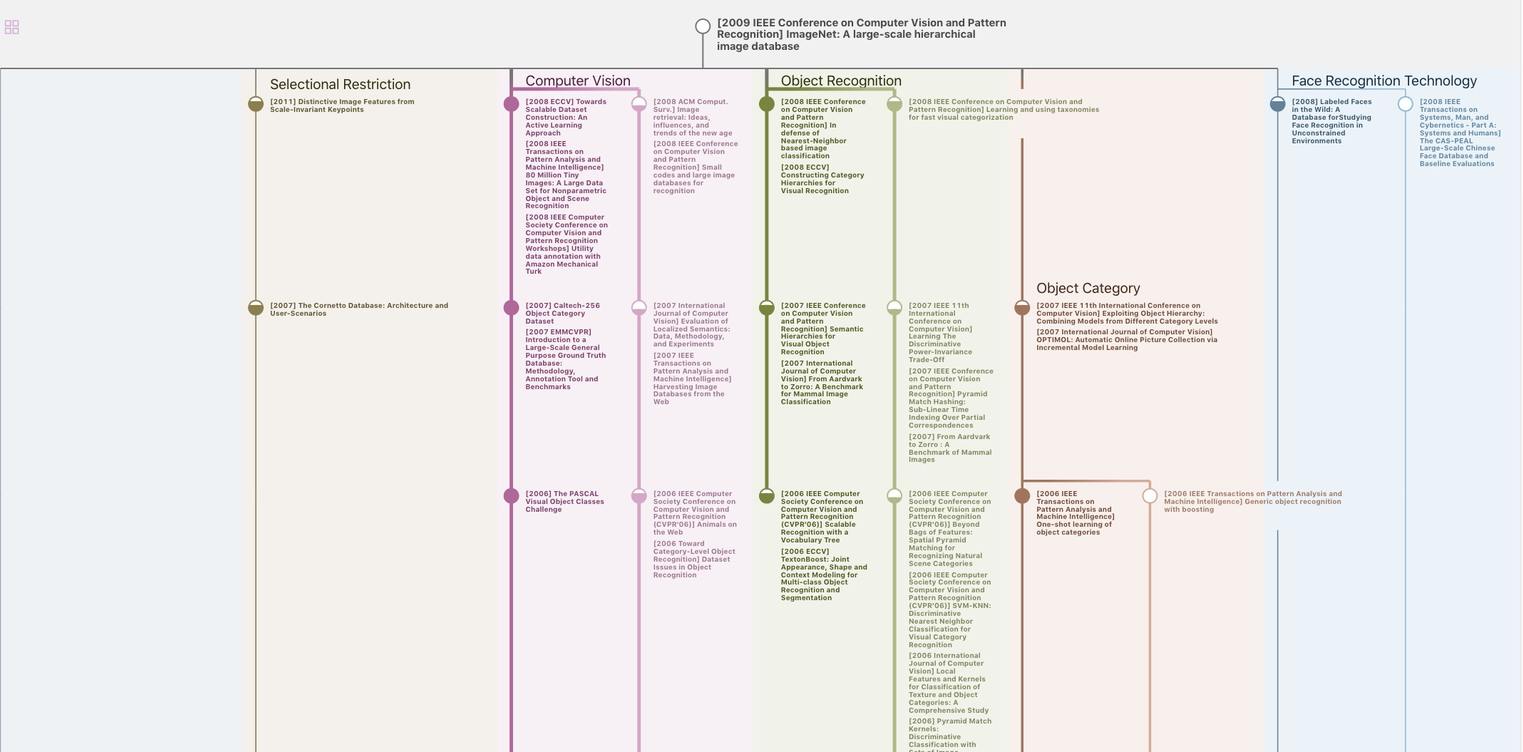
生成溯源树,研究论文发展脉络
Chat Paper
正在生成论文摘要