JetFlow: Generating Jets with Conditioned and Mass Constrained Normalising Flows
arXiv (Cornell University)(2022)
摘要
Fast data generation based on Machine Learning has become a major research topic in particle physics. This is mainly because the Monte Carlo simulation approach is computationally challenging for future colliders, which will have a significantly higher luminosity. The generation of collider data is similar to point cloud generation with complex correlations between the points. In this study, the generation of jets with up to 30 constituents with Normalising Flows using Rational Quadratic Spline coupling layers is investigated. Without conditioning on the jet mass, our Normalising Flows are unable to model all correlations in data correctly, which is evident when comparing the invariant jet mass distributions between ground truth and generated data. Using the invariant mass as a condition for the coupling transformation enhances the performance on all tracked metrics. In addition, we demonstrate how to sample the original mass distribution by interpolating the empirical cumulative distribution function. Similarly, the variable number of constituents is taken care of by introducing an additional condition on the number of constituents in the jet. Furthermore, we study the usefulness of including an additional mass constraint in the loss term. On the dataset, our model shows state-of-the-art performance combined with fast and stable training.
更多查看译文
关键词
Representation Learning,Statistical Modeling
AI 理解论文
溯源树
样例
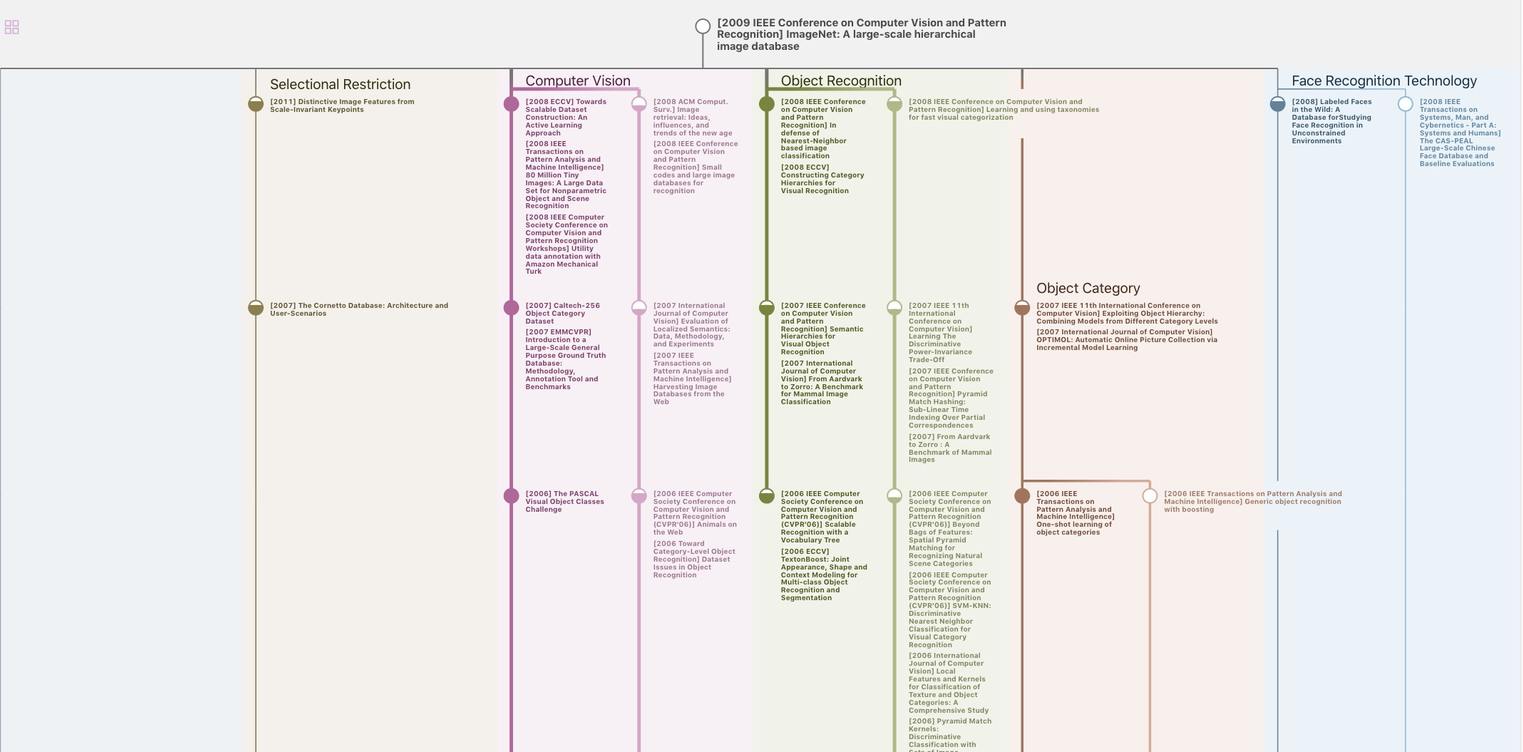
生成溯源树,研究论文发展脉络
Chat Paper
正在生成论文摘要